CVTGAD: Simplified Transformer with Cross-View Attention for Unsupervised Graph-Level Anomaly Detection
MACHINE LEARNING AND KNOWLEDGE DISCOVERY IN DATABASES: RESEARCH TRACK, ECML PKDD 2023, PT I(2023)
摘要
Unsupervised graph-level anomaly detection (UGAD) has received remarkable performance in various critical disciplines, such as chemistry analysis and bioinformatics. Existing UGAD paradigms often adopt data augmentation techniques to construct multiple views, and then employ different strategies to obtain representations from different views for jointly conducting UGAD. However, most previous works only considered the relationship between nodes/graphs from a limited receptive field, resulting in some key structure patterns and feature information being neglected. In addition, most existing methods consider different views separately in a parallel manner, which is not able to explore the inter-relationship across different views directly. Thus, a method with a larger receptive field that can explore the inter-relationship across different views directly is in need. In this paper, we propose a novel Simplified Transformer with Cross-View Attention for Unsupervised Graph-level Anomaly Detection, namely, CVTGAD. To increase the receptive field, we construct a simplified transformer-based module, exploiting the relationship between nodes/graphs from both intra-graph and inter-graph perspectives. Furthermore, we design a cross-view attention mechanism to directly exploit the view co-occurrence between different views, bridging the inter-view gap at node level and graph level. To the best of our knowledge, this is the first work to apply transformer and cross attention to UGAD, which realizes graph neural network and transformer working collaboratively. Extensive experiments on 15 real-world datasets of 3 fields demonstrate the superiority of CVTGAD on the UGAD task. The code is available at https://github.com/jindongli-Ai/CVTGAD.
更多查看译文
关键词
Transformer,Cross-View Attention,Graph-level Anomaly Detection,Unsupervised Learning,Graph Neural Network
AI 理解论文
溯源树
样例
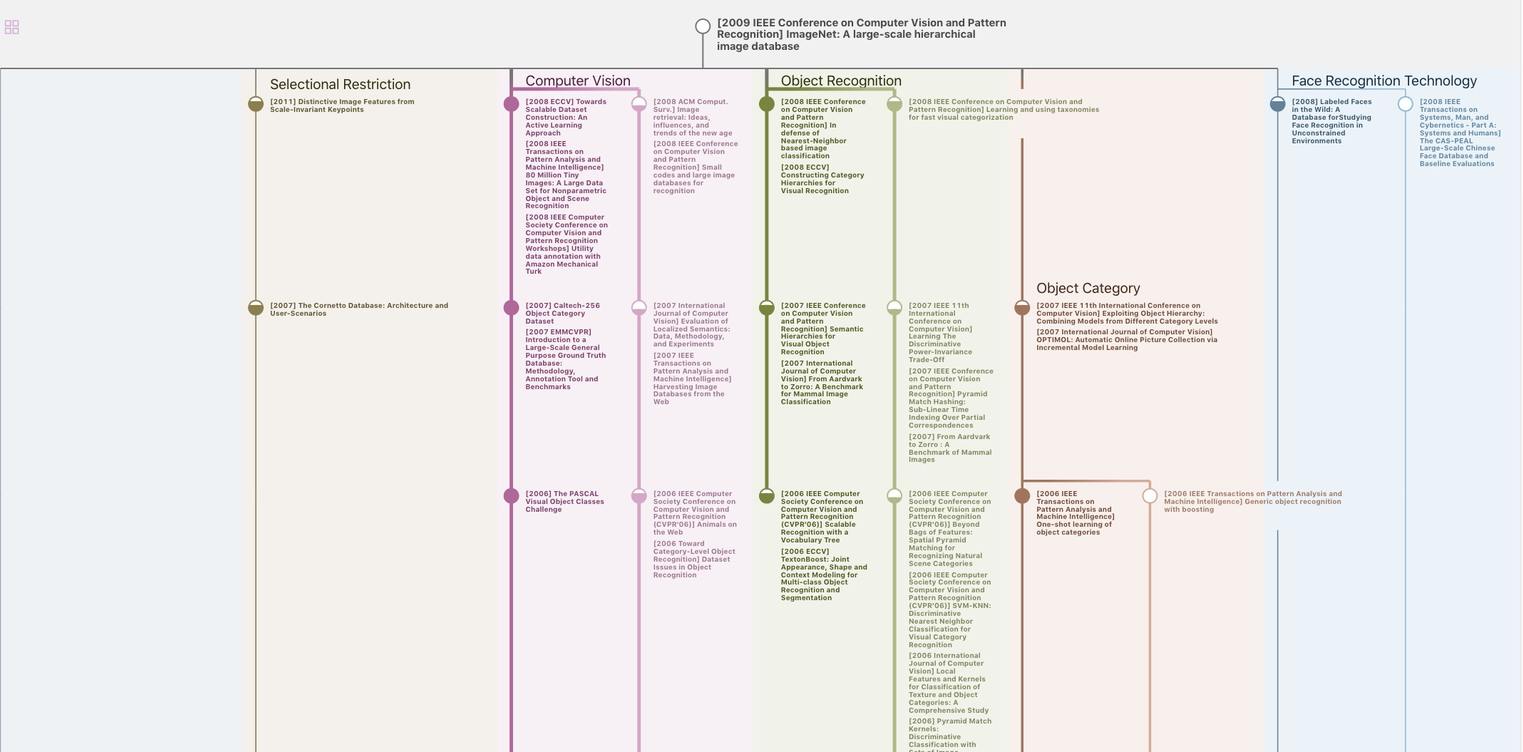
生成溯源树,研究论文发展脉络
Chat Paper
正在生成论文摘要