Choosing the Task Allocator: Effect on Performance and Satisfaction in Human-Agent Team.
FLAIRS(2023)
Abstract
Ad hoc human-agent teams, where team members interact without prior experience with teammates and only for a limited number of interactions, will be commonplace in dynamic environments with opportunity windows for collaboration between diverse groups. We study the efficacy of virtual ad-hoc teams, consisting of a human and an agent, collaborating to complete tasks in each of a few episodes. To maximize team potential, the relative expertise of team members must be measured and utilized in allocating tasks. As team members are not initially aware of each other's task competence and as humans often cannot accurately estimate their competencies, adapting allocation over the episodes is critical to team performance. Human team member satisfaction with allocations is also critical to determining team viability. We therefore use both these criteria to measure the effectiveness of task allocation procedures with varying degree of flexibility and human teammate control: (a) alternating, (b) performance adaptive, (c) agent-guided, (d) human-selected. We report on the relative strengths of these allocation procedures based on results from experiments with MTurk workers.
MoreTranslated text
Key words
Team Effectiveness,Virtual Teams,Leadership in Teams,Team Processes,Agent-Based Modeling
PDF
View via Publisher
AI Read Science
AI Summary
AI Summary is the key point extracted automatically understanding the full text of the paper, including the background, methods, results, conclusions, icons and other key content, so that you can get the outline of the paper at a glance.
Example
Background
Key content
Introduction
Methods
Results
Related work
Fund
Key content
- Pretraining has recently greatly promoted the development of natural language processing (NLP)
- We show that M6 outperforms the baselines in multimodal downstream tasks, and the large M6 with 10 parameters can reach a better performance
- We propose a method called M6 that is able to process information of multiple modalities and perform both single-modal and cross-modal understanding and generation
- The model is scaled to large model with 10 billion parameters with sophisticated deployment, and the 10 -parameter M6-large is the largest pretrained model in Chinese
- Experimental results show that our proposed M6 outperforms the baseline in a number of downstream tasks concerning both single modality and multiple modalities We will continue the pretraining of extremely large models by increasing data to explore the limit of its performance
Try using models to generate summary,it takes about 60s
Must-Reading Tree
Example
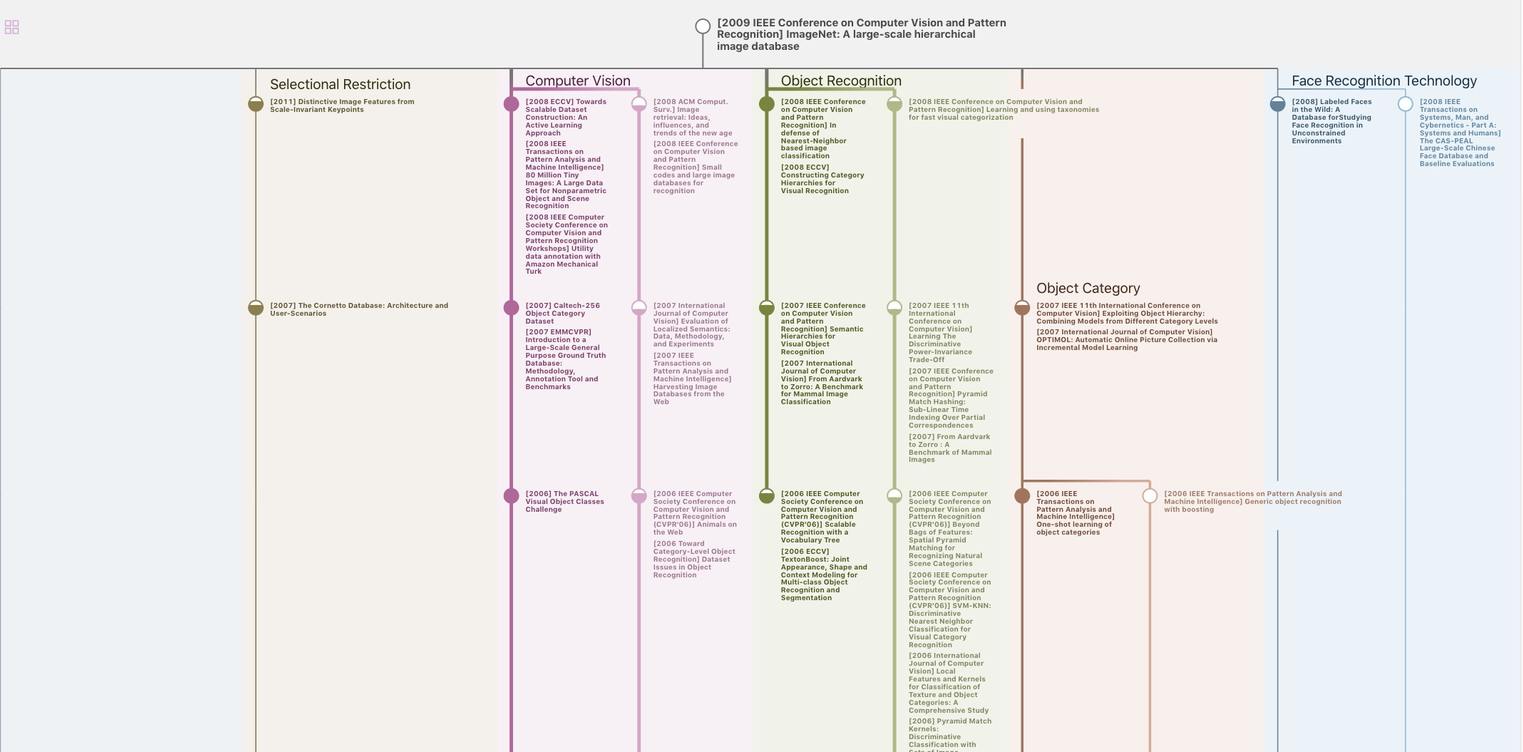
Generate MRT to find the research sequence of this paper
Data Disclaimer
The page data are from open Internet sources, cooperative publishers and automatic analysis results through AI technology. We do not make any commitments and guarantees for the validity, accuracy, correctness, reliability, completeness and timeliness of the page data. If you have any questions, please contact us by email: report@aminer.cn
Chat Paper
GPU is busy, summary generation fails
Rerequest