Multi Agent Reinforcement Learning Based Local Routing Strategy to Reduce End-to-End Delays in Segment Routing Networks.
INFOCOM Workshops(2023)
摘要
In this paper we propose a framework based on Deep Reinforcement Learning to proactively and autonomously take under control links loads in Segment Routing (SR) networks. The main idea is to monitor local link loads and, in case of anomalous situation, to execute local routing changes at milliseconds timescale. The solution proposed is based on a Multi Agent Reinforcement Learning (MARL) approach: a subset of nodes is equipped with a local agent, powered by a Deep Q-Network (DQN) algorithm, referred to as SRv6 rerouting for Local In-network Link Load Control (SR-LILLC). The main feature of SR-LILLC is to train the agents in a collaborative way, by defining a “shared” reward function, while working in an independent way during the operating phase. Moreover, the re-routing operation is performed in a transparent way for other network devices, without involving the centralized control plane, by exploiting the source routing feature of the SR. The performance evaluation conducted over real data sets shows that SR-LILLC is able to reduce the load on agents links without increasing the maximum link utilization of the network; moreover, the overall network performance are improved in terms of end-to-end delays.
更多查看译文
关键词
Self Driving Networks,Segment Routing,Deep Reinforcement Learning,Traffic Engineering
AI 理解论文
溯源树
样例
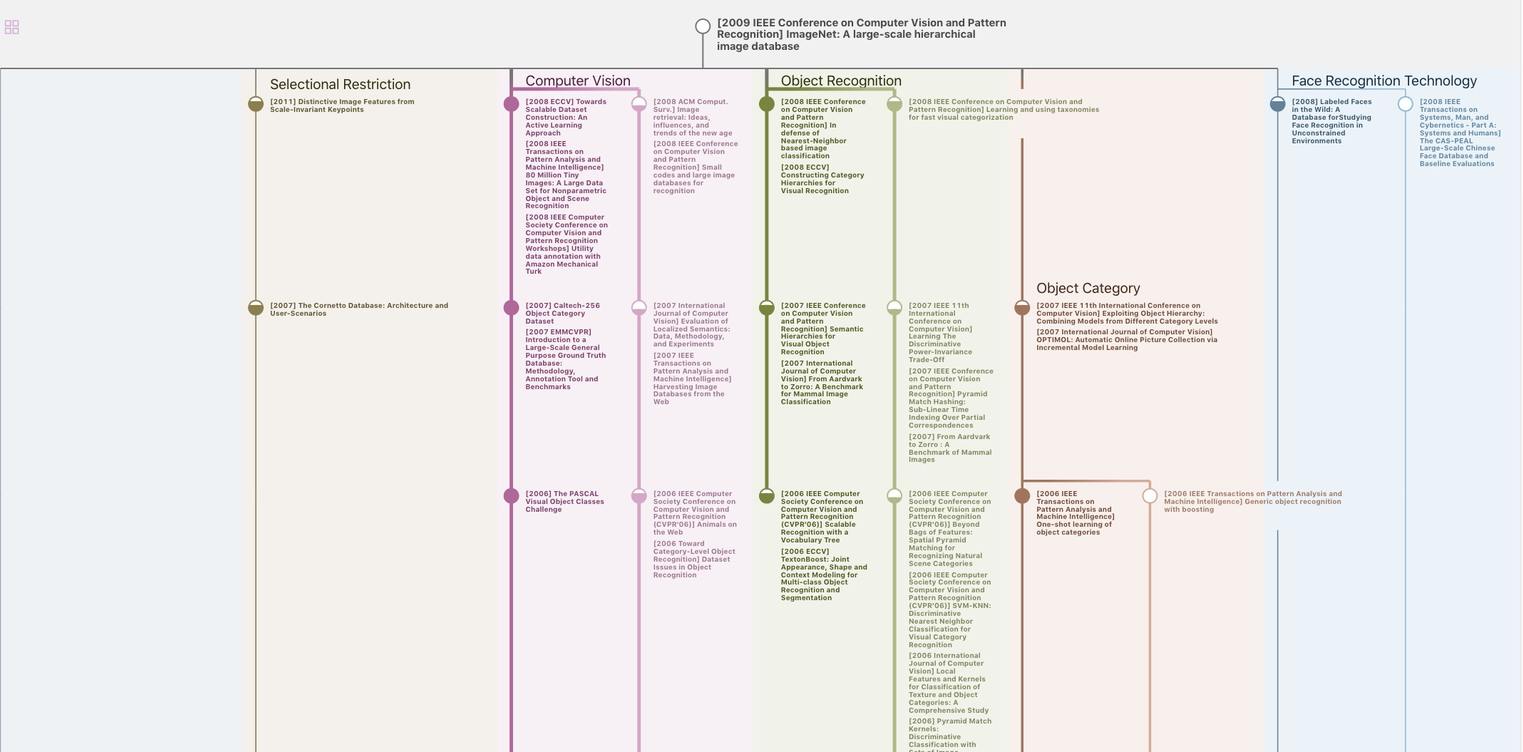
生成溯源树,研究论文发展脉络
Chat Paper
正在生成论文摘要