Streaming Session Recommendation Based on User's Global Attributes.
ISCC(2023)
摘要
Session-based recommendations are designed to predict the next item a user will click on. It is able to maintain the session structure of the session data. In practical scenarios, session data is dynamic and rapidly generated, which suggests the streaming nature of session data. Recent studies have shown that graph neural network (GNN) based approaches mainly focus on the current session and cannot process the latest session data. In this paper, we propose a streaming session-based recommendation system (UGNN). We use the Wasserstein model for data sampling. Then, the user configuration is embedded as a global attribute in the session graph and incorporated into the embedding vector of the recommendation system. Meanwhile, since the residual network of the Transformer model can effectively alleviate the pressure during model updates, we innovatively added Transformers to the model. Experiments on two real-world datasets show that our model outperforms state-of-the-art models.
更多查看译文
关键词
Session Recommendation,Graph Neural Network,Streaming Session-based Recommendation,Transformer
AI 理解论文
溯源树
样例
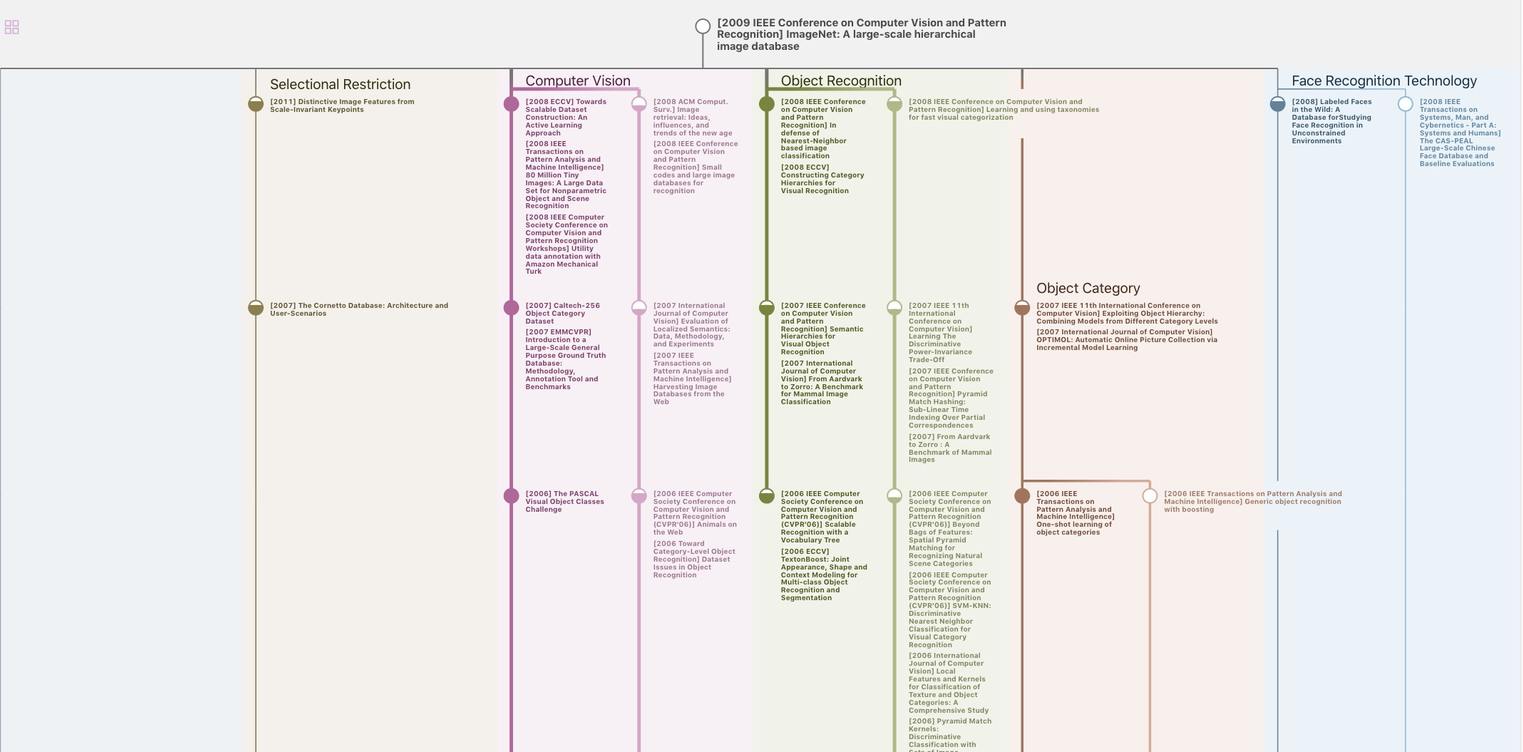
生成溯源树,研究论文发展脉络
Chat Paper
正在生成论文摘要