Full Index Deep Retrieval: End-to-End User and Item Structures for Cold-start and Long-tail Item Recommendation
PROCEEDINGS OF THE 17TH ACM CONFERENCE ON RECOMMENDER SYSTEMS, RECSYS 2023(2023)
摘要
End-to-end retrieval models, such as Tree-based Models (TDM) and Deep Retrieval (DR), have attracted a lot of attention, but they cannot handle cold-start and long-tail item recommendation scenarios well. Specifically, DR learns a compact indexing structure, enabling efficient and accurate retrieval for large recommendation systems. However, it is discovered that DR largely fails on retrieving coldstart and long-tail items. This is because DR only utilizes user-item interaction data, which is rare and often noisy for cold-start and long-tail items. Besides, end-to-end retrieval models are unable to make use of the rich item content features. To address this issue while maintaining the efficiency of DR indexing structure, we propose Full Index Deep Retrieval (FIDR) that learns indices for the full corpus items, including cold-start and long-tail items. In addition to the original structure in DR (called User Structure in FIDR) that learns with user-item interaction data (e.g., clicks), we add an Item Structure to embed items directly based on item content features (e.g., categories). With joint efforts of User Structure and Item Structure, FIDR makes cold-start items retrievable and also improves the recommendation quality of long-tail items. To our best knowledge, FIDR is the first to solve the cold-start and longtail recommendation problem for the end-to-end retrieval models. Through extensive experiments on three real-world datasets, we demonstrate that FIDR can effectively recommend cold-start as well as long-tail items, and largely promote overall recommendation performance without sacrificing inference efficiency. According to the experiments, the recall of FIDR is improved by 8.8%similar to 11.9%, while the inference of FIDR is as efficient as DR.
更多查看译文
关键词
Deep Retrieval,Cold-start,Long-tail
AI 理解论文
溯源树
样例
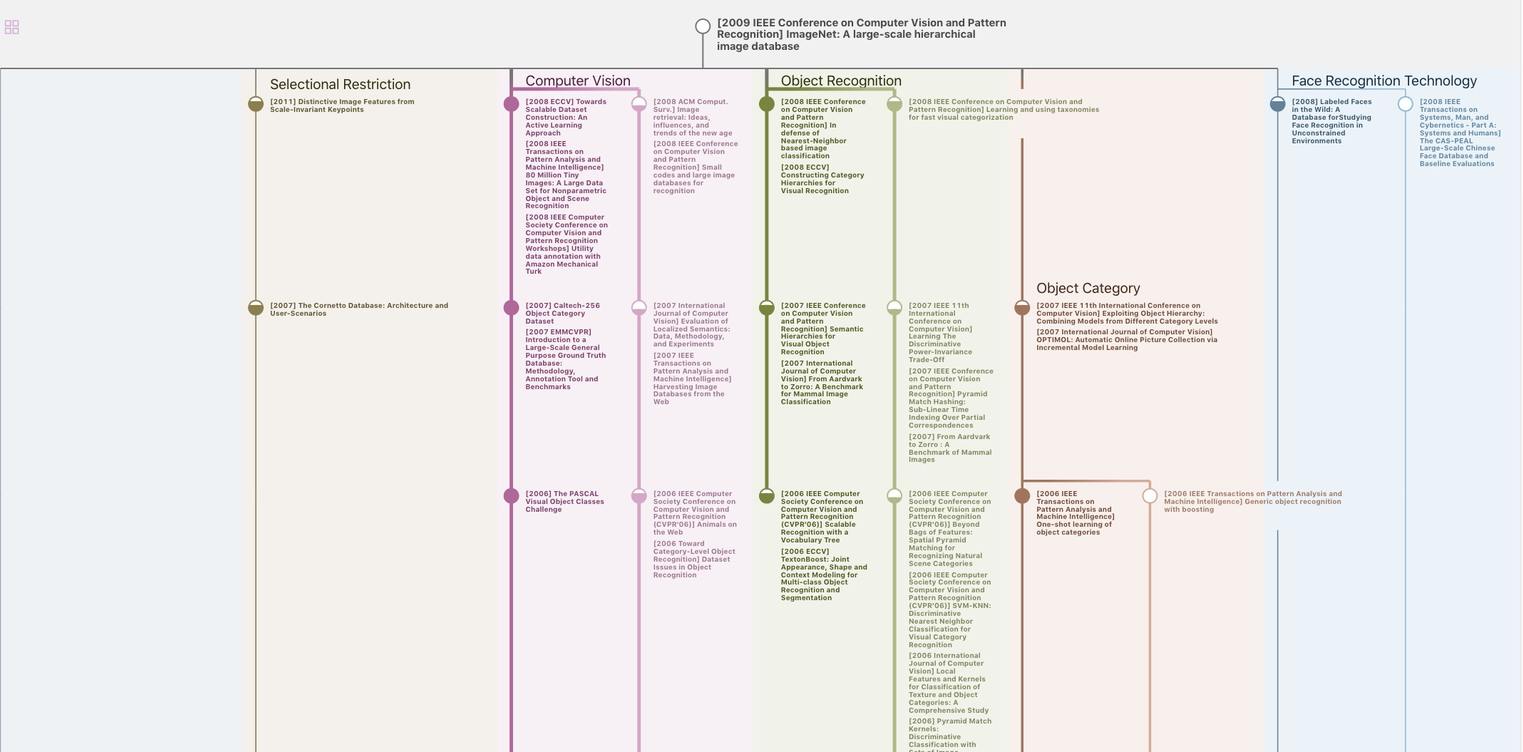
生成溯源树,研究论文发展脉络
Chat Paper
正在生成论文摘要