Constrained Gaussian-Process State-Space Models for Online Magnetic-Field Estimation.
FUSION(2023)
摘要
We address the magnetic-field simulteanous localization and mapping (SLAM) problem for global positioning. We leverage a previously-developed particle filter (PF)-based framework for online Bayesian inference and learning of Gaussian Process state-space models (GP-SSMs). We extend the framework to directly incorporate physical properties of the magnetic field in the GP formulation, by leveraging that magnetic fields under the absence of free currents are curl free. Because of its flexibility, the method can include any motion model that can be expressed by a general nonlinear function, with potential applications to, e.g., mobile robotics and pedestrian localization. Simulation results indicate that our method performs similar to recent batch methods for magnetic-field slam, while the computation times are feasible for online implementations.
更多查看译文
AI 理解论文
溯源树
样例
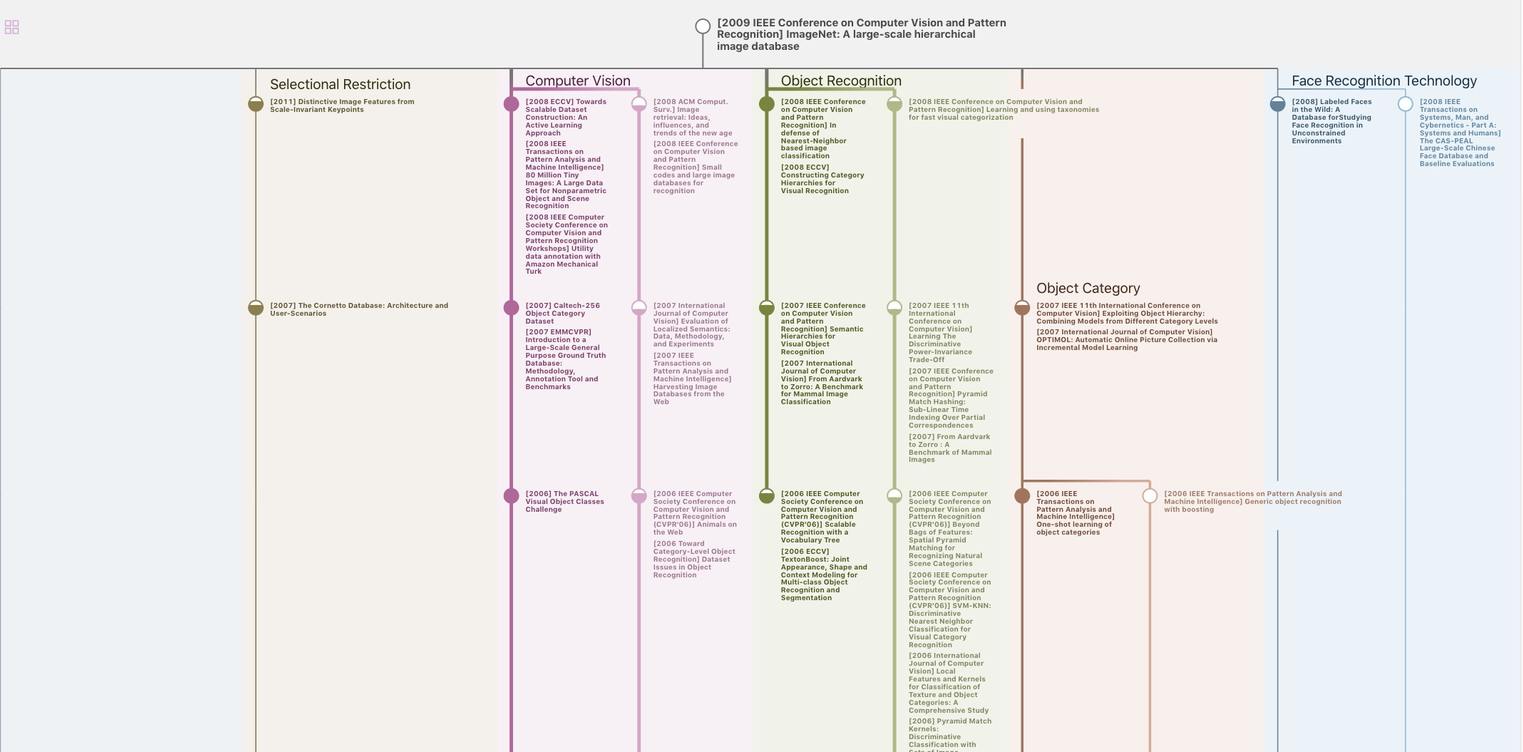
生成溯源树,研究论文发展脉络
Chat Paper
正在生成论文摘要