Understanding Peer Feedback Contributions Using Natural Language Processing.
EC-TEL(2023)
摘要
Peer feedback has been widely used in computer-supported collaborative learning (CSCL) setting to improve students’ engagement with massive courses. Although the peer feedback process increases students’ self-regulatory practice, metacognition, and academic achievement, instructors need to go through large amounts of feedback text data which is much more time-consuming. To address this challenge, the present study proposes an automated content analysis approach to identify relevant categories in peer feedback based on traditional and sequence-based classifiers using TF-IDF and content-independent features. We use a data set from an extensive course (N = 231 students) in the setting of engineering higher education. In particular, a total of 2,444 peer feedback messages were analyzed. The CRF classification model based on the TF-IDF features achieved the best performance. The results illustrate that the ability to scale up the automatic analysis of peer feedback provides new opportunities for student-improved learning and improved teacher support in higher education at scale.
更多查看译文
关键词
peer feedback contributions,processing
AI 理解论文
溯源树
样例
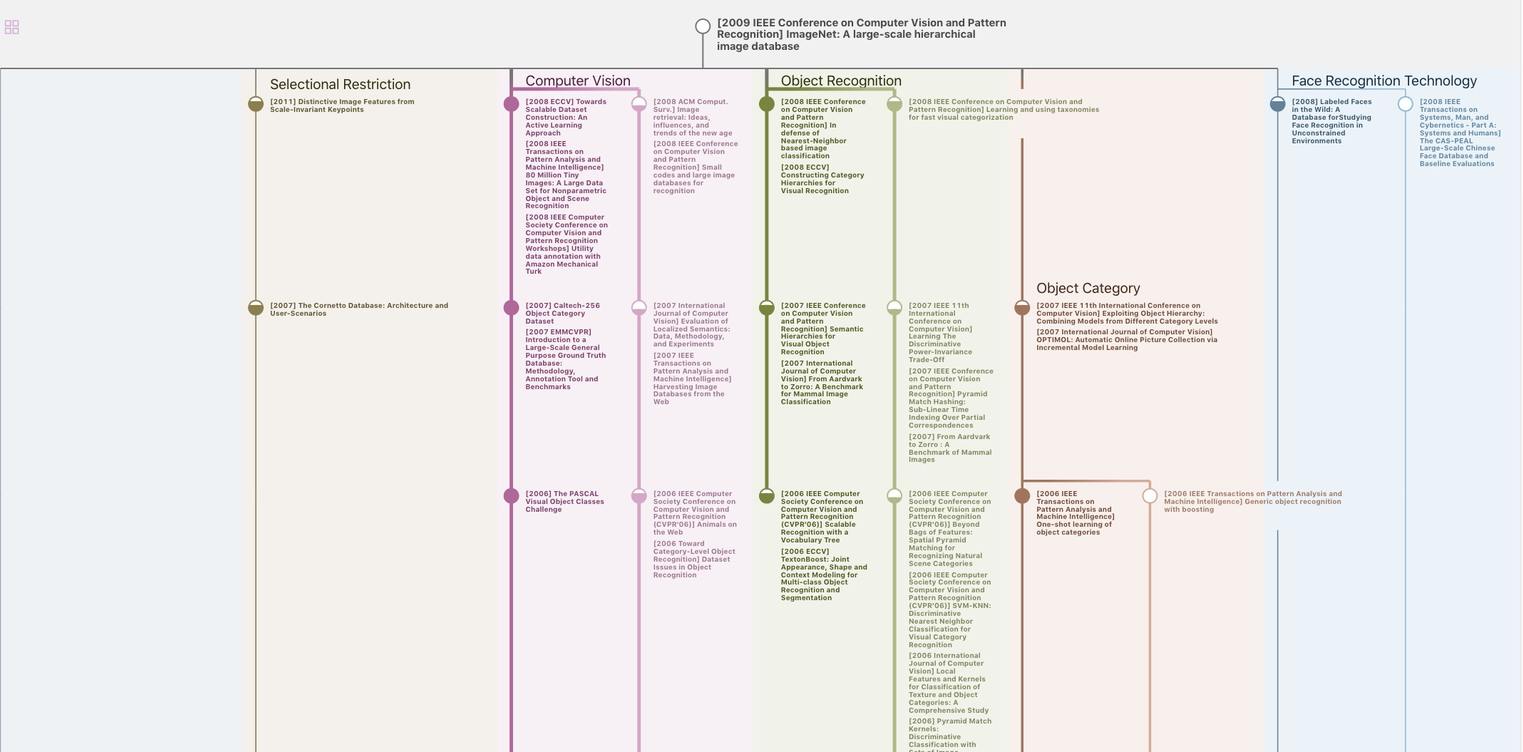
生成溯源树,研究论文发展脉络
Chat Paper
正在生成论文摘要