Weakly-supervised Semantic Segmentation on Historical Document Images.
RACS(2023)
摘要
Weakly-Supervised Semantic Segmentation (WSSS) has been widely studied as a feasible option to reduce the expensive annotation costs of deep semantic segmentation models. While numerous studies have proposed novel approaches for generating pseudo-labels and demonstrated their effectiveness, their domain is limited to natural scene images. There is a growing need for a comprehensive exploration of WSSS in the domain of document images, given the increasing number of digitized historical document images and the growing importance of document image segmentation for successful information retrieval. Importantly, document images possess inherent characteristics that distinguish them from natural scene images, rendering conventional image-level labels unsuitable. Consequently, the application of recent WSSS frameworks designed for natural scene images is limited. In this work, we propose a simple yet effective pseudo-label generation technique using content-adaptive geometric feature analysis. This approach enables the training of a segmentation model in a weakly-supervised manner without relying on image-level labels. Our method utilizes a Gravity-map, which can highlight potential regions of interest without requiring a priori-knowledge, serving as an initial coarse pixel-level label. The Gravity-map is subsequently refined through simple binarization and noise removal to form a pseudo-label. Finally, a segmentation model is trained using the generated pseudo-labels. Experimental results on the publicly available historical document collection demonstrate that the proposed pseudo-label generation technique offers a viable option for training the semantic segmentation model in the document image domain.
更多查看译文
AI 理解论文
溯源树
样例
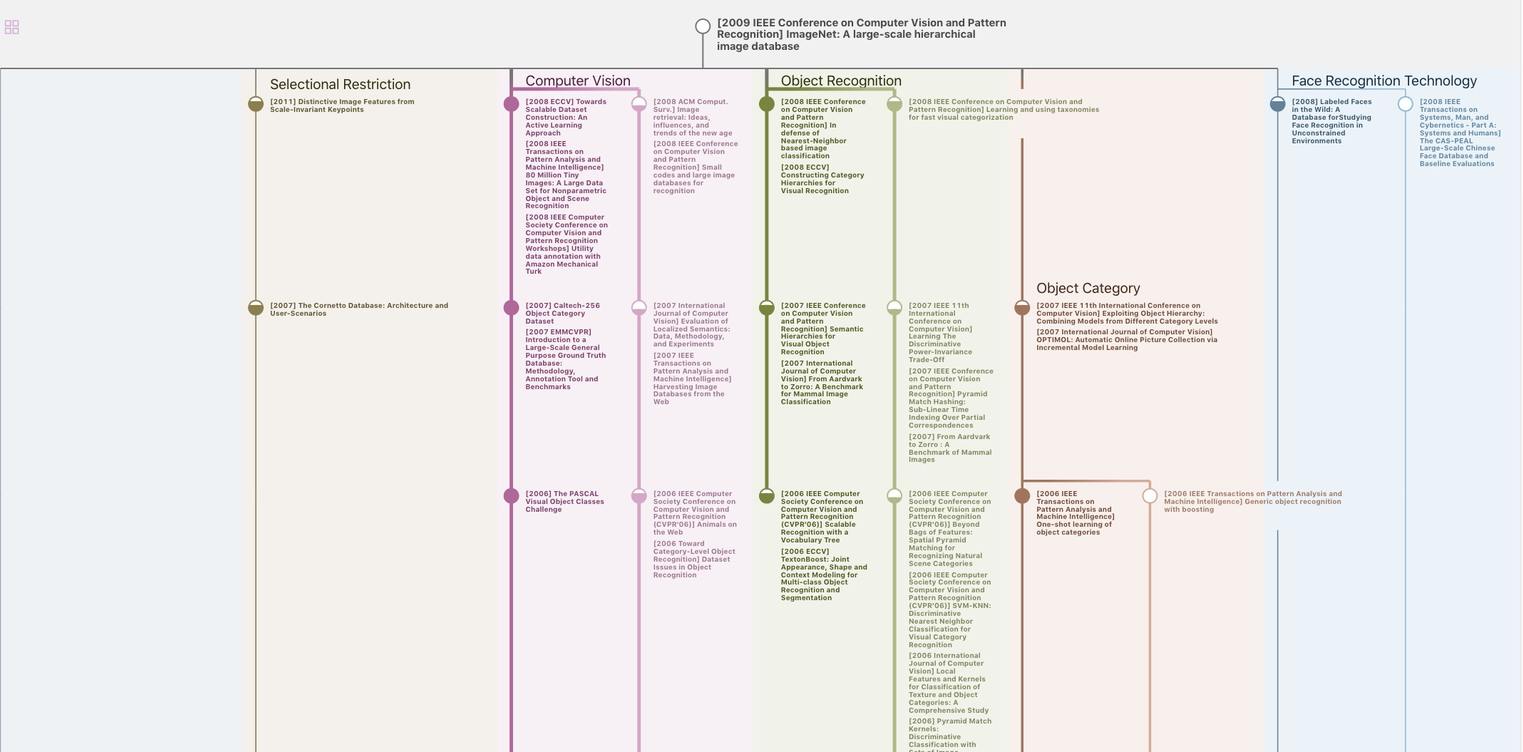
生成溯源树,研究论文发展脉络
Chat Paper
正在生成论文摘要