DE-Forest - Optimized Decision Tree Ensemble
COMPUTATIONAL COLLECTIVE INTELLIGENCE, ICCCI 2023(2023)
摘要
Classifier ensembles are still in the spotlight due to their proven applications for many practical problems. One of the most interesting approaches is methods proposing the construction of such models based on learned classifiers using randomly selected attributes. Among the essential algorithms in this area is Random Forest, which uses decision trees as base classifiers. Although these methods obtain outstanding quality for many tasks, the random selection of attributes for individual base classifiers used above does not guarantee the optimal ensemble selection. In this paper, we proposed DE-Forest - an ensemble classifier learning method using evolutionary techniques to select non-random and optimized subsets. Differential Evolution was chosen as the optimization algorithm, selecting the best set of attributes to learn the individual base classifiers. The quality of the proposed method has been evaluated based on computer experiments performed on many benchmark datasets. The obtained results are promising and show the superiority of the proposed method over the benchmark solutions.
更多查看译文
关键词
Random Forest,classifier ensemble,classification,optimization
AI 理解论文
溯源树
样例
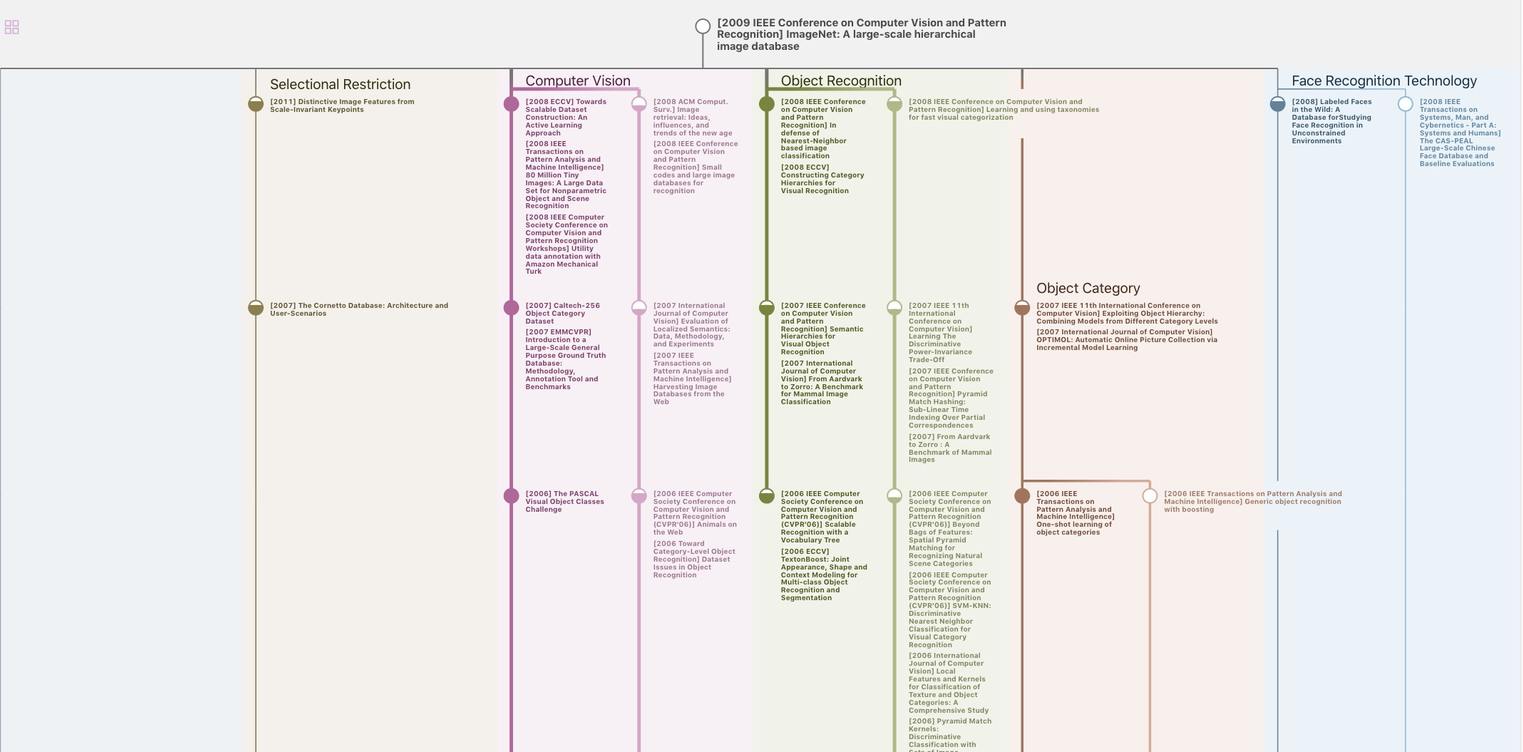
生成溯源树,研究论文发展脉络
Chat Paper
正在生成论文摘要