Trending Now: Modeling Trend Recommendations
PROCEEDINGS OF THE 17TH ACM CONFERENCE ON RECOMMENDER SYSTEMS, RECSYS 2023(2023)
摘要
Modern recommender systems usually include separate recommendation carousels such as 'trending now' to list trending items and further boost their popularity, thereby attracting active users. Though widely useful, such 'trending now' carousels typically generate item lists based on simple heuristics, e.g., the number of interactions within a time interval, and therefore still leave much room for improvement. This paper aims to systematically study this under-explored but important problem from the new perspective of time series forecasting. We first provide a set of rigorous definitions related to item trendiness and formulate the trend recommendation task as a one-step time series forecasting problem. We then propose a deep latent variable model, dubbed Trend Recommender (TrendRec), to forecast items' future trends and generate trending item lists. Furthermore, we design associated evaluation protocols for trend recommendation. Experiments on real-world datasets from various domains show that our TrendRec significantly outperforms the baselines, verifying our model's effectiveness.
更多查看译文
关键词
Recommender Systems,Trend Recommendation,Bayesian Deep Learning
AI 理解论文
溯源树
样例
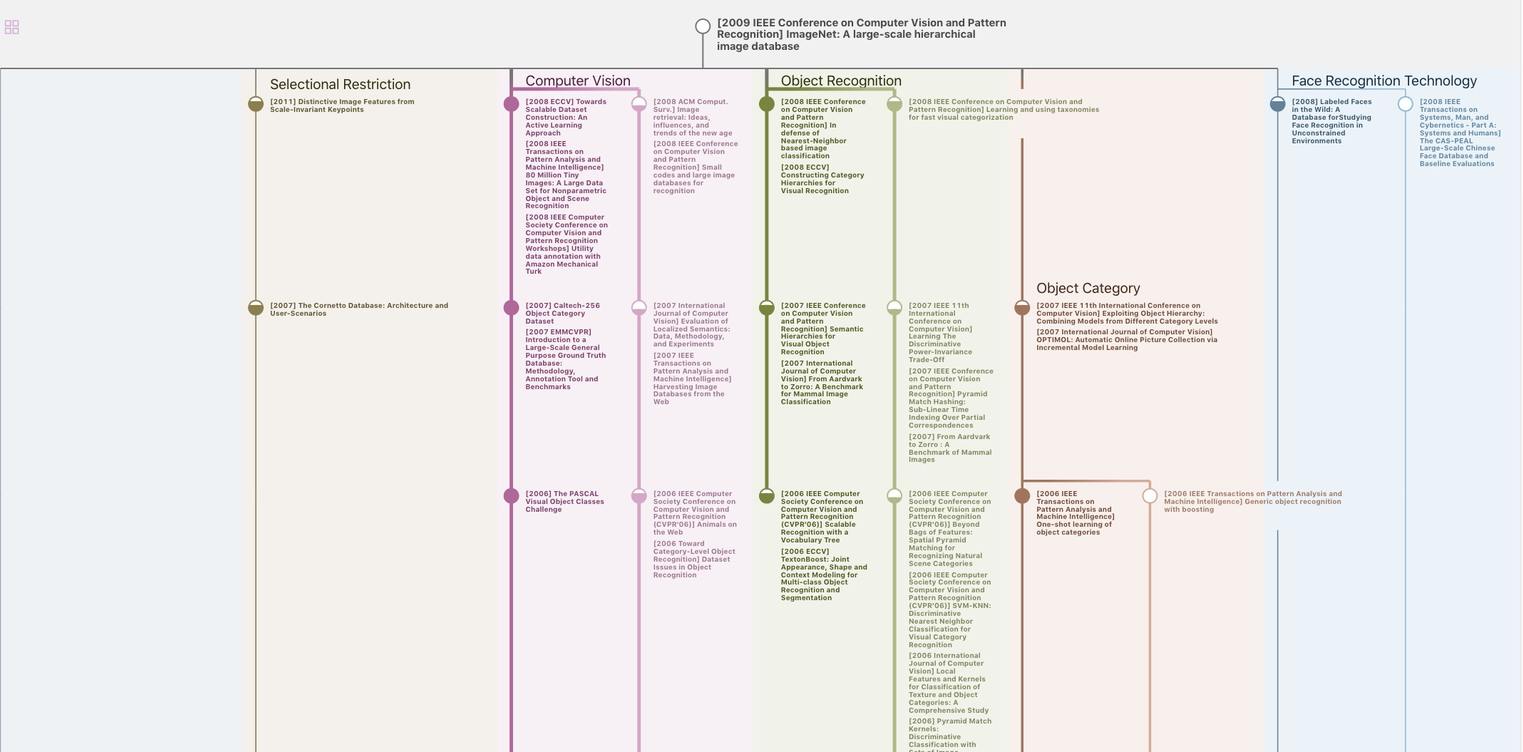
生成溯源树,研究论文发展脉络
Chat Paper
正在生成论文摘要