Removal of Confounding Factors using GA-SVM Feature Adaptation: Application on Detection of Vocal Fatigue thru sEMG Classification.
CEC(2023)
摘要
As machine learning solutions become increasingly more ubiquitous in medical diagnosis, researchers are becoming equally more aware of the possibility of confounded predictions being produced by these same models. This realization derives, for example, from the observation that sample-wise crossvalidation leads to highly underestimated error predictors when compared to subject-wise cross validation. However, without a reliable approach to remove spurious, confounding factors such as age, gender, or even the type/brand of equipment used, these same machine learning solutions will be fated to produce poor results despite the metrics for error estimation employed. In this research, we propose an optimization approach, using genetic algorithms, to adapt the feature vectors in order to maximize the prediction accuracy of a given classifiers, while minimizing the correlation between the features and the potential confounding factors. Our results, when applied to the diagnostic of vocal fatigue, have shown great improvement in terms of the generalization capability of the chosen SVM classifier. The system was evaluated using subject-wise (i.e. leave-one-subject-out) cross-validation, which demonstrated the effectiveness of this new confounding removal approach.
更多查看译文
关键词
surface electromyography,Genetic Algorithm,Support Vector Machine,Confounding Factor
AI 理解论文
溯源树
样例
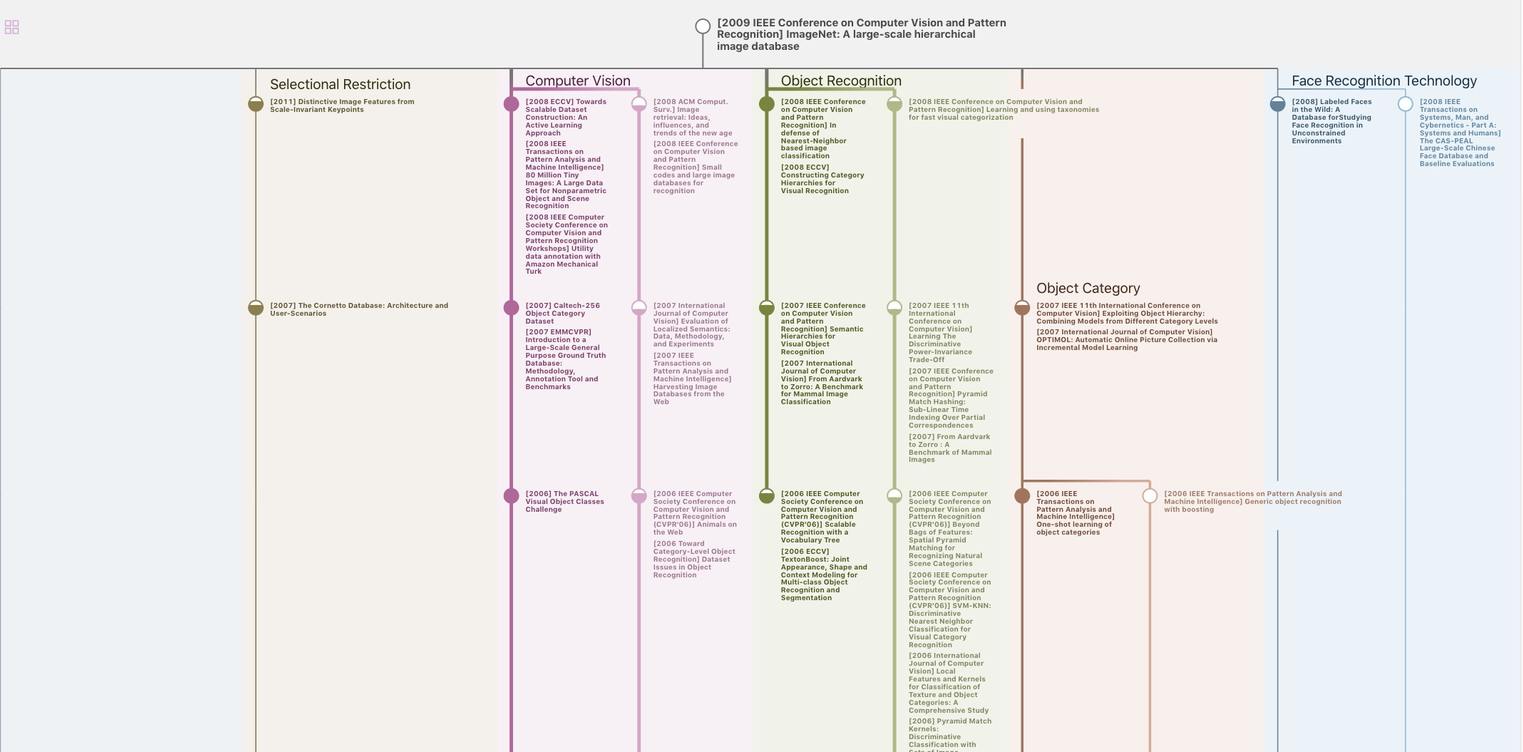
生成溯源树,研究论文发展脉络
Chat Paper
正在生成论文摘要