AFNFA: An Approach to Automate NCCL Configuration Exploration
PROCEEDINGS OF THE 7TH ASIA-PACIFIC WORKSHOP ON NETWORKING, APNET 2023(2023)
摘要
With the continuously increasing scale of deep neural network models, there is a clear trend towards distributed DNN model training. State-of-the-art training frameworks support this approach using collective communication libraries such as NCCL, MPI, Gloo, and Horovod. These libraries have many parameters that can be adjusted to fit different hardware environments, and these parameters can greatly impact training performance. Therefore, careful tuning of parameters for each training environment is required. However, given the large parameter space, manual exploration can be time-consuming and laborious. In this poster, we introduce AFNFA, which stands for AI For Network For AI. It is an automated program that utilizes machine learning and simulated annealing to explore NCCL parameters. Preliminary evaluation results demonstrate that compared to the default configuration, the configuration explored by AFNFA improves NCCL communication performance by 22.90%.
更多查看译文
AI 理解论文
溯源树
样例
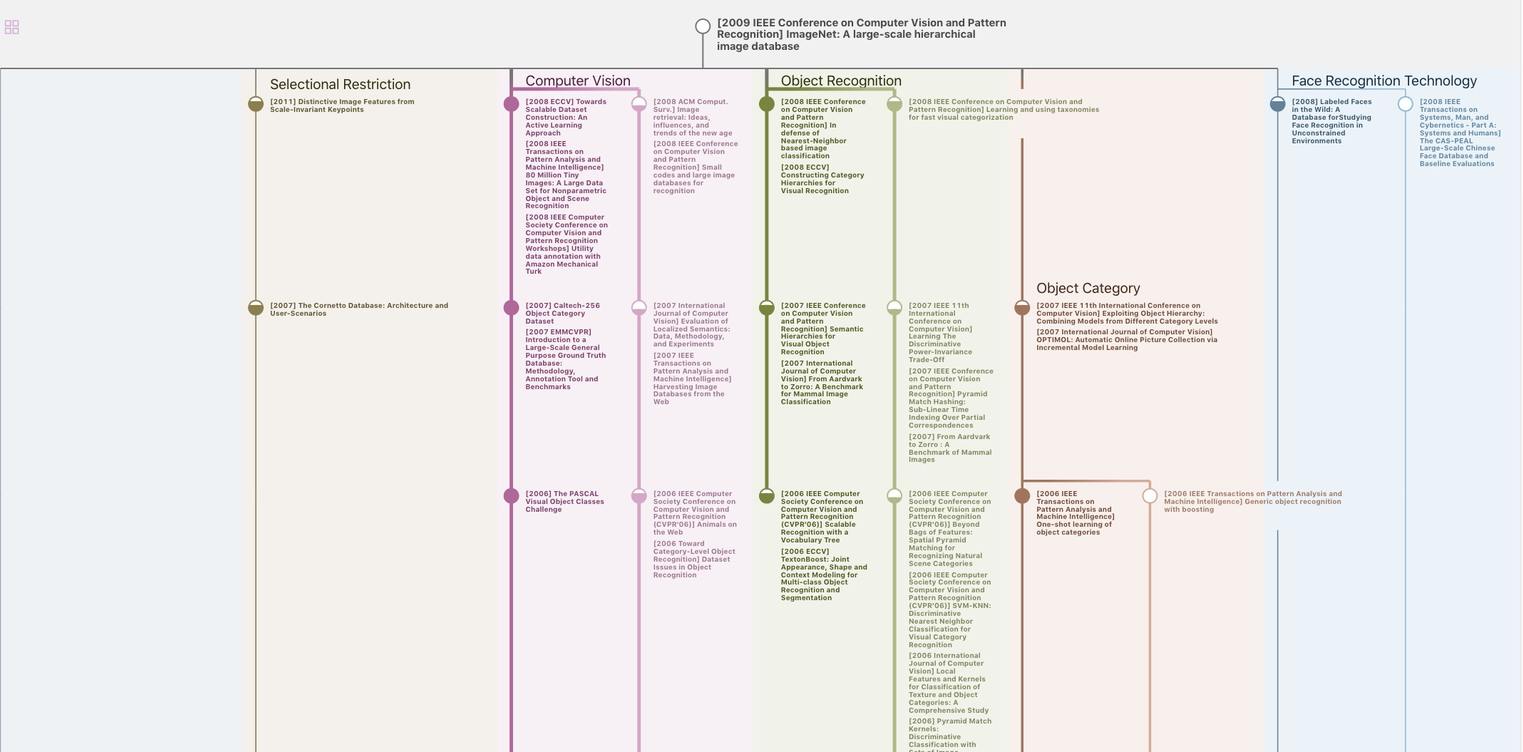
生成溯源树,研究论文发展脉络
Chat Paper
正在生成论文摘要