Boosting Generalized Few-Shot Learning by Scattering Intra-class Distribution
MACHINE LEARNING AND KNOWLEDGE DISCOVERY IN DATABASES: RESEARCH TRACK, ECML PKDD 2023, PT II(2023)
摘要
Generalized Few-Shot Learning (GFSL) applies the model trained with the base classes to predict the samples from both base classes and novel classes, where each novel class is only provided with a few labeled samples during testing. Limited by the severe data imbalance between base and novel classes, GFSL easily suffers from the prediction shift issue that most test samples tend to be classified into the base classes. Unlike the existing works that address this issue by either multi-stage training or complicated model design, we argue that extracting both discriminative and generalized feature representations is all GFSL needs, which could be achieved by simply scattering the intra-class distribution during training. Specifically, we introduce two self-supervised auxiliary tasks and a label permutation task to encourage the model to learn more image-level feature representations and push the decision boundary from novel towards base classes during inference. Our method is one-stage and could perform online inference. Experiments on the miniImageNet and tieredImageNet datasets show that the proposed method achieves comparable performance with the state-of-the-art multi-stage competitors under both traditional FSL and GFSL tasks, empirically proving that feature representation is the key for GFSL.
更多查看译文
关键词
Generalized Few-Shot Learning,Scatter Intra-class Distribution,Feature Representation
AI 理解论文
溯源树
样例
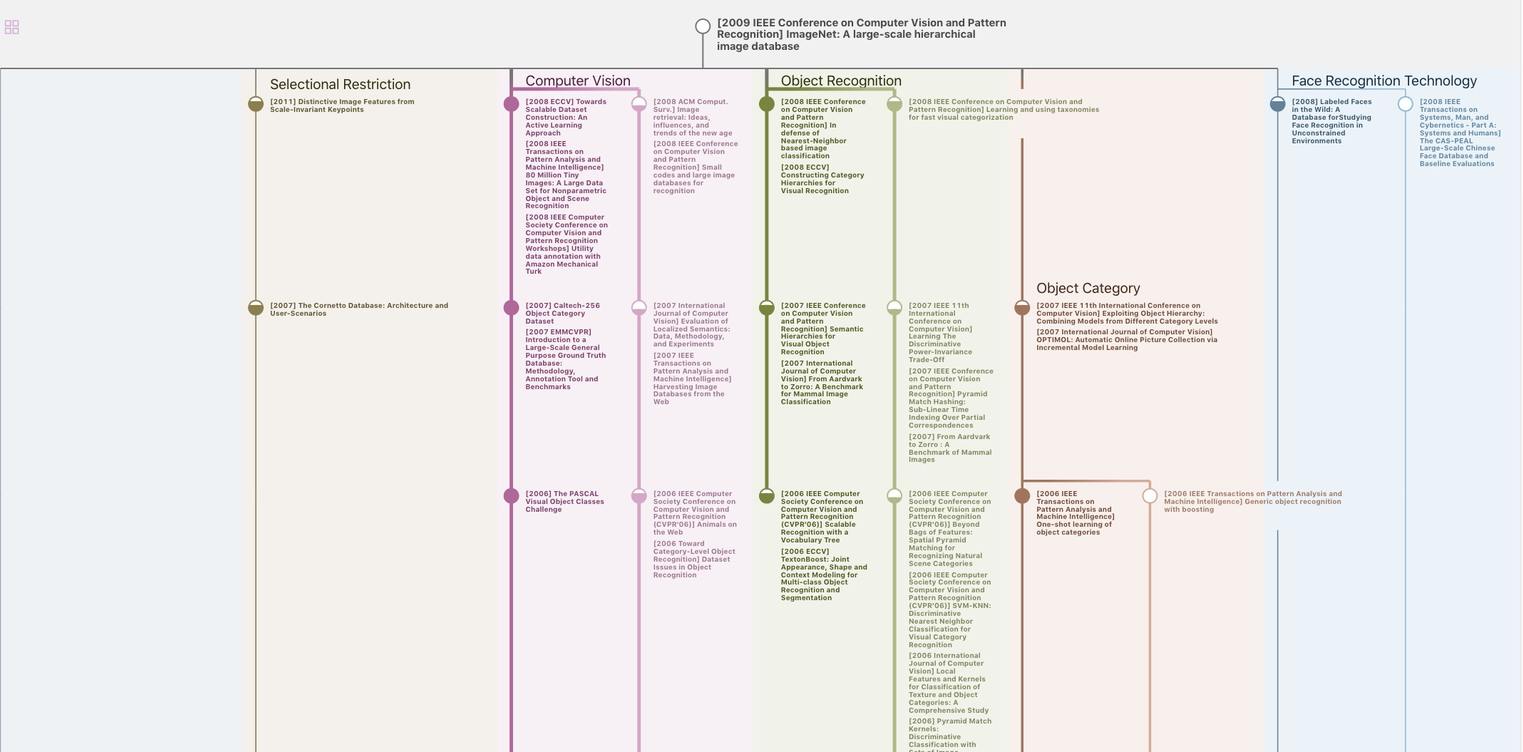
生成溯源树,研究论文发展脉络
Chat Paper
正在生成论文摘要