Downsampling GAN for Small Object Data Augmentation
COMPUTER ANALYSIS OF IMAGES AND PATTERNS, CAIP 2023, PT I(2023)
摘要
The limited visual information provided by small objects-under 32x32 pixels-makes small object detection a particularly challenging problem for current detectors. Moreover, standard datasets are biased towards large objects, limiting the variability of the training set for the small objects subset. Although new datasets specifically designed for small object detection have been recently released, the detection precision is still significantly lower than that of standard object detection. We propose a data augmentation method based on a Generative Adversarial Network (GAN) to increase the availability of small object samples at training time, boosting the performance of standard object detectors in this highly demanding subset. Our Downsampling GAN (DS-GAN) generates new small objects from larger ones, avoiding the unrealistic artifacts created by traditional resizing methods. The synthetically generated objects are inserted in the original dataset images in plausible positions without causing mismatches between foreground and background. The proposed method improves the AP(s)(@[.5,.95]) and AP(s)(@.5) of a standard object detector in the UVDT small subset by more than 4 and 10 points, respectively.
更多查看译文
关键词
object detection,GAN,data augmentation
AI 理解论文
溯源树
样例
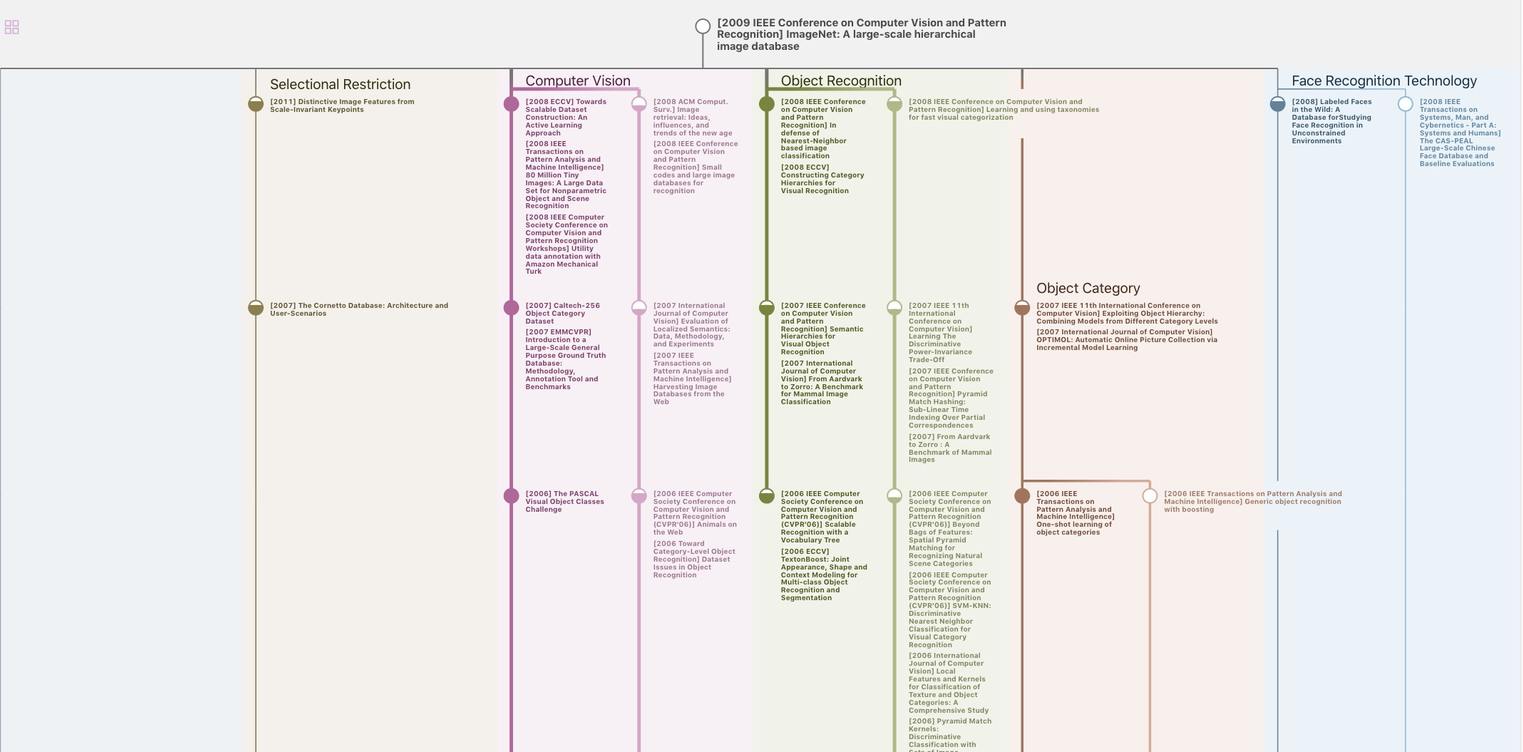
生成溯源树,研究论文发展脉络
Chat Paper
正在生成论文摘要