Assessing Urban Resilience to Flooding at County Level Using Multi-Modal Geospatial Data.
Agro-Geoinformatics(2023)
摘要
Urban resilience refers to the capacity of an urban system to adapt and respond to changes, including the ability to better cope with future disaster risks. With the intensifying impact of global climate change, cities are becoming more vulnerable to natural disasters. It is crucial for cities to effectively resist and maintain sustainable economic and social development in the face of these disasters. This paper, taking the “2021.07.20 Henan rainstorm” flood disaster in the Weihe river basin as a study case, applying Sentinel-1 (S1) synthetic aperture radar (SAR) images and other multi-modal geospatial data, aims to assess county-scale urban resilience against flooding. First, the random forest classifier was adopted to extract water bodies at periods of pre-flood, during-flood and post-flood from the preprocessed S1 data. Second, the flood recovery rate (FRR) was chosen for representing urban flood resilience, and was calculated at county-level based on the water bodies of the three periods. Third, data of the 12 factors of social, economic, community and environment dimensions were collected and transformed, and were used to explore and evaluate the main impacting factors on county-level FRRs with the aid of Pearson correlation analysis and principal component analysis (PCA). The results show that: 1) Districts in the southwest have higher recovery levels, while districts in the east have lower recovery levels. 2) The four factors of points of interest (POI) all have significant positive effects on FRR, while topography and slope have considerable negative impacts on FRR. 3) The distribution of FRR and the weights of factors’ influence on FRR can be combined for developing relevant policies for enhancing urban flood resilience.
更多查看译文
关键词
Urban resilience,Flood recovery rate,Multi-modal geospatial data,Random forest classification,Sentinel-1 image,Points of interest (POI),Principal component analysis (PCA)
AI 理解论文
溯源树
样例
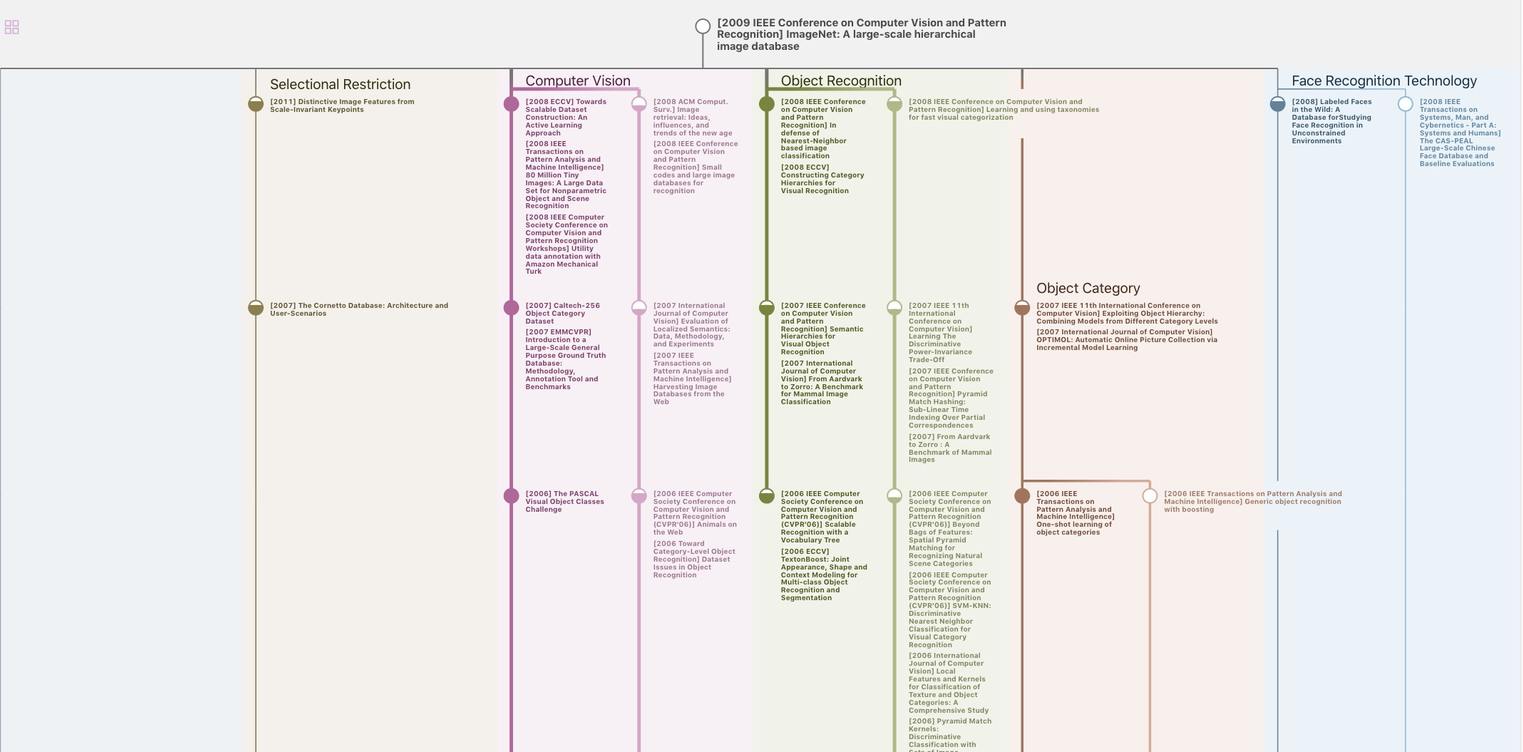
生成溯源树,研究论文发展脉络
Chat Paper
正在生成论文摘要