Multiple Object Tracking Based on Variable GIoU-Embedding Matrix and Kalman Filter Compensation
ARTIFICIAL NEURAL NETWORKS AND MACHINE LEARNING, ICANN 2023, PT IX(2023)
摘要
Despite tracking-by-detection having shown dramatically rapid improvement, most existing approaches are still scrabbling in dense pedestrian tracking. To address this problem, this work presents a new multiple object tracking approach, named VacoTrack. This method combines variable GIoU-Embedding matrix (VGE) and Kalman Filter compensation, which introduces motion compensation operation over trajectory parameters to construct virtual uniform linear trajectories for objects. This method combines GIoU distance and Embedding cosine distance of objects variably as a new association matrix VGE to adjustably calculate similarity matrix in facing different occlusion problem. After association, this method sends all tracked trajectories back to Kalman Filter, including constructed virtual trajectories for re-matched objects, and then operates Kalman Filter compensation to fine tune trajectory parameters. Thus, this approach regards the motion patterns of objects as uniform linear motion patterns to identify them across dense pedestrian, and improve robustness. Our proposed approach achieves 64.43 and 63.14 HOTA on MOT17 and MOT20 benchmarks respectively and outperforms state-of-the-art in most evaluation metrics.
更多查看译文
关键词
Multiple Object Tracking,Variable GIoU-Embedding Matrix,Kalman Filter Compensation,Dense Pedestrian Tracking,Uniform Linear Motion Pattern
AI 理解论文
溯源树
样例
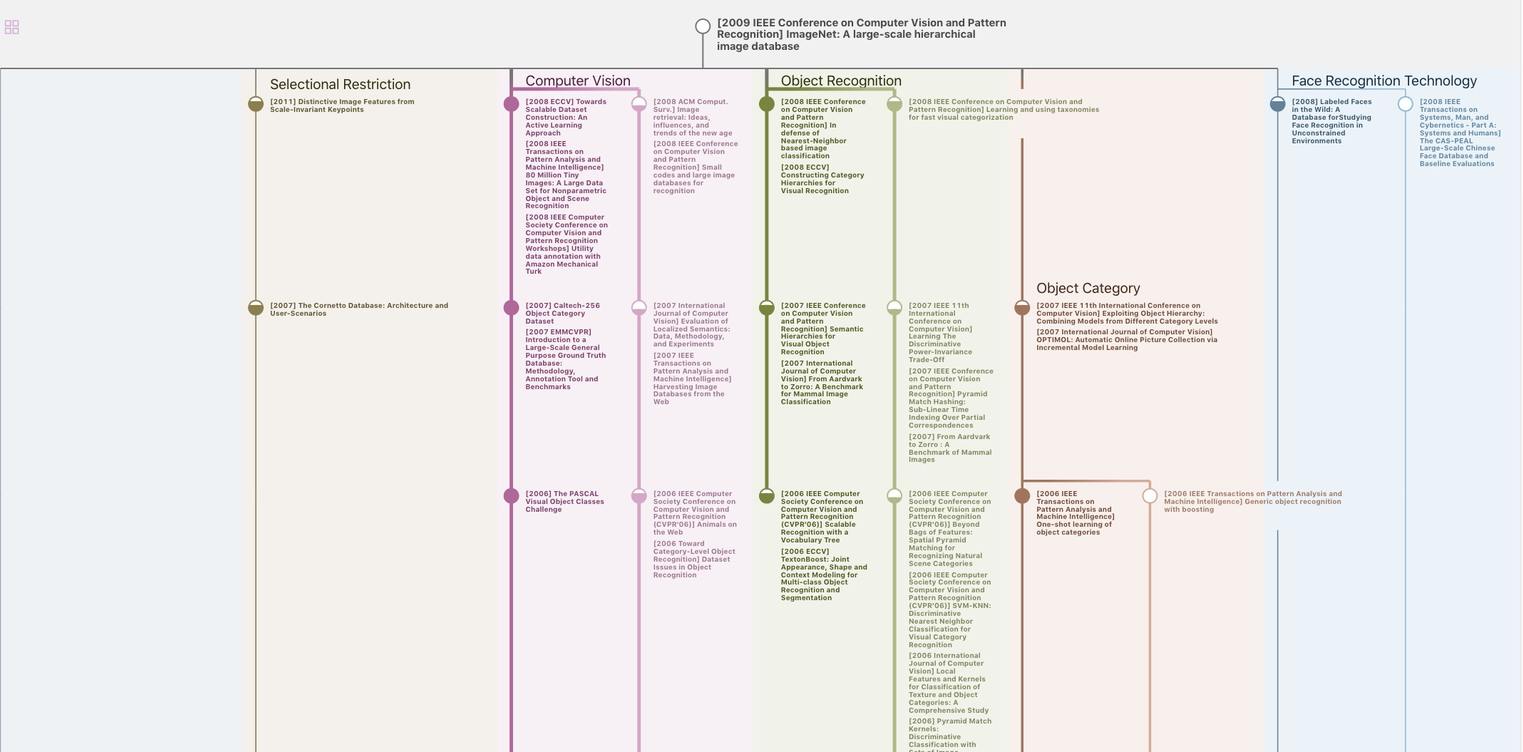
生成溯源树,研究论文发展脉络
Chat Paper
正在生成论文摘要