Accurate extraction of fragmented field boundaries using classification-assisted and CNN-based semantic segmentation methods.
Agro-Geoinformatics(2023)
摘要
The accurate extraction of cropland field parcels in fragmented agricultural landscapes poses significant challenges due to the small sizes and irregular shapes of the fields. Here, we proposed a hierarchical framework for delineating agricultural field boundaries based on the level of field fragmentation. Firstly, a modified Recurrent Residual Convolutional Neural Network based on U-Net (R2U-Net) was applied to delineate the agricultural field boundaries. Then, a rule-based decision fusion strategy assisted with a classification method was developed to address the under-segmentation problem in Plains-Basin scenes, and an adaptive generalized buffer algorithm (AGBA) was used to address the incomplete-segmentation problem in Plateau-Hilly scenes. We conducted tests on the proposed methods in Yangming County, Heilongjiang Province, China, using time series of Sentinel-2 images with a spatial resolution of 10-m. The results demonstrate the outstanding performance of the proposed method in delineating fragmented field boundaries, with an overall accuracy of 85.29% and F1 score of 0.84 in fragmented areas. The methods proposed in this study provide a practical solution for accurately extracting field boundaries in highly fragmented and heterogeneous agricultural landscapes.
更多查看译文
关键词
Cropland,field boundary,time-series data,Sentinel-2,fragmented agricultural landscape
AI 理解论文
溯源树
样例
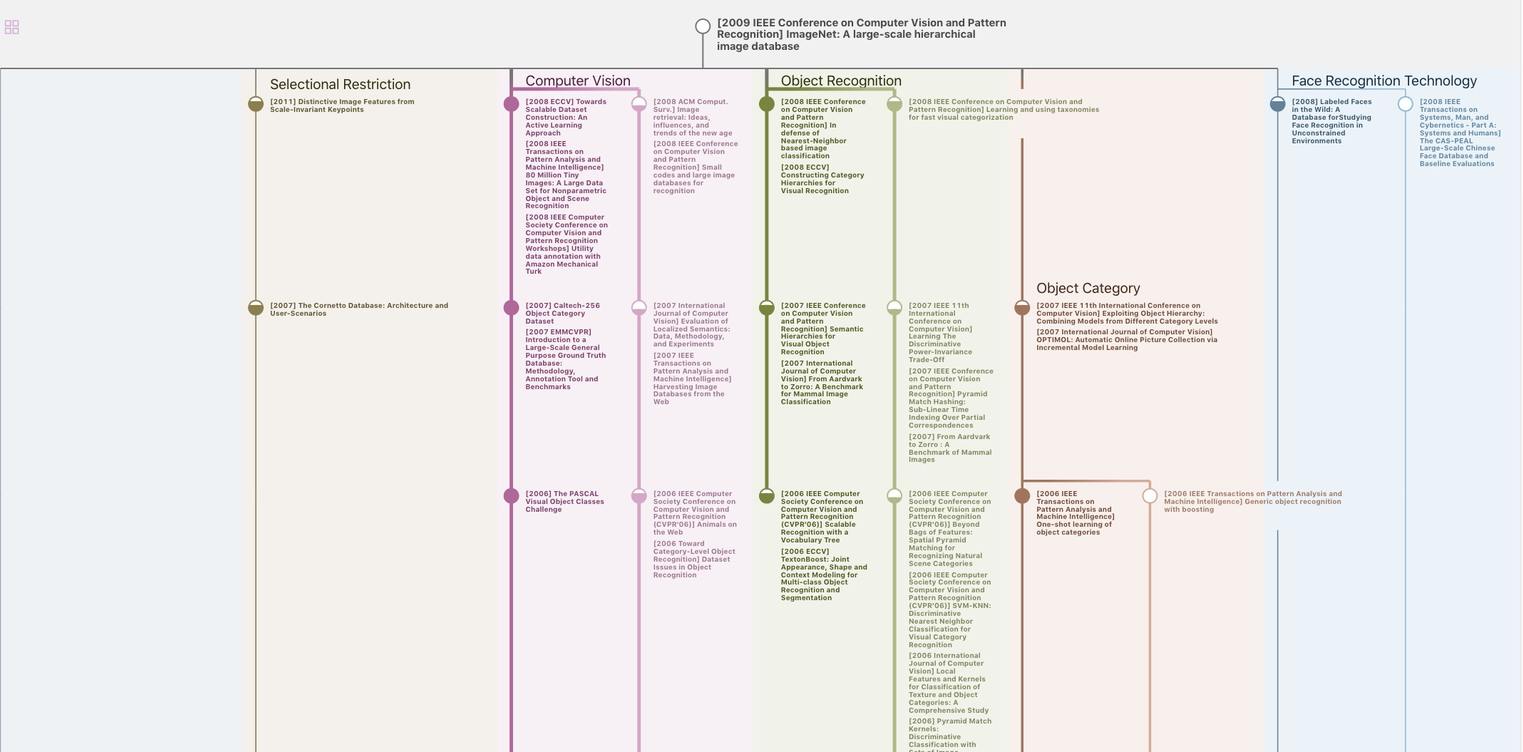
生成溯源树,研究论文发展脉络
Chat Paper
正在生成论文摘要