Semilayer-Wise Partial Quantization Without Accuracy Degradation or Back Propagation
ARTIFICIAL NEURAL NETWORKS AND MACHINE LEARNING, ICANN 2023, PT IX(2023)
摘要
In edge AI technologies, reducing memory bandwidth and computational complexity without reducing inference accuracy is a key challenge. To address this difficulty, partial quantization has been proposed to reduce the number of bits in weight parameters of neural network models. However, existing techniques monotonically degrade accuracy with the compression ratio without retraining. In this paper, we propose an algorithm for semilayer-wise partial quantization without accuracy degradation or back-propagation retraining. Each layer is divided into two channel groups (semilayers): one being positive for loss degradation and the other negative. Each channel is classified as positive or negative in terms of cross-entropy loss and assigned to a semilayer accordingly. The evaluation was performed with validation data as input. Then, the quantization priority for every semilayer is determined based on the magnitude in the Kullback-Leibler divergence of the softmax output before and after quantization. We observed that ResNet models achieved no degradation in accuracy at certain parameter compression ratios (i.e., 79.43%, 78.01%, and 81.13% for ResNet-18, ResNet-34, and ResNet-50, respectively) in partial 6-bit quantization on classification tasks using the ImageNet dataset.
更多查看译文
关键词
Partial Quantization,Sensitivity Analysis,Image Classification
AI 理解论文
溯源树
样例
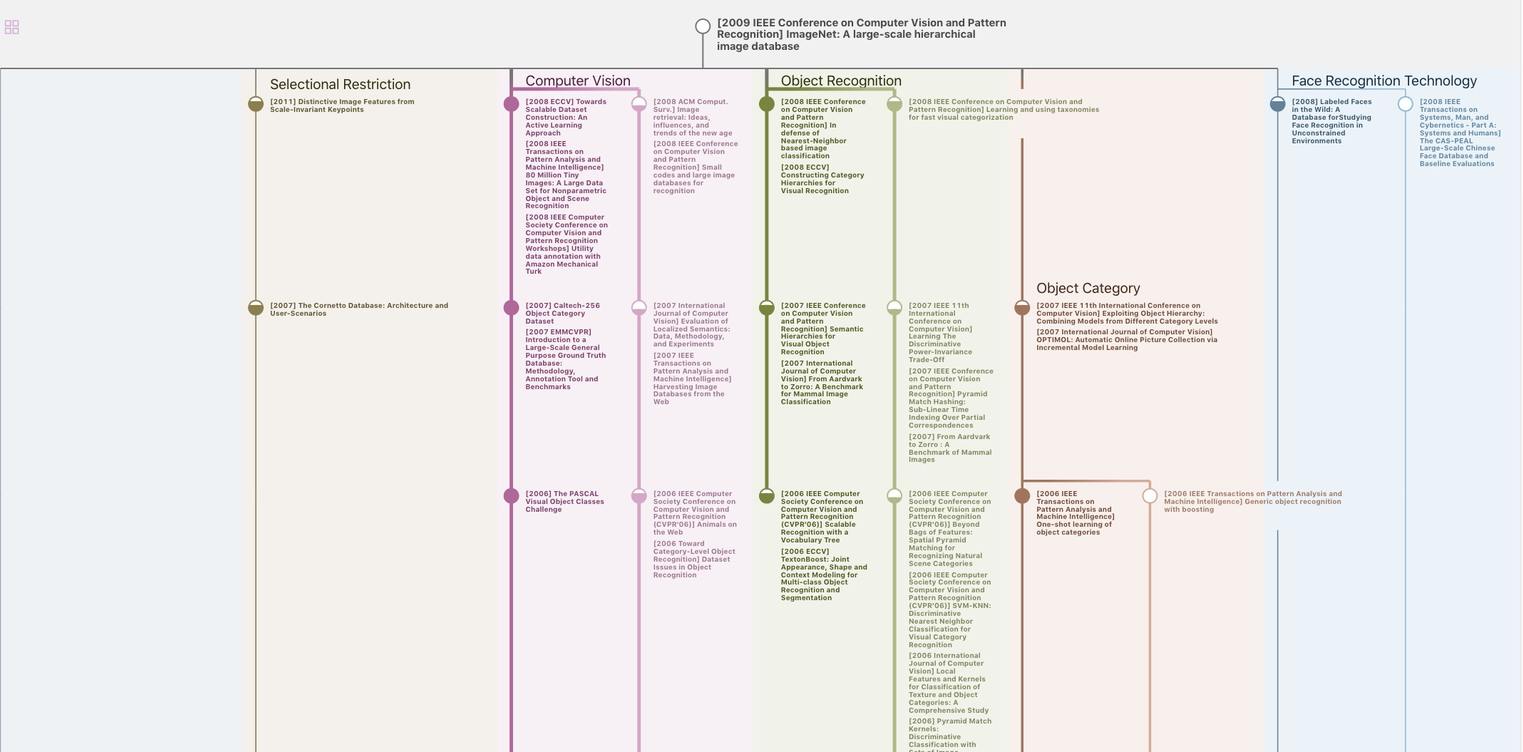
生成溯源树,研究论文发展脉络
Chat Paper
正在生成论文摘要