Comparing Apples and Oranges? On the Evaluation of Methods for Temporal Knowledge Graph Forecasting
MACHINE LEARNING AND KNOWLEDGE DISCOVERY IN DATABASES: RESEARCH TRACK, ECML PKDD 2023, PT III(2023)
摘要
Due to its ability to incorporate and leverage time information in relational data, Temporal Knowledge Graph (TKG) learning has become an increasingly studied research field. To predict the future based on TKG, researchers have presented innovative methods for Temporal Knowledge Graph Forecasting. However, the experimental procedures employed in this research area exhibit inconsistencies that significantly impact empirical results, leading to distorted comparisons among models. This paper focuses on the evaluation of TKG Forecasting models: We examine the evaluation settings commonly used in this research area and highlight the issues that arise. To make different approaches to TKG Forecasting more comparable, we propose a unified evaluation protocol and apply it to re-evaluate state-of-the-art models on the most commonly used datasets. Ultimately, we demonstrate the significant difference in results caused by different evaluation settings. We believe this work provides a solid foundation for future evaluations of TKG Forecasting models, thereby contributing to advancing this growing research area.
更多查看译文
关键词
Temporal Knowledge Graphs,Temporal Graphs,Temporal Knowledge Graph Forecasting
AI 理解论文
溯源树
样例
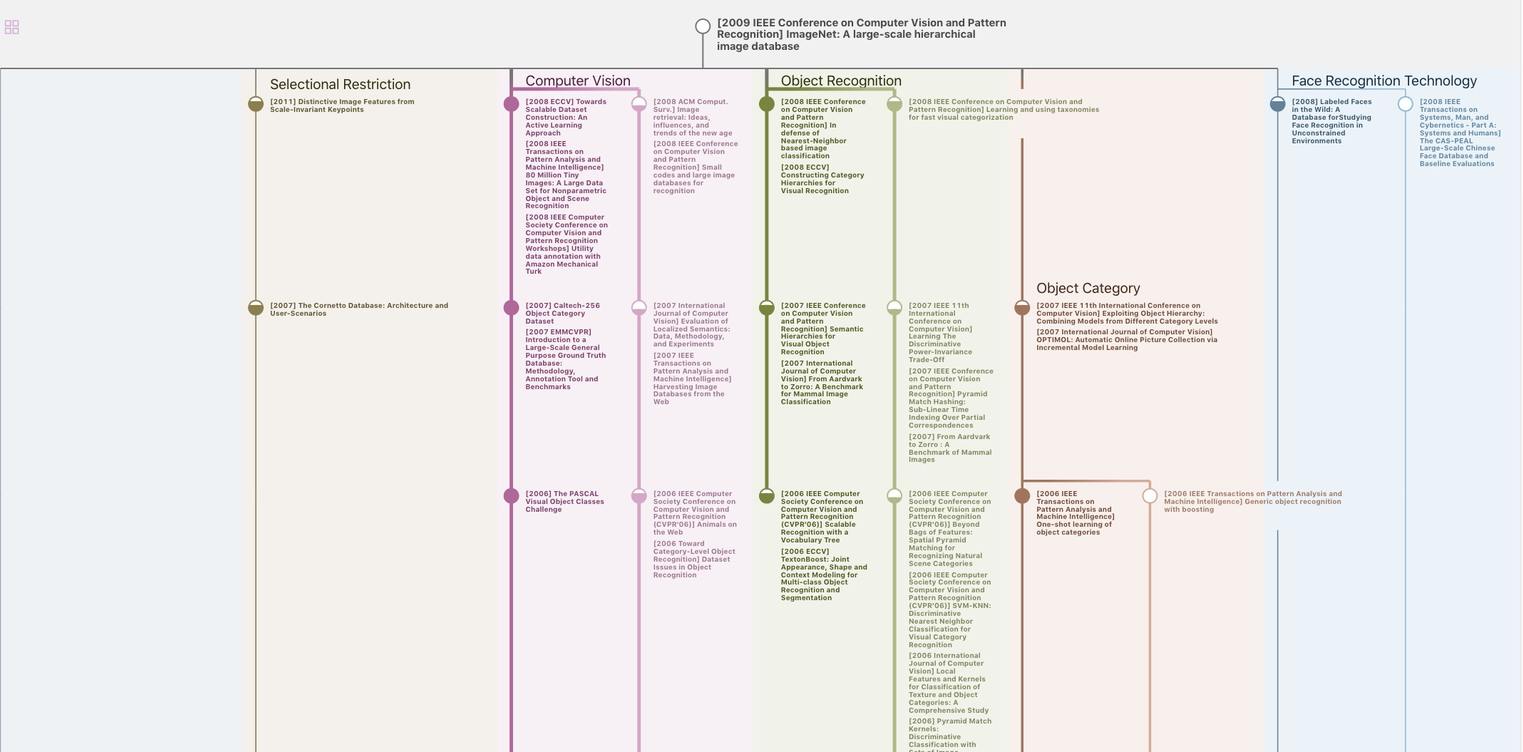
生成溯源树,研究论文发展脉络
Chat Paper
正在生成论文摘要