Learning Bezier-Durrmeyer Type Descriptors for Classifying Curves - Preliminary Studies.
ICAISC (1)(2023)
摘要
We aim to propose a new approach for generating descriptors for functional data that are represented by curves that may not be functions but rather parametrically described curves in 2D, 3D, etc. The idea of generating these descriptors is based on the Bezier curves, but instead of using classical control points, we propose to generalize the Durrmeyer approach for parametrically defined vector curves. The Durrmeyer-type descriptors are then estimated from noisy samples of the underlying curve and serve as input vectors to a selected classifier that is learned to recognize from which class of curves noisy observations come. The Bezier curves are not rapidly convergent. However, our aim is not to reconstruct functions but to recognize them, maintaining the shape-preserving and variation-diminishing properties of these curves that increase the classification accuracy in the presence of noise without invoking pre-filtering procedures. For more complicated curves, one can directly apply our approach to their parts in a similar way as polynomial splines are used. The proposed algorithm for learning descriptors of the Bezier-Durrmeyer type and training classifiers on them was tested on synthetic, but interesting per se, data from two families of the Lissajous curves observed with high amplitude noise.
更多查看译文
关键词
curves,bezier-durrmeyer
AI 理解论文
溯源树
样例
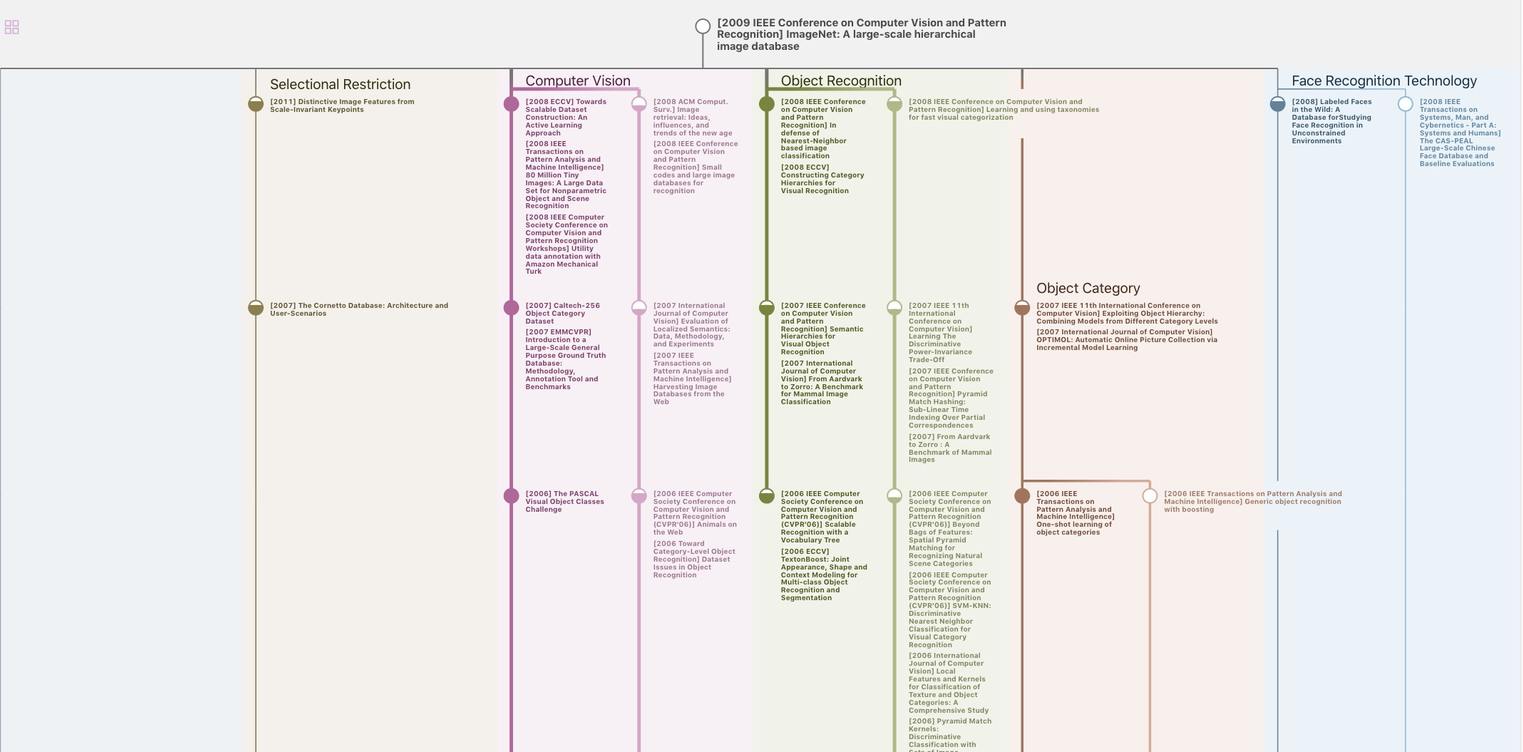
生成溯源树,研究论文发展脉络
Chat Paper
正在生成论文摘要