On-FPGA Spiking Neural Networks for Multi-variable End-to-End Neural Decoding
APPLIED RECONFIGURABLE COMPUTING. ARCHITECTURES, TOOLS, AND APPLICATIONS, ARC 2023(2023)
摘要
In the field of brain-machine interface (BMI), deep learning algorithms have been steadily advancing as the go-to instrument for the key task of neural decoding. However, to function in real-time on portable devices, these algorithms must adhere to stringent limitations on computational power and memory. In this work, we exploit spiking neural networks (SNNs) within a real-time neural decoding system deployed on a low-end Artix-7 FPGA. The system is capable of decoding the spike activity in intracortical neural signals, recorded by a 96-channels microelectrode array, to continuously and concurrently track five target variables in a reach-to-grasp experiment. We have assessed our approach on a widely used reference dataset, achieving a decoding accuracy comparable with alternatives in literature, which exploit more complex deep learning models on the same dataset to decode a single target variables. Our system uses around 20 times less parameters than other non-SNN approaches and consumes 56.4 mW.
更多查看译文
关键词
Neural decoding,SNN,FPGA,Low power
AI 理解论文
溯源树
样例
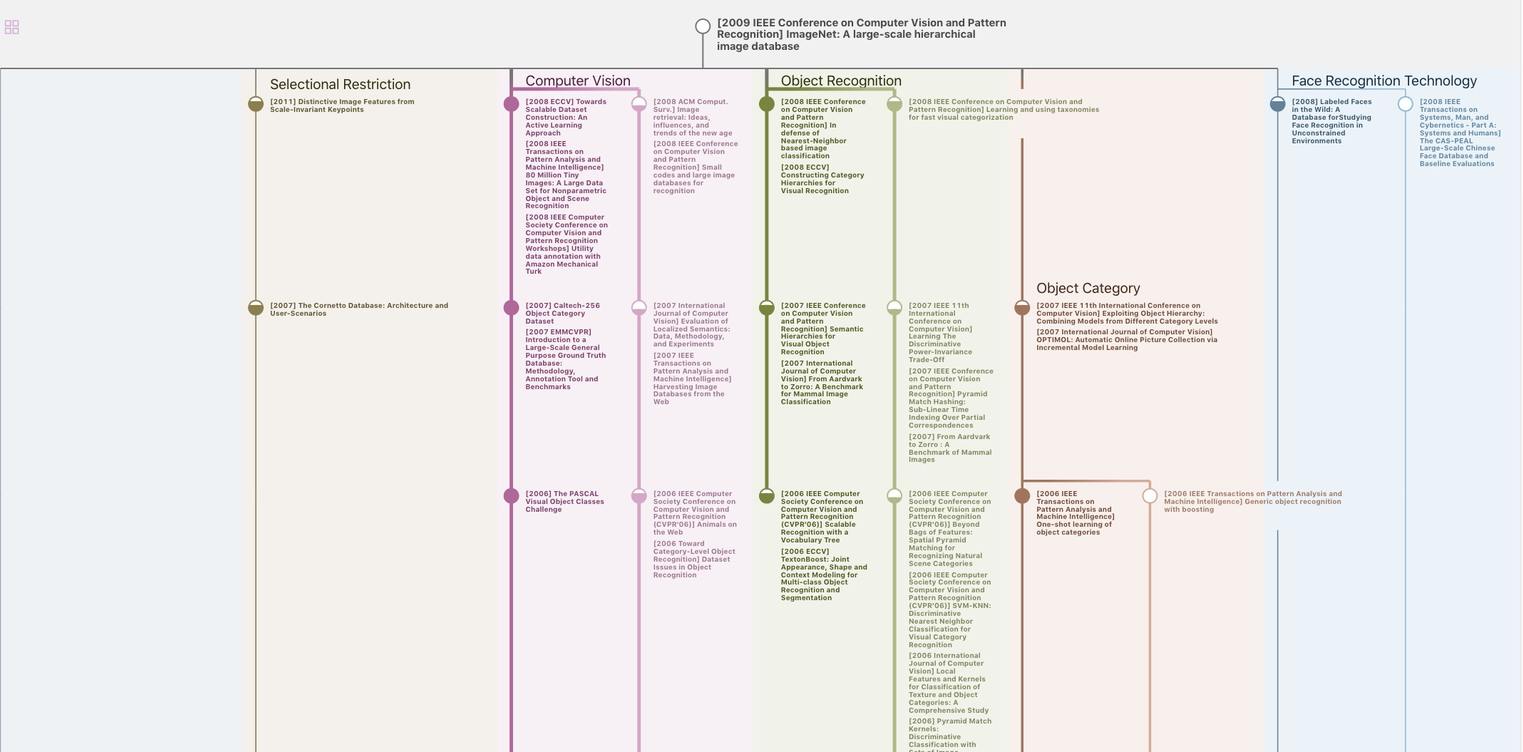
生成溯源树,研究论文发展脉络
Chat Paper
正在生成论文摘要