Leveraging Deep Reinforcement Learning for Geolocation-based MIMO Transmission in FD-RAN
ICCC(2023)
摘要
In the fully-decoupled radio access network (FD-RAN), the base stations (BSs) are classified as control BSs, uplink BSs, and downlink BSs for better flexibility of the BS-user equipment (UE) association and elasticity of resource scheduling. However, the high feedback delay brought by the physically decoupling architecture renders conventional feedback-based MIMO transmission ineffective. Considering the strong correlation between user geolocation and the channel propagation environment, especially when a line-of-sight (LOS) path exists, we propose a geolocation-based MIMO transmission scheme that determines the user’s precoder and modulation and coding scheme (MCS) without requiring the conventional feedback procedures. To capture the correlation, we utilize deep reinforcement learning (DRL) to perform the task of mapping from geolocation to transmission parameters. Based on the principles of Type I codebook, we further decompose the task into subtasks to reduce the action space. The extensive simulations on realistic ray-tracing channels demonstrate comparable performance to CSI feedback-based methods in 5G under ideal (zero feedback delay) conditions and better performance against 5G (with feedback delay) and DRL without task decomposition.
更多查看译文
关键词
MIMO,precoding,codebook,deep reinforcement learning,FD-RAN
AI 理解论文
溯源树
样例
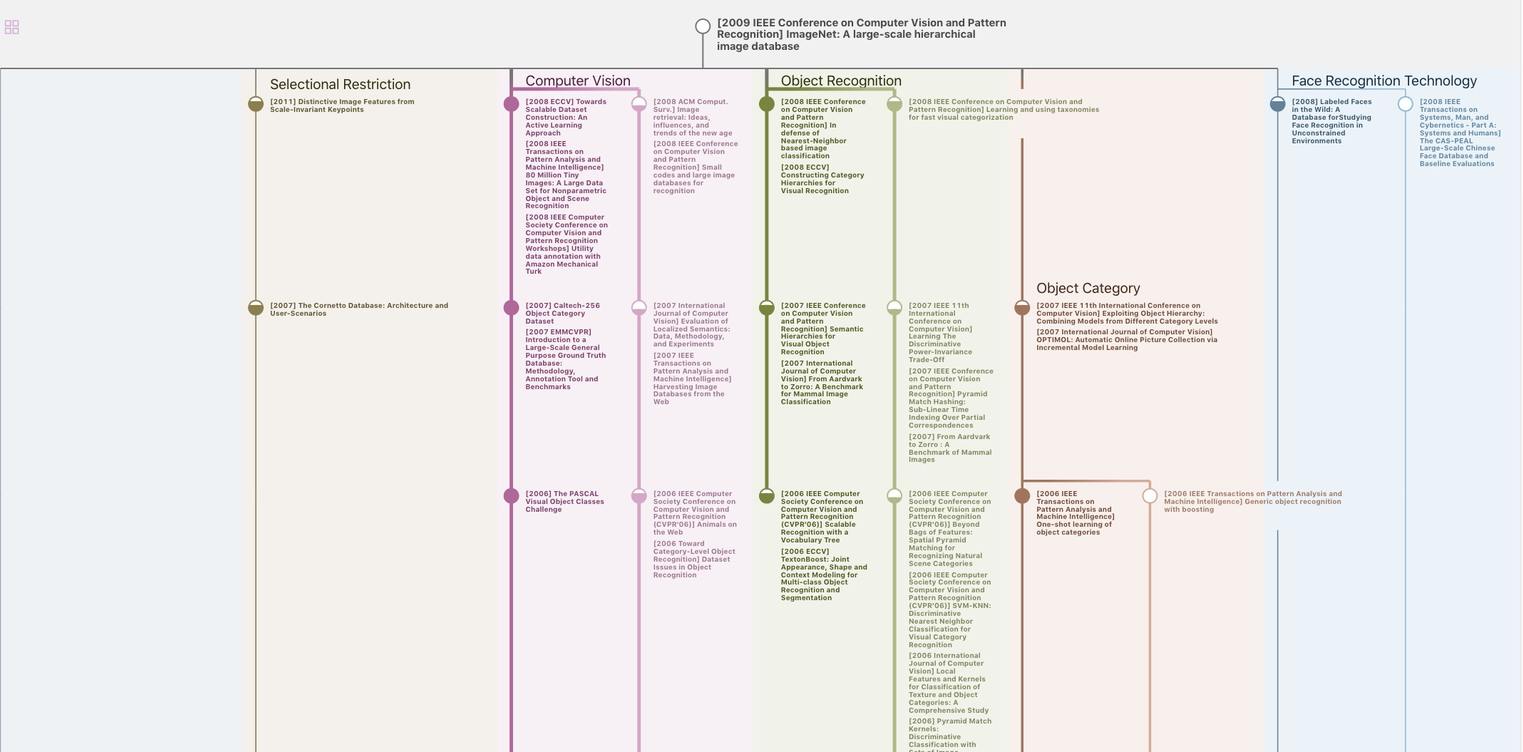
生成溯源树,研究论文发展脉络
Chat Paper
正在生成论文摘要