GBSMOTE: A Robust Sampling Method Based on Granular-ball Computing and SMOTE for Class Imbalance.
ICMAI(2023)
摘要
In recent years, the imbalanced classification problem has received much attention. SMOTE is one of the most popular methods to improve the performance of unbalanced data classification models. SMOTE changes the data distribution of unbalanced data sets by adding a few generated class samples, but the SMOTE algorithm has some limitations of its own, which may lead to problems such as the generated samples are noisy, the generated samples aggravate the boundary blurring, etc., which are especially obvious in the presence of samples with label noise. Granular-ball computing is an efficient, robust and scalable modeling method developed in the field of granular computing in recent years, and we can obtain clear decision boundaries by dividing data sets through granular-ball. Accordingly, this paper proposes a method, called Granular-ball SMOTE(GBSMOTE),to solve the above problems by first dividing the data set by granular-ball computing and then using SMOTE oversampling inside the granular-ball. The experimental results show the effectiveness of the proposed method, which is more prominent in the samples with label noise.
更多查看译文
AI 理解论文
溯源树
样例
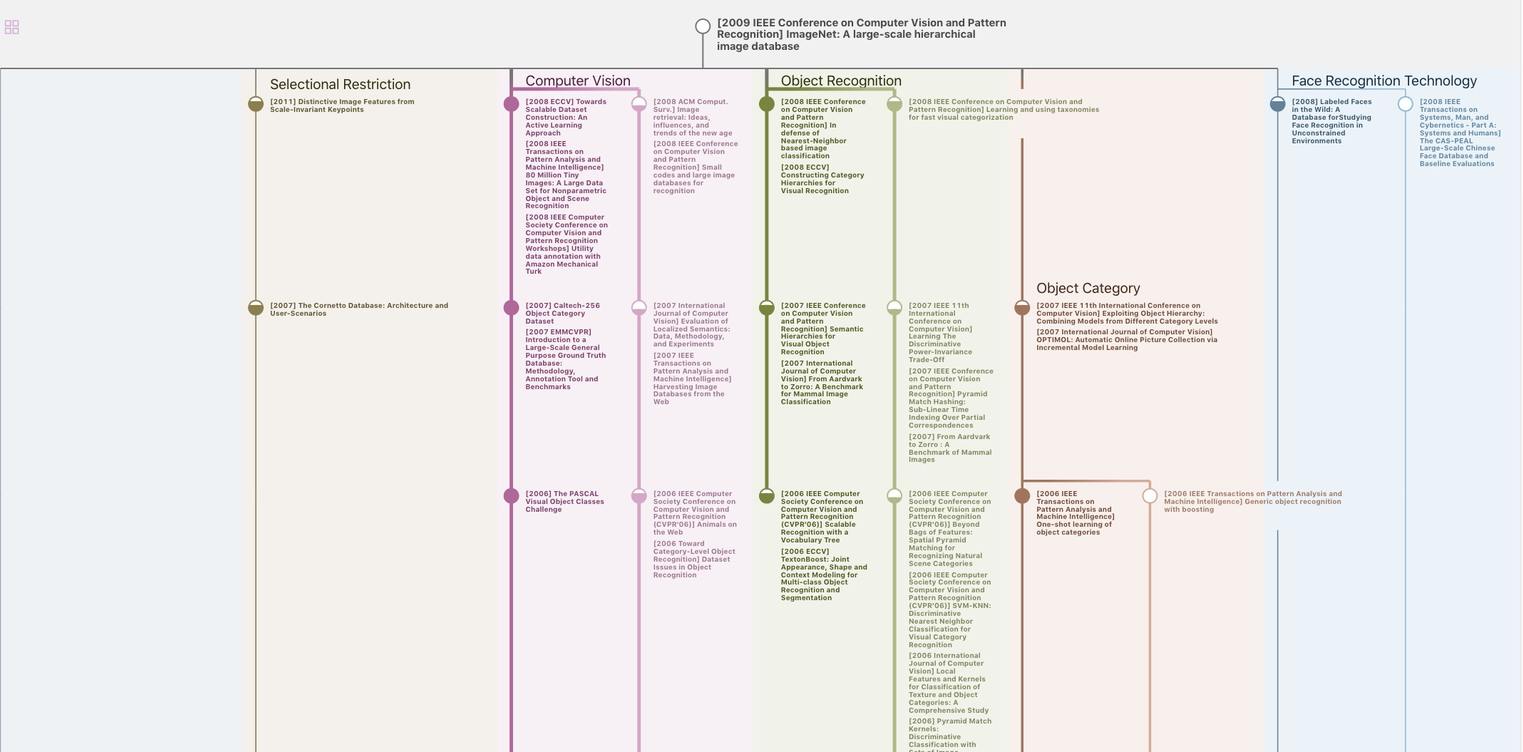
生成溯源树,研究论文发展脉络
Chat Paper
正在生成论文摘要