In-Network Probabilistic Monitoring Primitives under the Influence of Adversarial Network Inputs
PROCEEDINGS OF THE 7TH ASIA-PACIFIC WORKSHOP ON NETWORKING, APNET 2023(2023)
摘要
Network management tasks heavily rely on network telemetry data. Programmable data planes provide novel ways to collect this telemetry data efficiently using probabilistic data structures like bloom filters and their variants. Despite the benefits of the data structures (and associated data plane primitives), their exposure increases the attack surface. That is, they are at risk of adversarial network inputs. In this work, we examine the effects of adversarial network inputs to bloom filters that are integral to data plane primitives. Bloom filters are probabilistic and inherently susceptible to pollution attacks which increase their false positive rates. To quantify the impact, we demonstrate the feasibility of pollution attacks on FlowRadar, a network monitoring and debugging system that employs a data plane primitive to collect traffic statistics. We observe that the adversary can corrupt traffic statistics with a few well-crafted malicious flows (tens of flows), leading to a 99% drop in the accuracy of the core functionality of the FlowRadar system.
更多查看译文
关键词
Network security,Programmable data planes,Probabilistic data structures,Bloom filters,Adversarial influence
AI 理解论文
溯源树
样例
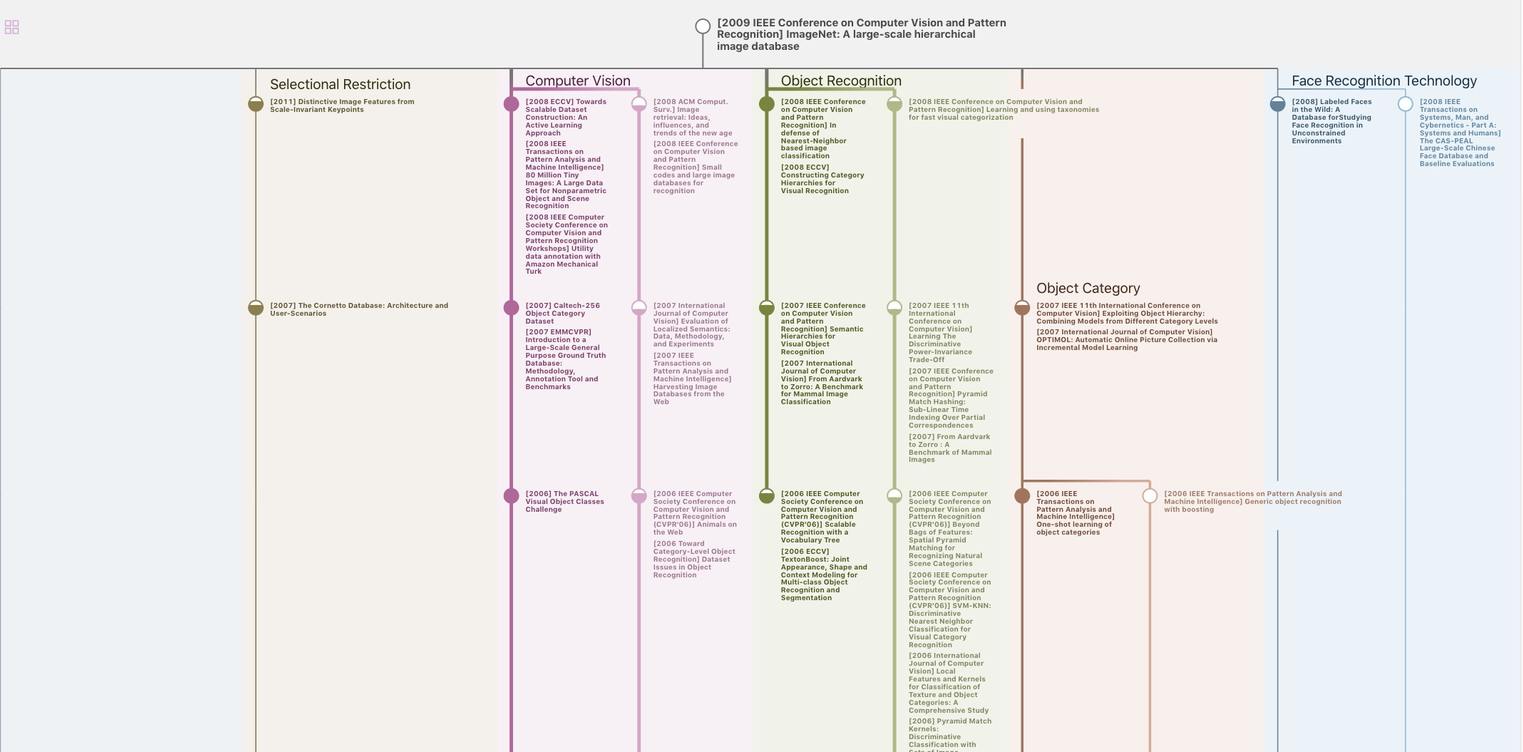
生成溯源树,研究论文发展脉络
Chat Paper
正在生成论文摘要