An Efficient Massive MIMO Detector Based on Deep Learning and Approximate Matrix Inversion Methods.
SmartNets(2023)
摘要
The use of massive multiple-input multiple-output (mMIMO) technology is essential for the fifth-generation (5G) and sixth-generation (6G) networks. However, the computational complexity of detection techniques and approximation methods can be high due to matrix inversion. Deep learning (DL) has been proposed as a tool to improve the efficiency of massive MIMO systems. This study proposes a hybrid-based low-complexity detector employing deep learning and approximate matrix inversion. The Richardson method and multi-scale multi-skip connection network (MMNet) form the presented hybrid detection framework. The output of the first iteration of approximate matrix inversion methods is fed into the MMNet algorithm in order to obtain superior performance. The results are compared with the MMSE-based and conventional MMNet-based detectors to determine/benchmark the performance. The simulation results and benchmarks with an MMSE-based and conventional MMNet-based detectors further designate that employing the proposed model significantly enhances the detection performance.
更多查看译文
关键词
5G,6G,deep learning,detection,mMIMO,Richardson iteration
AI 理解论文
溯源树
样例
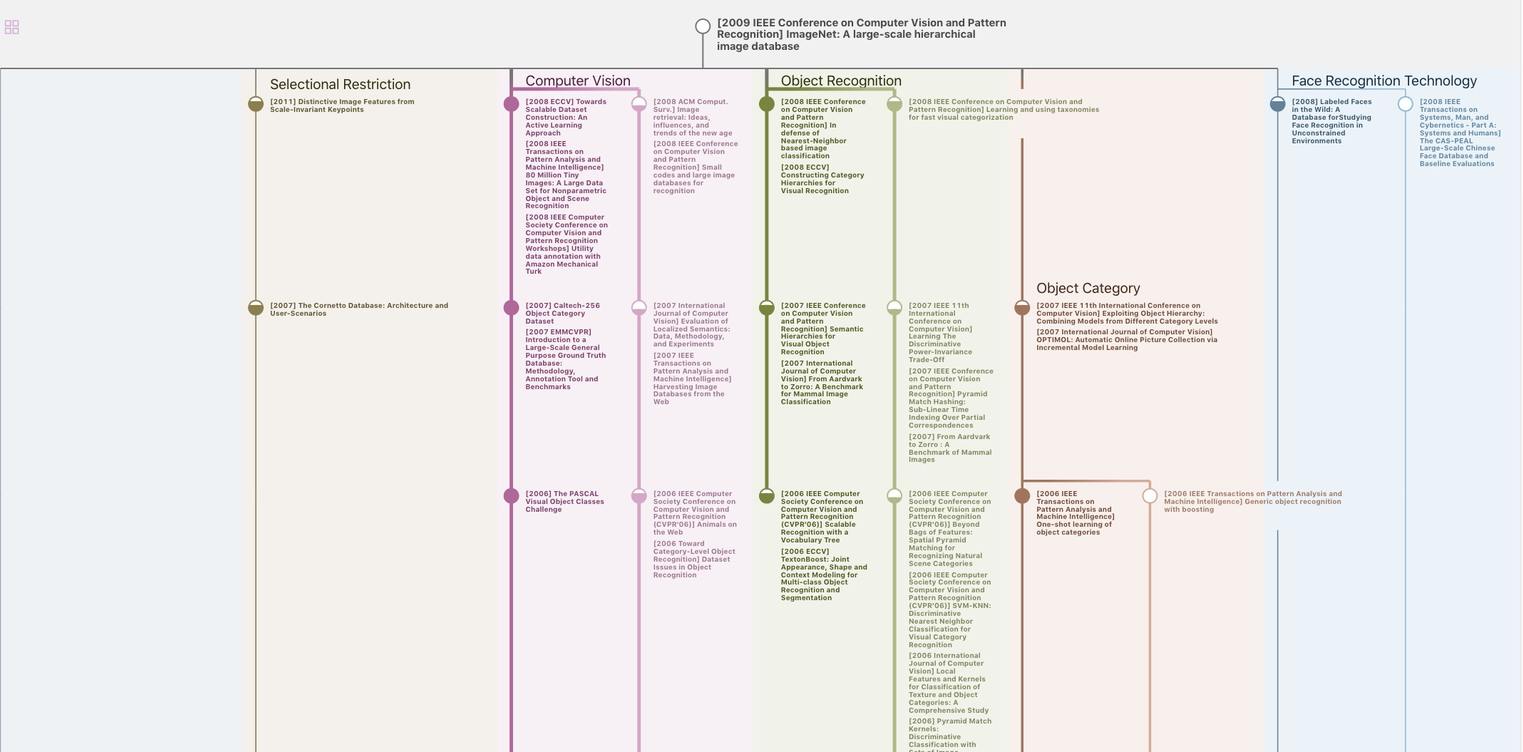
生成溯源树,研究论文发展脉络
Chat Paper
正在生成论文摘要