Hyper-Accelerated Learning for Brain-Computer Interfaces via Partial Target-Aware Optimal Transport.
Workshop on Smart Wearable Systems and Applications(2023)
摘要
Brain-computer interfaces (BCIs) have surfaced as a powerful modality in human-machine interaction and wearable technology with powered futuristic applications like virtual reality, robot control, gaming, etc. Using BCIs, the brain's intent can be harnessed without explicit communication. Despite the vast promise, systems designed for BCIs generalize poorly to new or unseen individuals due to high variability in brain signals among different subjects, resulting in long retraining/calibration sessions. This lack of generalization is typically attributed to a covariate shift of signals in the probability space, which manifests itself as disparate marginal and class conditional distributions. In this paper, we overview the factors contributing to poor generalization on a more granular level by analyzing a specific brain signal called the Error Potential (ErrP), a signal well-known for its noisy characteristics and high variability, and propose a novel algorithm to mitigate the associated covariate shift using partial target-aware optimal transport. We demonstrate our method on an ErrP dataset collected in our lab. Our method outperforms state-of-the-art models for cross-user generalization which translates to a reduction in calibration time by an order of magnitude.
更多查看译文
AI 理解论文
溯源树
样例
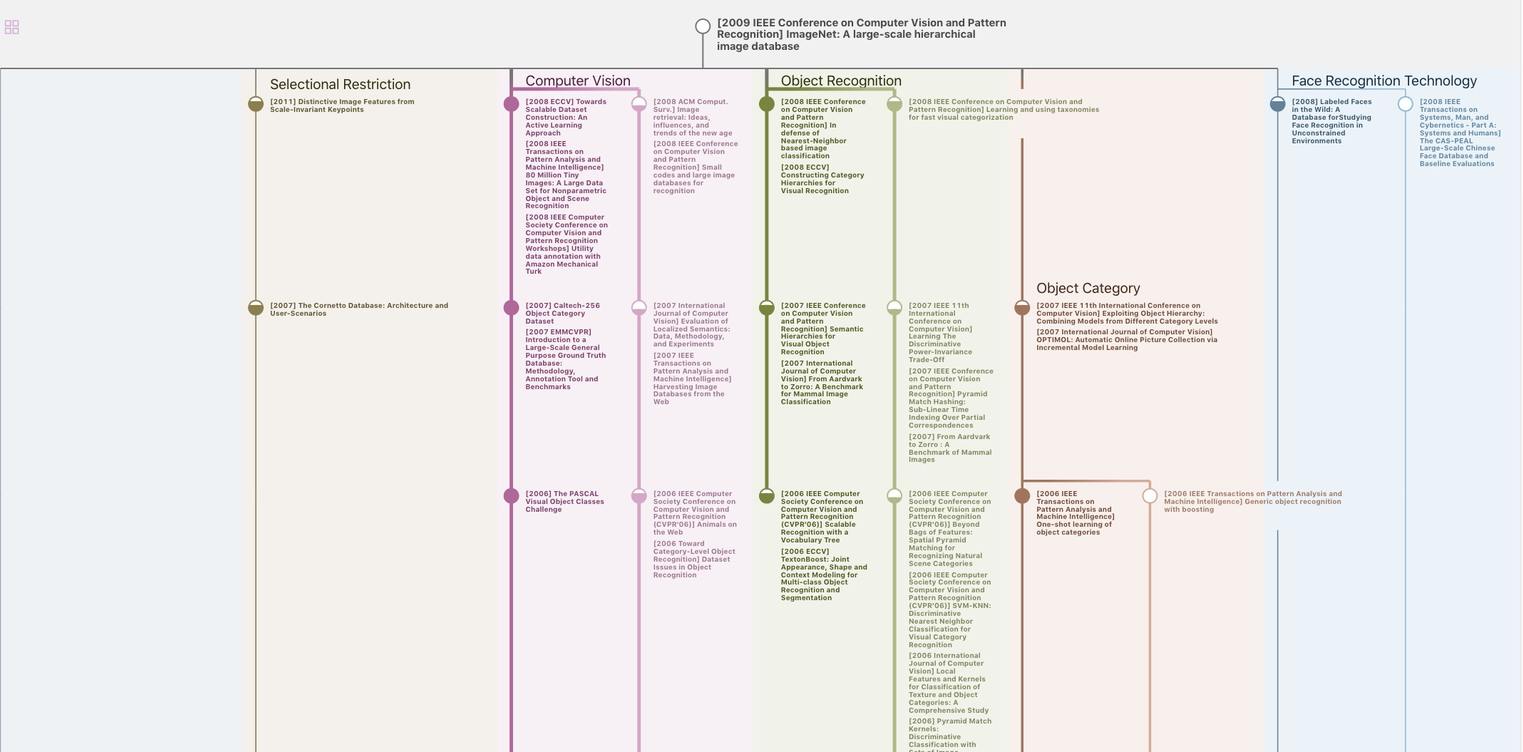
生成溯源树,研究论文发展脉络
Chat Paper
正在生成论文摘要