Incremental Recommendation Algorithm Based on the Influence Propagation Model
ARTIFICIAL NEURAL NETWORKS AND MACHINE LEARNING, ICANN 2023, PT VII(2023)
摘要
Recently, Graph based recommendation algorithms have gained more and more attention due to their flexible and unified embedding representation of both users and items as well as the effective modeling of context information for efficient recommendation. However, most of the existing graph recommendation methods are designed upon the static interaction graph, while neglecting the dynamic evolution of the graph. This paper proposes a lightweight Influence Propagation Model, namely IPM, for efficient recommendation on the dynamic evolving graphs. Specifically, IPM models the accumulating and propagating procedure of influence on the user-item interaction graph to obtain the characteristics of users and items. For dynamic changed edges of the graph, the amount of information about the relevant users and items can be quickly updated by propagating the impact associated with the added interactions. Our model exhibits very efficient performance and comparable recommendation results with the same experimental setup compared to advanced graph recommendation algorithms and dynamic graph embedding algorithms.
更多查看译文
关键词
Recommendation Algorithm,Incremental Algorithm,Graph Machine Learning,Influence Propagation Procedure
AI 理解论文
溯源树
样例
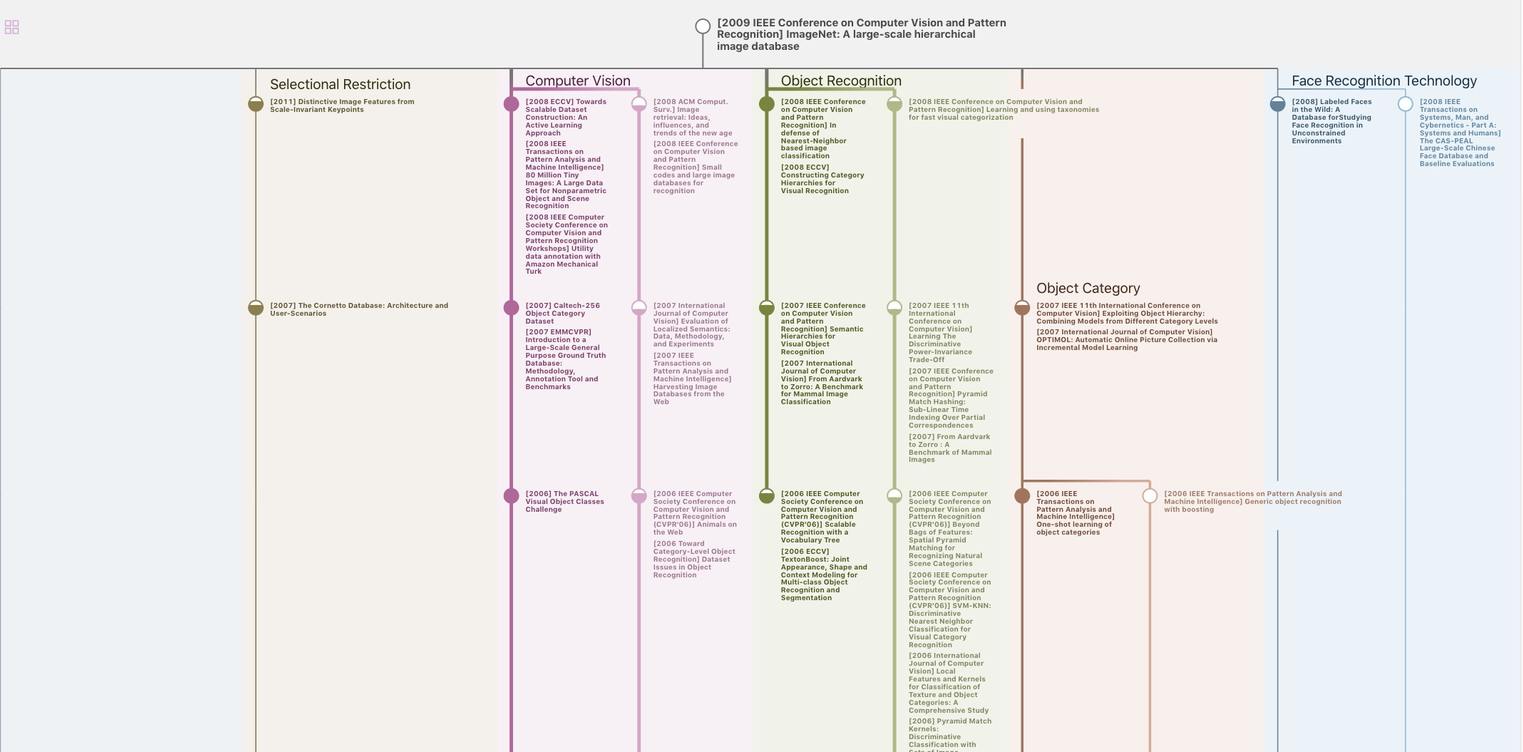
生成溯源树,研究论文发展脉络
Chat Paper
正在生成论文摘要