An Enhanced Subregion Dominance Relation for Evolutionary Many-Objective Optimization.
NCAA (1)(2023)
Abstract
Pareto dominance-based approach is a classical method for solving multi-objective optimization problems (MOPs). However, as the number of objectives increases, the selection pressure drops sharply. Solutions with good convergence and diversity are hardly obtained. To tackle these issues, this paper proposes an enhanced subregion dominance (called ESD-dominance) relation for evolutionary many-objective optimization. In ESD-dominance, individuals in the population are associated with a set of uniform reference vectors according to the Euclidean distance. Individuals associated with the same reference vector constitute a subregion. To enhance the convergence, each subregion is re-layered based on a new convergence metric. To maintain the diversity, the density in different subregions is considered. In order to validate the performance of ESD-dominance, a modified NSGA-II (called ESD-NSGA-II) algorithm is constructed based on the proposed dominance relation. In the experiments, a set of WFG benchmark problems with 3, 5, 8, and 15 objectives are tested. Computational results show the competitiveness of ESD-NSGA-II when compared with eight other state-of-the-art algorithms.
MoreTranslated text
Key words
enhanced subregion dominance relation,optimization,evolutionary,many-objective
AI Read Science
Must-Reading Tree
Example
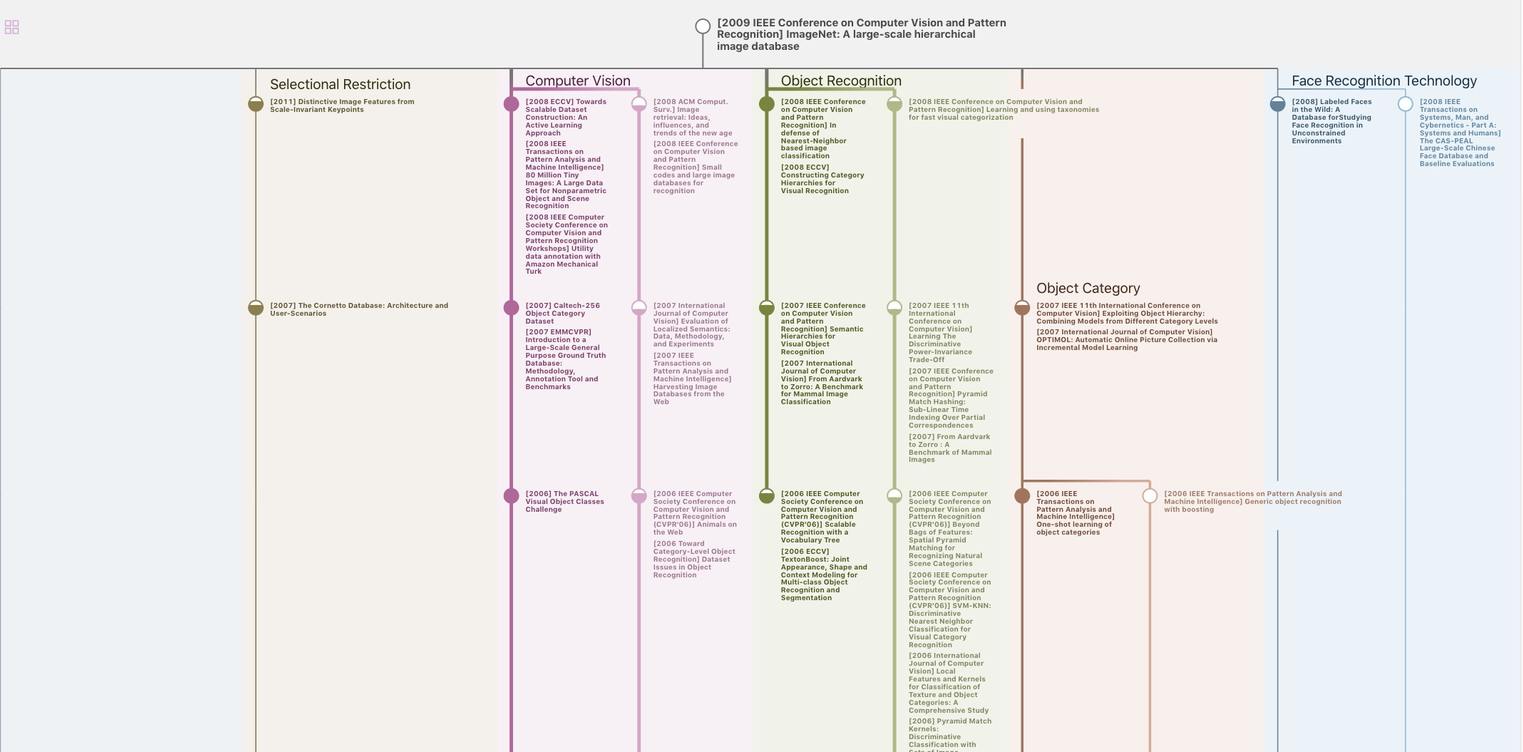
Generate MRT to find the research sequence of this paper
Chat Paper
Summary is being generated by the instructions you defined