Multi-party Computation for Privacy and Security in Machine Learning: a practical review
CSR(2023)
摘要
Machine Learning, particularly Deep Learning, is transforming society in any of its fundamental domains - healthcare, culture, finance, transportation, education, just to mention a few. However Machine Learning suffers from serious weaknesses in privacy and security due to the large amount of data (datasets for training and parameters in trained models) and the probabilistic approximation inherent in any ML function. Multi-Party Computation (MPC) is a family of techniques and tactic with a sound scientific and operative base that can be applied to mitigate some relevant weaknesses of ML. In particular, privacy in training may be assured by MPC with federated learning techniques (these may be considered particular interpretations and implementation of a general MPC method) and also security in training and inference may be enforced by continuous model testing using MPC is a technique that allows multiple parties to evaluate a machine learning model on their private data without revealing it to each other. This brief paper is a practical and essential review on how to use MPC to mitigate privacy and security issues in ML.
更多查看译文
关键词
deep learning,federated learning techniques,general MPC method,healthcare,machine learning,machine learning model,ML function,multiparty computation,multiple parties,operative base,probabilistic approximation
AI 理解论文
溯源树
样例
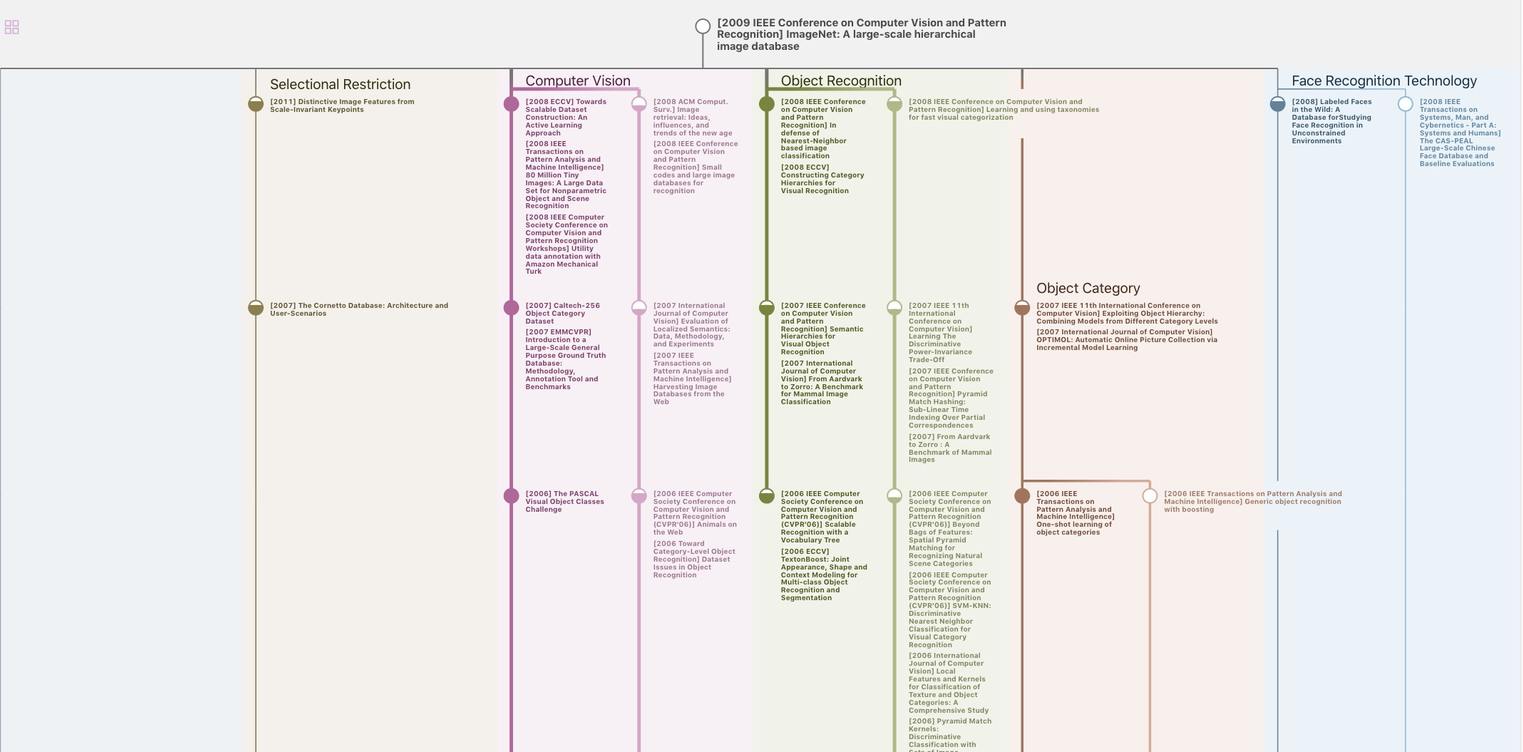
生成溯源树,研究论文发展脉络
Chat Paper
正在生成论文摘要