DSP-Net: Diverse Structure Prior Network for Image Inpainting.
ICME(2023)
摘要
The latest deep learning-based approaches have advanced diverse image inpainting task. However, existing methods limit to be aware of the structure information well, which constricts the performance of diverse generations. The intuitive representation of diversity generation is the structure change since the structure is the basis of the image. In this paper, we make full use of the structure information and propose the diverse structure prior network (DSP-Net). Specifically, there are two stages in DSP-Net to generate the diverse structure first and refine the texture next. For the diverse structure generation, we prompt the structural distribution to be similar to the Gaussian distribution to sample the diverse structural prior. With these priors, we refine the texture with a proposed propagation attention module. Meanwhile, we propose a structure diversity loss to enhance the ability of diverse structure generation further. Experiments on benchmark datasets including CelebA-HQ and Places2 indicate that DSP-Net is effective for diverse and visually realistic image restoration.
更多查看译文
关键词
Diverse Image Inpainting, Structure Priors
AI 理解论文
溯源树
样例
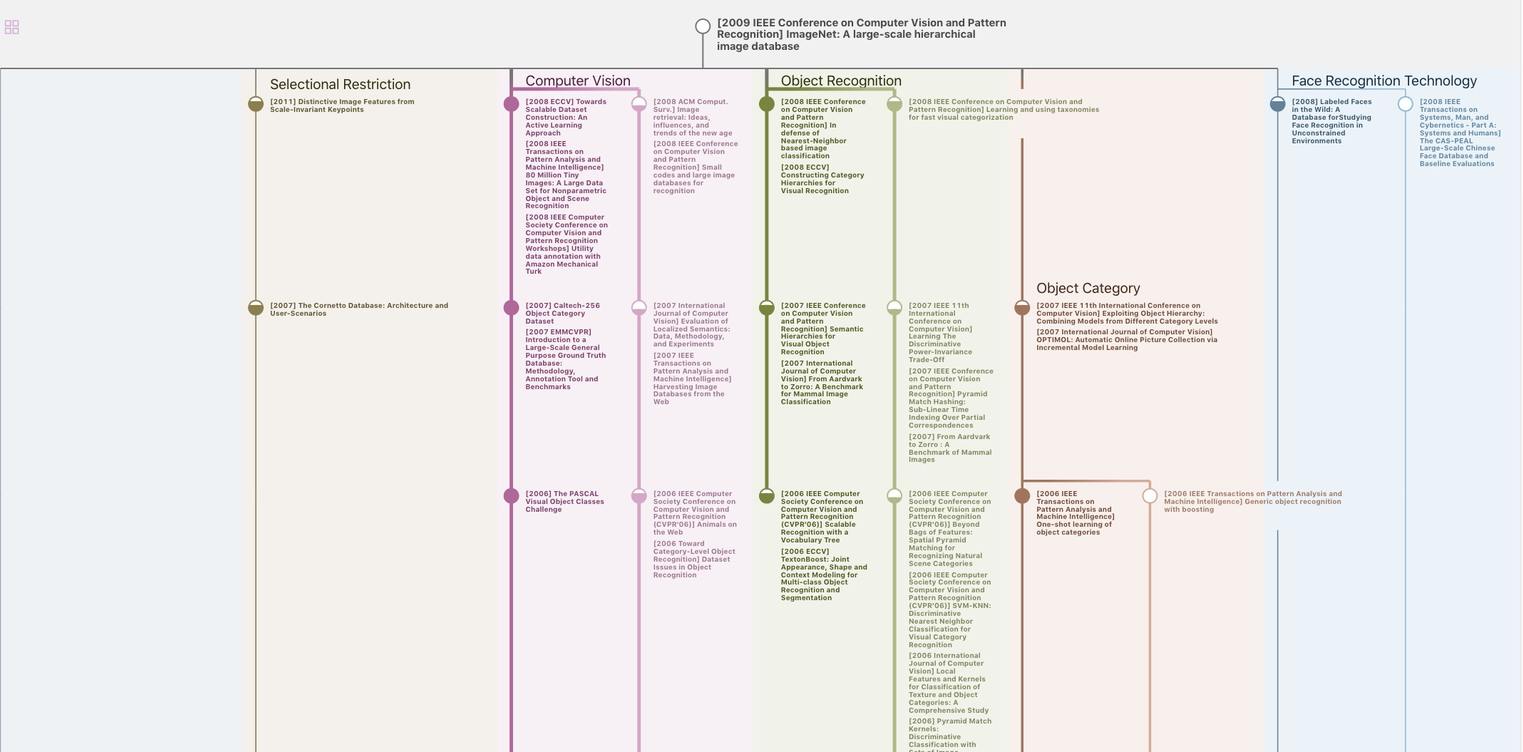
生成溯源树,研究论文发展脉络
Chat Paper
正在生成论文摘要