Robust Subgraph Augmentation for Graph Convolutional Networks with Few Labeled Nodes
ICARM(2023)
摘要
Graph Neural Networks (GNNs) have garnered significant attention owing to their remarkable capacity to extract representations from non-Euclidean data. Despite their success, the performance of prevalent GNNs degrades significantly when structural noise is introduced into the graph data. Besides, the reliance of GNNs on a considerable volume of labeled nodes presents challenges in dealing with the low-data regime where labeled nodes are scarce. This paper aims to address a challenging scenario in which structural noise and the low-data regime occur simultaneously. Specifically, we exploit the valuable information from the local topologies, i.e. subgraphs, of a graph as the augmented supervision signals for the extensive amounts of unlabeled nodes. Moreover, to alleviate the effects of structural noise, we remove potential noisy edges linking dissimilar nodes, generating robust subgraphs for the downstream node classification tasks. We conduct a series of experimental studies on canonical node classification tasks using three graph datasets. The results of our experimental analyses demonstrate that our approach can achieve state-of-the-art performance under structural noises and the low-data regime.
更多查看译文
关键词
Graph Neural Networks,Robust Machine Learning,Subgraph,Node Classification
AI 理解论文
溯源树
样例
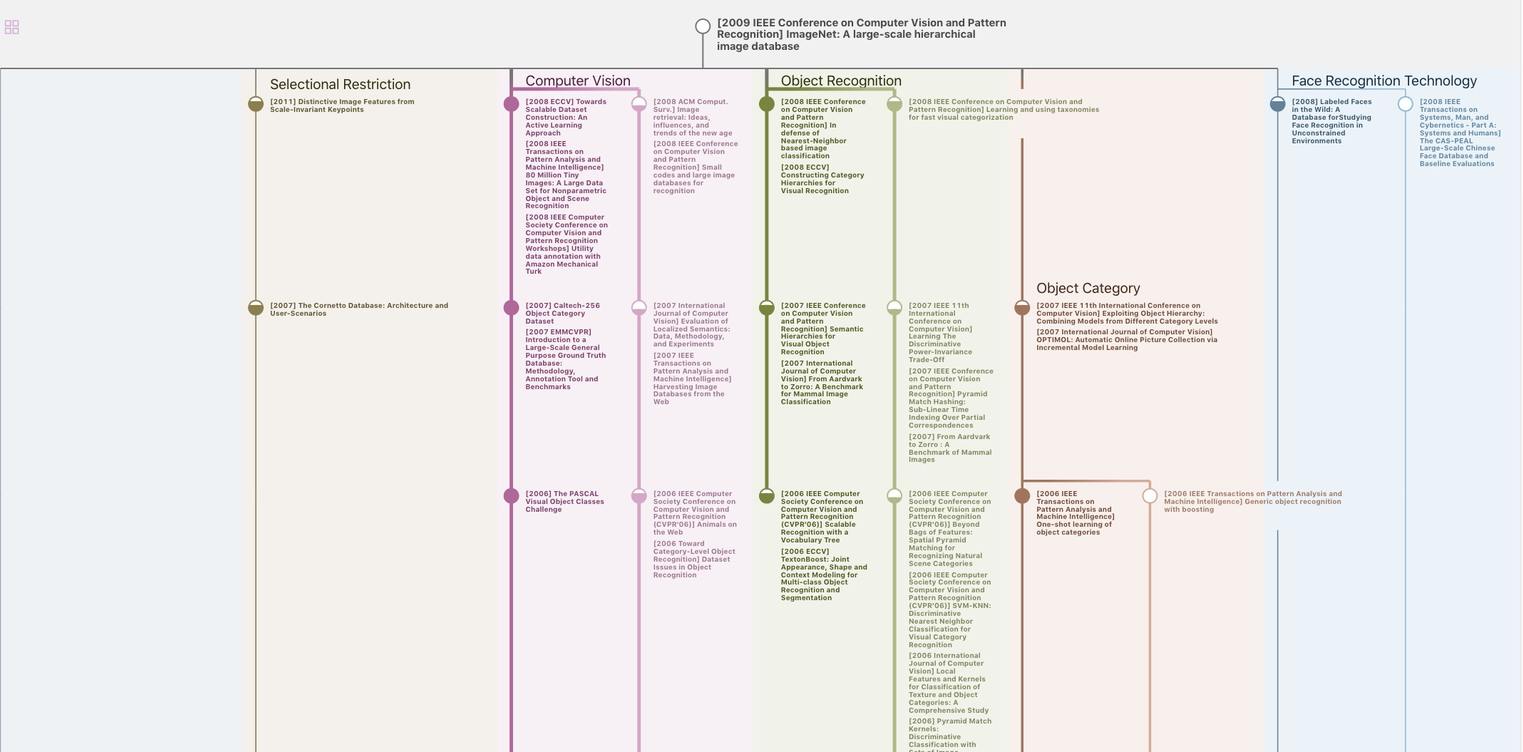
生成溯源树,研究论文发展脉络
Chat Paper
正在生成论文摘要