Microsaccade Segmentation using Directional Variance Analysis and Artificial Neural Networks.
IRI(2023)
摘要
Fixational eye movements (FEMs) are an essential component of vision and there is considerable research interest in using them as biomarkers of brain injury and neurodegeneration. Study of FEMs often involves segmenting them into their individual components, primarily microsaccades and drifts. In practice, velocity (or acceleration) thresholds are commonly adapted-while they are generally imperfect-requiring tuning of thresholds and manual correction and verification by human graders. Manual segmentation and correction is a tedious and time-consuming process for human graders. Fortunately, it can be observed from Tracking scanning laser ophthalmoscopy (TSLO) video recordings that the directional variances of FEMs can be extracted to mathematically characterize microsaccades for segmentation and distinguish from drift. Therefore, we perform a directional variance analysis, extract relevant features, and automate the model using artificial neural networks (ANN). We propose and compare two directional variance approaches along with an ANN model for the segmentation of microsaccades. The first approach utilizes a single-point based feature variance, whereas the second approach utilizes a sliding-window based feature variance with the information from several time points. We calculate several statistical metrics to characterize the features of the microsaccades such as the number of microsaccades, microsaccade peak velocity and acceleration, and microsaccade duration. We have also calculated the accuracy, precision, sensitivity, and specificity scores for each approach to compare their performance. The single-point models labeled the FEM data with an accuracy of 70% whereas the sliding-window approach had an accuracy of 85%. When comparing the percent errors of the approaches to the ground truth, the sliding-window approach performs significantly better than the single-point approach as it captures more relevant directional variance features of FEMs.
更多查看译文
关键词
Fixational eye movement, microsaccade, artificial neural networks, directional variance, feature space
AI 理解论文
溯源树
样例
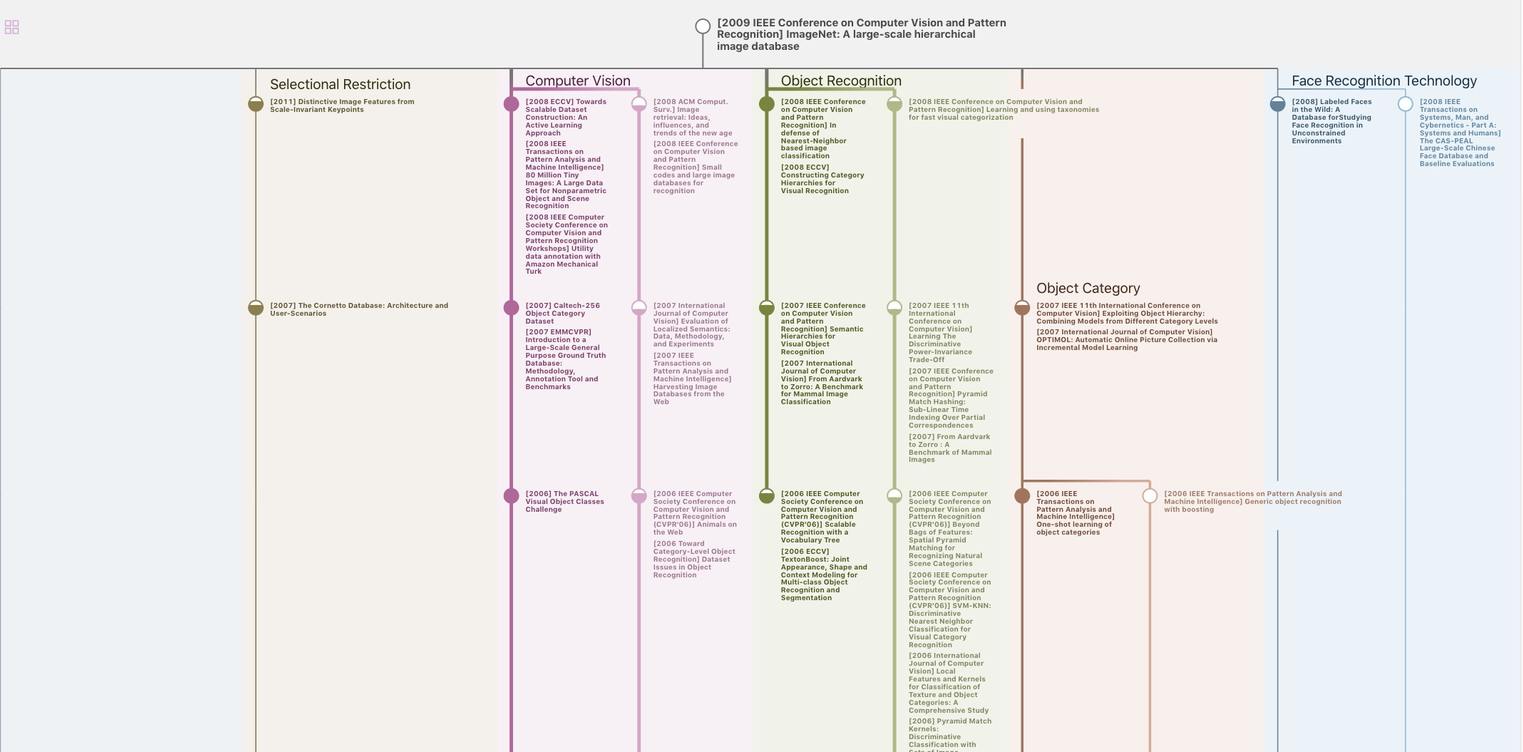
生成溯源树,研究论文发展脉络
Chat Paper
正在生成论文摘要