Safe DNN-type Controller Synthesis for Nonlinear Systems via Meta Reinforcement Learning.
DAC(2023)
摘要
There is a pressing need to synthesize provable safety controllers for nonlinear systems as they are embedded in many safety-critical applications. In this paper, we propose a safe Meta Reinforcement Learning (Meta-RL) approach to synthesize deep neural network (DNN) controllers for nonlinear systems subject to safety constraints. Our approach incorporates two phases: Meta-RL for training the controller network, and formal safety verification based on polynomial optimization solving. In the training phase, we provide a training framework which pretrains a unified meta-initial controller for control systems by meta-learning. An important benefit of the proposed Meta-RL approach lies in that it is much more effective and succeeds in more controller training tasks compared with existing typical RL methods, e.g., Deep Deterministic Policy Gradient (DDPG). To formally verify the safety properties of the closed-loop system with the learned controller, we develop a verification procedure by using polynomial inclusion computation in combination with barrier certificate generation. Experiments on a set of benchmarks, including systems with dimension up to 12, demonstrate the effectiveness and applicability of our method.
更多查看译文
关键词
formal verification,controller synthesis,reinforcement learning,meta learning
AI 理解论文
溯源树
样例
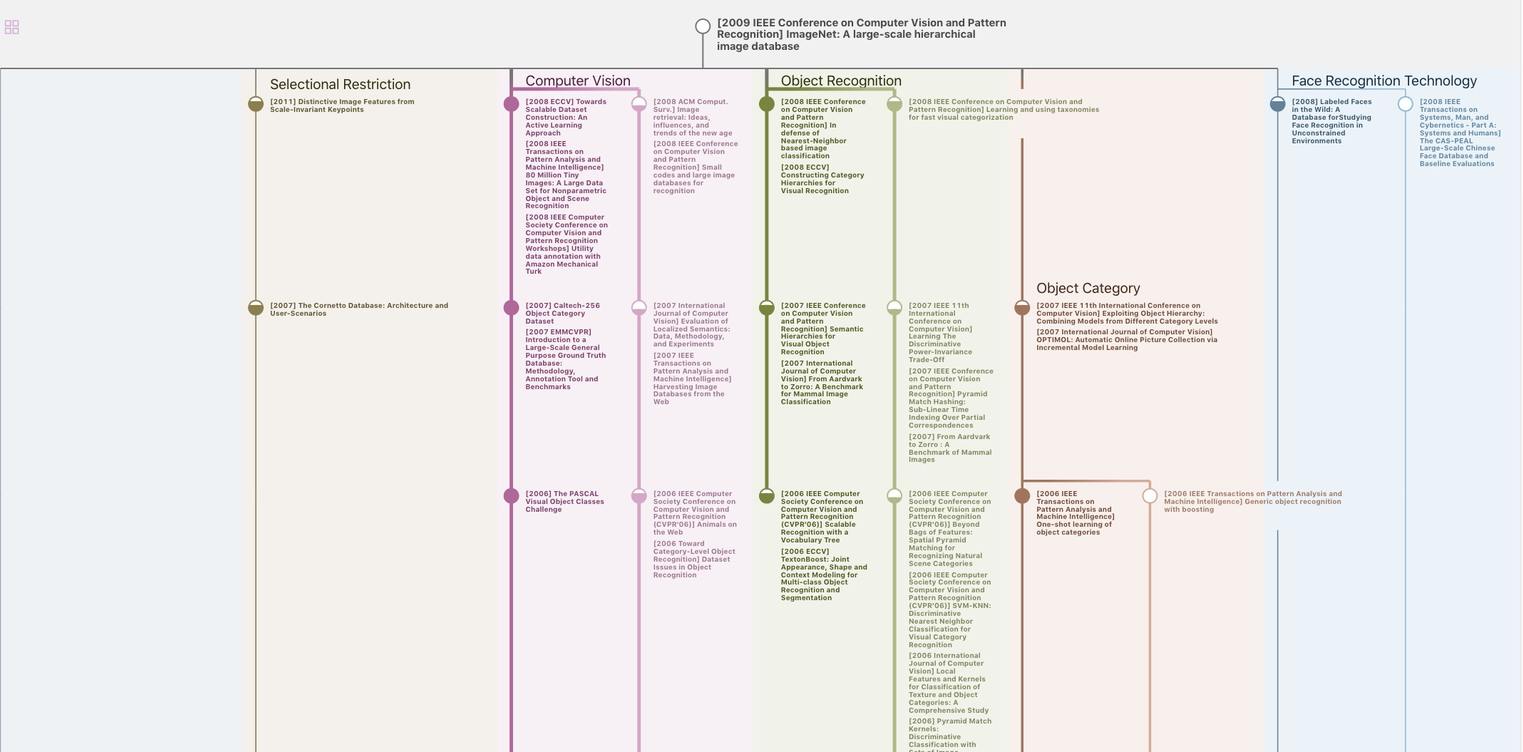
生成溯源树,研究论文发展脉络
Chat Paper
正在生成论文摘要