Sparse Representation based Deep Residual Geometry Compression Network for Large-scale Point Clouds
ICME(2023)
摘要
The increasing applications of 3D point clouds require efficient compression techniques to achieve high-quality and low-delay services. However, the computational efficiency and rate-distortion performance for large-scale dense point clouds are still challenging, and the phenomenon of reconstruction ability degradation also exists when the network is deep. To solve these challenges, we propose a novel fully end-to-end point cloud compression model based on sparse convolution. Specifically, we adopt a long-range-residual aided architecture to avoid the reconstruction degradation and high computational complexity of deep networks. Further, we propose a multi-scale geometry compression module to construct an end-to-end network that avoids the accumulation of reconstruction distortion during decoding. Experiments on the large-scale Moving Picture Experts Group (MPEG) PCC benchmarks show that our model outperforms the latest Video-based Point Cloud Compression (VPCC) scheme in terms of lossy geometry compression by 50.4% in D1 BD-rate and 50.8% in D2 BD-rate, while maintaining affordable processing speed and memory consumption.
更多查看译文
关键词
Point cloud compression,sparse representation,geometry compression
AI 理解论文
溯源树
样例
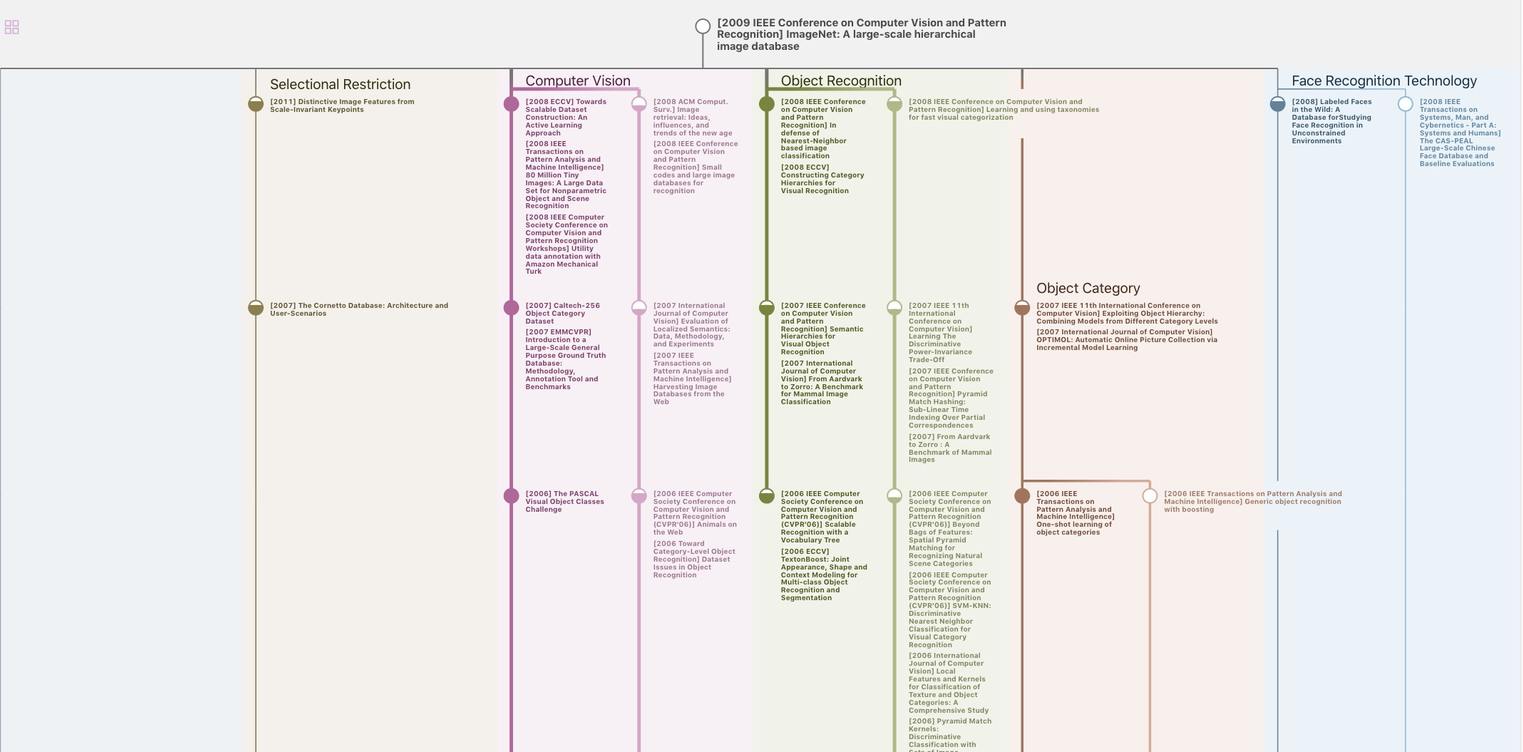
生成溯源树,研究论文发展脉络
Chat Paper
正在生成论文摘要