Phishing URL Detection with Prototypical Neural Network Disentangled by Triplet Sampling.
CISIS-ICEUTE(2023)
摘要
Phishing attacks continue to pose a significant threat to internet security, with phishing URLs being among the most prevalent attacks. Detecting these URLs is challenging, as attackers constantly evolve their tactics. Few-shot learning has emerged as a promising approach for learning from limited data, making it ideal for the task of phishing URL detection. In this paper, we propose a prototypical network (DPN) disentangled by triplet sampling that learns disentangled URL prototypes to improve the accuracy of phishing detection with limited data. The key idea is to capture the underlying structure and characteristics of URLs, making it highly effective in detecting phishing URLs. This method involves sampling triplets of anchor, positive, and negative URLs to train the network, which encourages the embedding space to be more separable between phishing and benign URLs. To evaluate the proposed method, we have collected and assessed a real-world dataset consisting of one million URLs, and additionally utilized two benchmark URL datasets. Our method outperforms the state-of-the-art models, achieving accuracies of 98.0% in a 2-way 50-shot task and 98.32% in a 2-way 5000-shot task. Moreover, the experiments highlight the advantages of using a disentangled representation of URLs, where t-SNE visualizations reveal distinct and well-separated URL prototypes.
更多查看译文
关键词
url detection,prototypical neural network,neural network
AI 理解论文
溯源树
样例
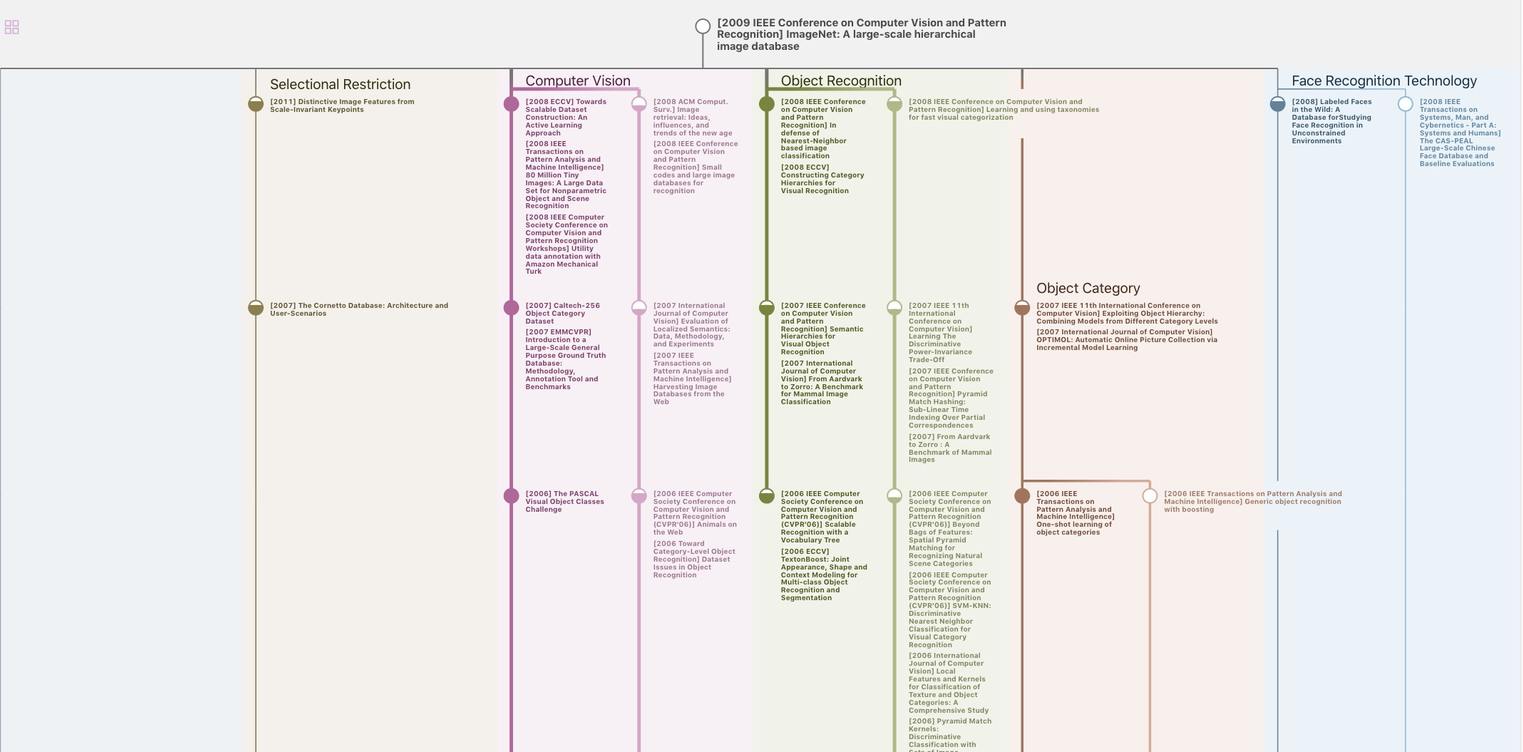
生成溯源树,研究论文发展脉络
Chat Paper
正在生成论文摘要