Buffer Awareness Neural Adaptive Video Streaming for Avoiding Extra Buffer Consumption.
INFOCOM(2023)
摘要
Adaptive video streaming has already been a major scheme to transmit videos with high quality of experience (QoE). However, the improvement of network traffics and the high compression efficiency of videos enable clients to accumulate too much buffer, which might cause colossal data waste if users close the session early before the session ends. In this paper, we consider buffer-aware adaptive bitrate (ABR) mechanisms to overcome the above concerns. Formulating the buffer-aware rate adaptation problem as multi-objective optimization, we propose DeepBuffer, a deep reinforcement learning-based approach that jointly takes proper bitrate and controls the maximum buffer. To deal with the challenges of learning-based buffer-aware ABR composition, such as infinite possible plans, multiple bitrate levels, and complex action space, we design adequate preference-driven inputs, separate action outputs, and invent high sample-efficiency training methodologies. We train DeepBuffer with a broad set of real-world network traces and provide a comprehensive evaluation in terms of various network scenarios and different video types. Experimental results indicate that DeepBuffer rivals or outperforms recent heuristics and learning-based ABR schemes in terms of QoE while heavily reducing the average buffer consumption by up to 90%. Extensive real-world experiments further demonstrate the substantial superiority of DeepBuffer.
更多查看译文
关键词
ABR schemes,average buffer consumption,buffer awareness neural adaptive video streaming,buffer-aware adaptive bitrate mechanisms,buffer-aware rate adaptation problem,colossal data waste,compression efficiency,deep reinforcement learning-based approach,DeepBuffer,high sample-efficiency training methodologies,learning-based buffer-aware ABR composition,maximum buffer,multiobjective optimization,network traffics,preference-driven inputs,QoE,quality of experience,real-world network traces,video types
AI 理解论文
溯源树
样例
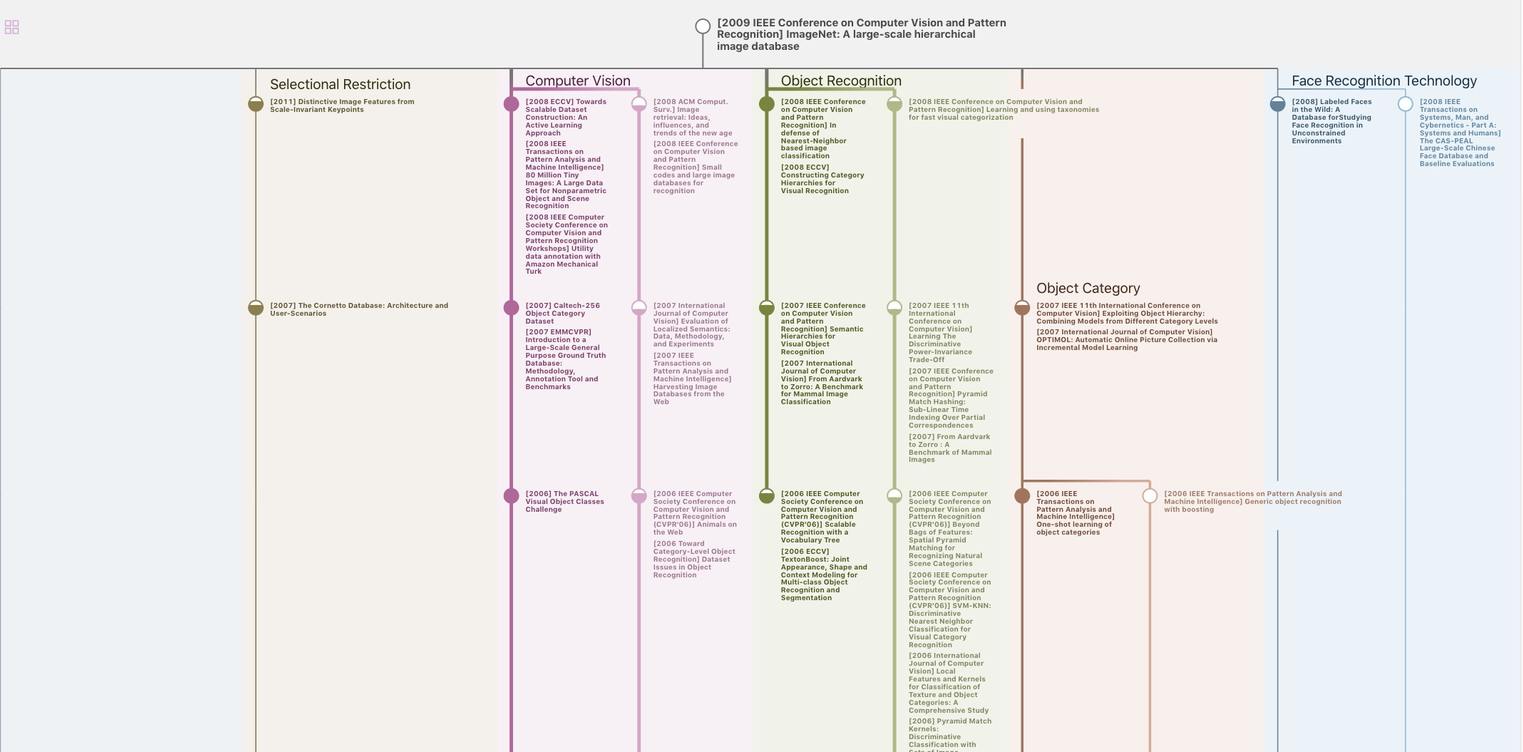
生成溯源树,研究论文发展脉络
Chat Paper
正在生成论文摘要