Machine Learning Approaches for Cell Viability
SIU(2023)
摘要
Cell viability is important for clinical studies such as stem cell treatments, cancer treatments, aesthetics, and cosmetics. In order to apply the right treatment and approach, the total cell viability rate in the sample should be known. At this point, it is critical to correctly classify the cells in the sample as live or dead. This study aims to classify cells as dead or live by using machine learning algorithms. Within the scope of the study, the performances of artificial learning classifiers were compared using random forest, XGBoost, and LightGBM algorithms, which are ensemble learning methods. The experimental study used two different datasets including fibroblast cells and mesenchymal stem cells. For both datasets, algorithms were run with the best parameter values after hyper-parameter optimization for each algorithm. While the best accuracy value for fibroblast cells was obtained from the XGBoost algorithm with a value of 97.69%, the best accuracy value for mesenchymal stem cells was obtained from the LightGBM algorithm with a value of 92.42%.
更多查看译文
关键词
Cell viability,Random Forest,XGBoost,LightGBM
AI 理解论文
溯源树
样例
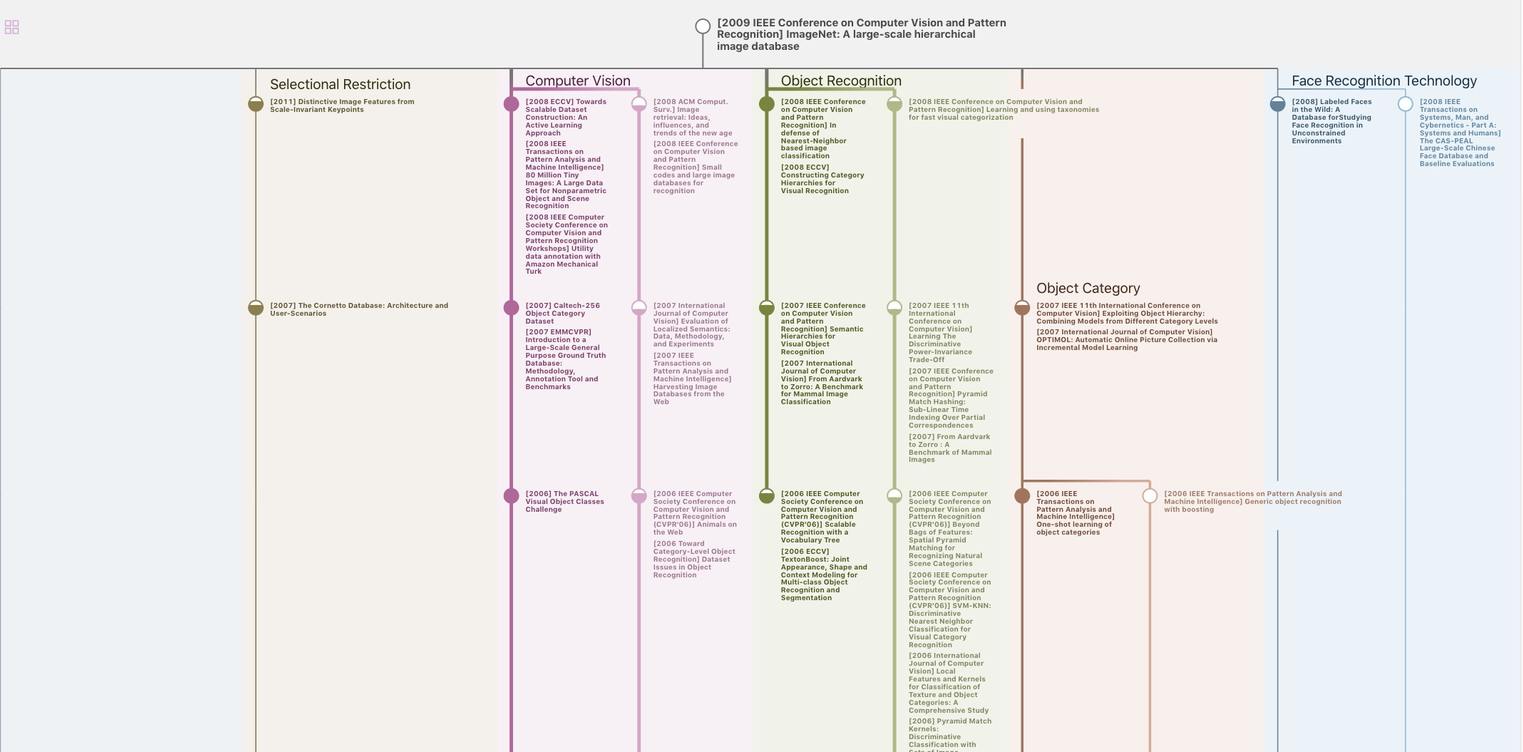
生成溯源树,研究论文发展脉络
Chat Paper
正在生成论文摘要