Ensemble Learning for Predicting Task Connectivity over Time in Cloud Data Centers.
INFOCOM Workshops(2023)
摘要
The rapid growth and interdependencies of cloud-hosted services and applications have made it imperative to optimize data center resource management while maintaining low operating costs. Inefficient resource use, rising energy consumption, and operating costs impact cloud provider's ability to provide high-quality services on an elastic basis. However, changing the selection and decision-making processes based on how the tasks are set up can improve scheduling and resource management in cloud data centers. In this paper, we develop a multivariate time series prediction model for task connectivity based on windowing characterization and ensemble learning methods. The high cardinality features are handled using a counter encoder, and the task trace data is transformed using a sliding window, from which features are extracted and used in conjunction with the task profile data to train and tune the candidate estimators. The best model outcomes are then used to construct an ensembled estimator. As part of the evaluation, a baseline comparison is performed in order to determine how well ensemble learning predicts task connectivity over time. The model outcomes are assisted using standard classification metrics such as accuracy, precision, and recall, including the F1 score, Kappa, and Matthews correlation coefficient. The results show that the proposed model outperformed the traditional models in most performance metrics, indicating the successful implementation of an ensemble learning approach for task connectivity predictions in large-scale cloud data centers.
更多查看译文
关键词
Task Connectivity Prediction,Ensemble Learning Methods,Windowing Characterization,Cloud-Hosted Services,Datacenter Resource Management
AI 理解论文
溯源树
样例
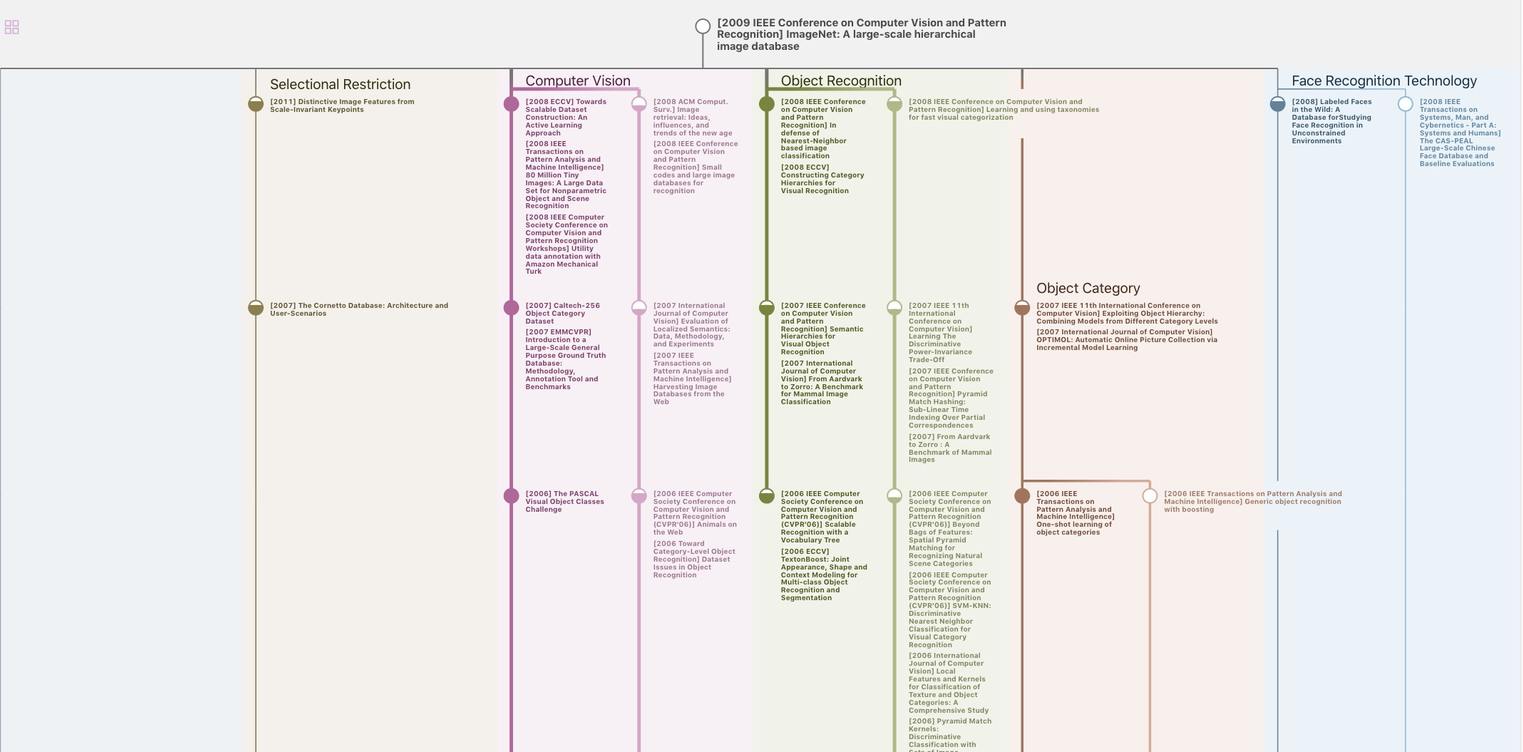
生成溯源树,研究论文发展脉络
Chat Paper
正在生成论文摘要