Adaptive Random Forest with Dynamic Detectors for Evolving Data Stream Classification.
ICCAI(2023)
摘要
Evolving data streams containing concept drift breaks the assumption that data are independent and identically distributed (IID) in traditional machine learning models. A series of ensemble-based models have been adapted to data stream learning tasks due to the abilities of dynamic partially update and cooperate with drift detection methods. Adaptive Random Forests (ARF), one of the state-of-the-art in the stream setting, encounters high resource requirements in both efficiency and memory like all ensemble methods. We are committed to reducing running cost without compromising ARF’s performance. In this paper, we propose the Adaptive Random Forest with Dynamic Detectors (ARFDD) which utilizes diversity measurement to combine homogeneous detectors and split heterogeneous ones accordingly, leads to lower computing costs and thus speed up in applications. Experiments on all 8 synthetic and 4 real datasets show a great CPU-time thrift while avoiding performance degradation.
更多查看译文
AI 理解论文
溯源树
样例
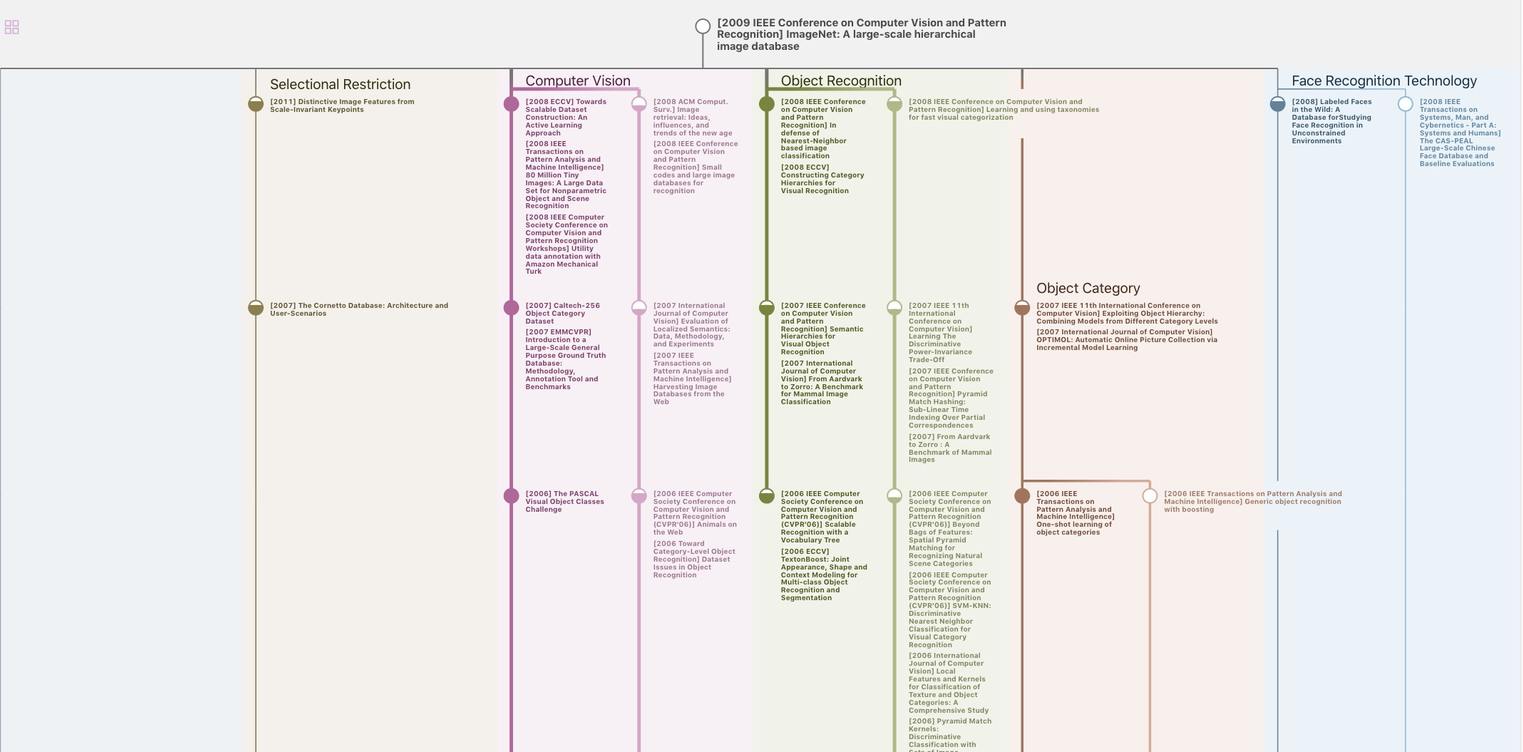
生成溯源树,研究论文发展脉络
Chat Paper
正在生成论文摘要