Weakly Supervised Few-Shot and Zero-Shot Semantic Segmentation with Mean Instance Aware Prompt Learning.
2023 IEEE International Conference on Multimedia and Expo (ICME)(2023)
摘要
The existing Zero-Shot Segmentation (ZSS) and Few-Shot Segmentation (FSS) methods utilize fully supervised pixel-labeled seen classes to segment unseen classes. Pixel-level labels are hard to obtain, and using weak supervision in the form of inexpensive image labels is often more practical. To this end, we propose a novel unified weakly supervised Zero-Shot and Few-Shot semantic segmentation pipeline that can perform ZSS and FSS on novel classes without using pixel-level labels for either the base (seen) or the novel (unseen) classes. We propose Mean Instance Aware Prompt based Network (MIAPNet), a novel language-guided segmentation pipeline that i) learns context vectors with batch aggregates (mean) to map class prompts to image features and ii) decouples weak ZSS/FSS into weak semantic segmentation and Zero-Shot segmentation. MIAPNet beats existing methods for weak generalized ZSS and weak FSS by 39 and 3 mIOU points respectively on PASCAL VOC and weak FSS by 5 mIOU points on MS COCO.
更多查看译文
关键词
Zero-Shot Segmentation,Few-Shot Segmentation,Language-Vision Models,Prompt Learning,Weakly Supervised Segmentation
AI 理解论文
溯源树
样例
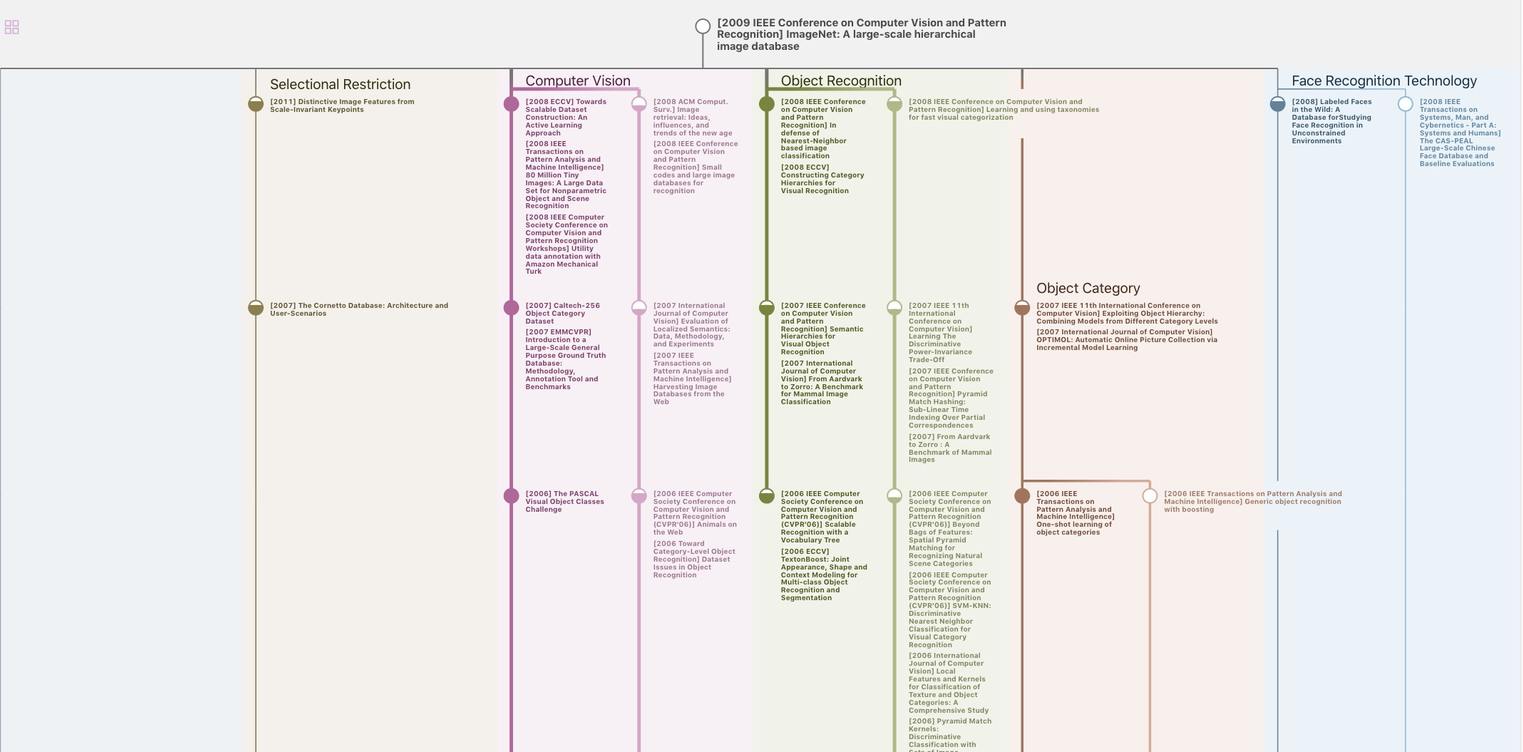
生成溯源树,研究论文发展脉络
Chat Paper
正在生成论文摘要