Dynamic Graph Convolutional Transformer for Short-term Wind Speed Forecasting.
ICCAI(2023)
摘要
Wind speed forecasting is still a challenging problem, especially considering the correlations of spatial and temporal domains. However, the changing properties of spatial dependencies over time are ignored in most existing algorithms. In this paper, we propose a novel spatio-temporal machine learning algorithm, named Dynamic Graph Convolutional Transformer (DGCT), for wind speed forecasting. The key contribution of the proposed method is that graph convolutional networks are embedded into self-attention layers of Transformer to capture spatio-temporal correlations to improve the accuracy of forecasting. For the changing properties of spatial dependencies, we model the spatial dependencies as a mixture of global and localized patterns, which are represented by static and dynamic matrices respectively. Moreover, an auxiliary network is designed to generate the dynamic matrix, which further improve the forecasting accuracy. Experiments on two real-world datasets demonstrate that the proposed method outperformed other existing methods consistently.
更多查看译文
AI 理解论文
溯源树
样例
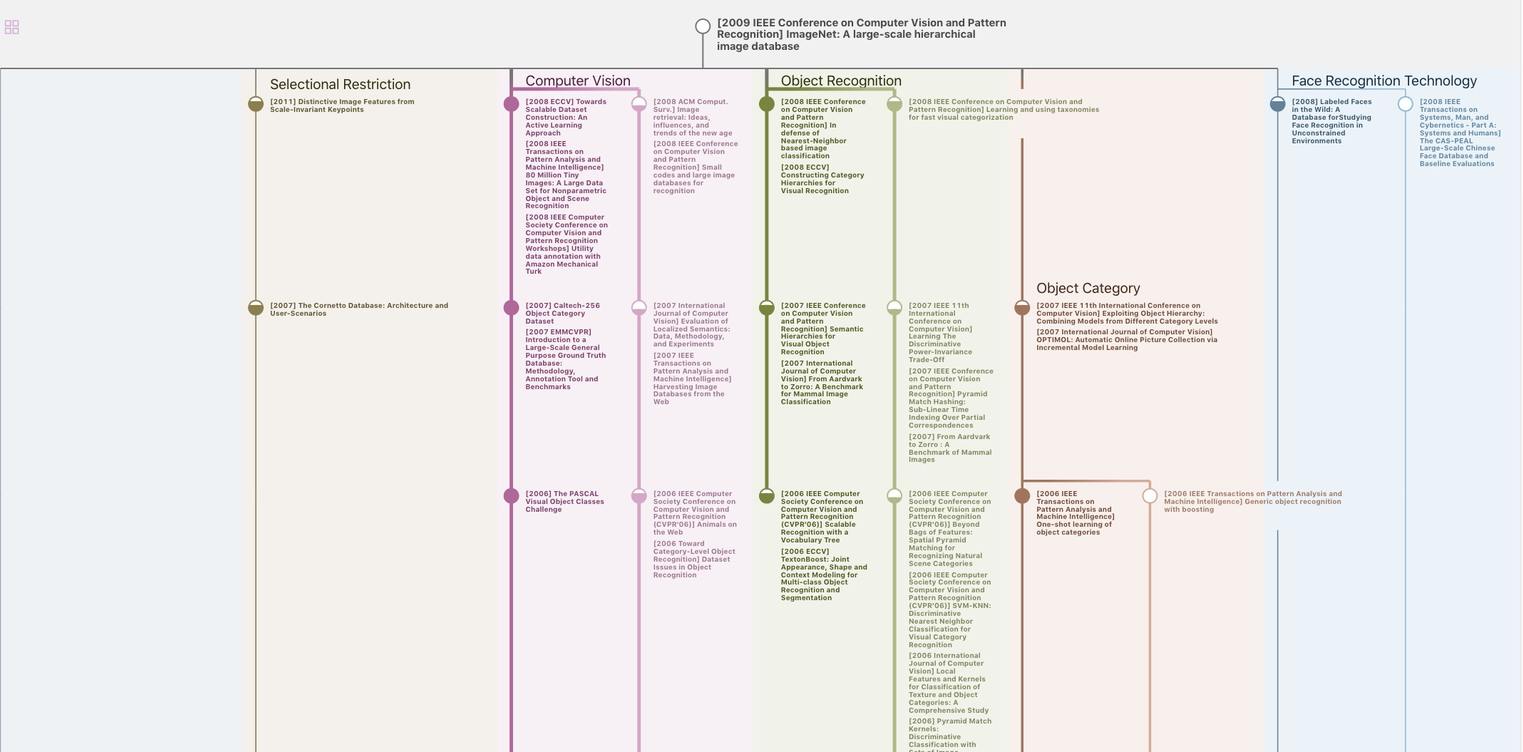
生成溯源树,研究论文发展脉络
Chat Paper
正在生成论文摘要