Learning Representations for MR Image Retrieval: Contrastive Models Versus Other Supervision Strategies.
ISBI(2023)
摘要
Content-Based Image Retrieval (CBIR) is an image search technique which consists of retrieving from a database the most similar images to a query image, based on their representations. In this paper, we propose to learn such representations in the context of MRI using a convolutional neural network trained in a self-supervised contrastive fashion, which requires a lower degree of supervision than state-of-the-art supervised approaches. Embedded in a CBIR pipeline for medical images, we apply this method to the BRaTS MR dataset. We also present an approach to evaluate the retrieval results using the annotations already available in the dataset. To capture the complementarity across different MR sequences, we investigate the benefit of information fusion in learning relevant representations. Our experiments show that a weakly supervised training may be advantageous not only in terms of requiring fewer annotations, but also because it may yield increased performance compared to its supervised counterparts.
更多查看译文
关键词
MRI,image retrieval,contrastive representation learning,weak supervision,sequence fusion
AI 理解论文
溯源树
样例
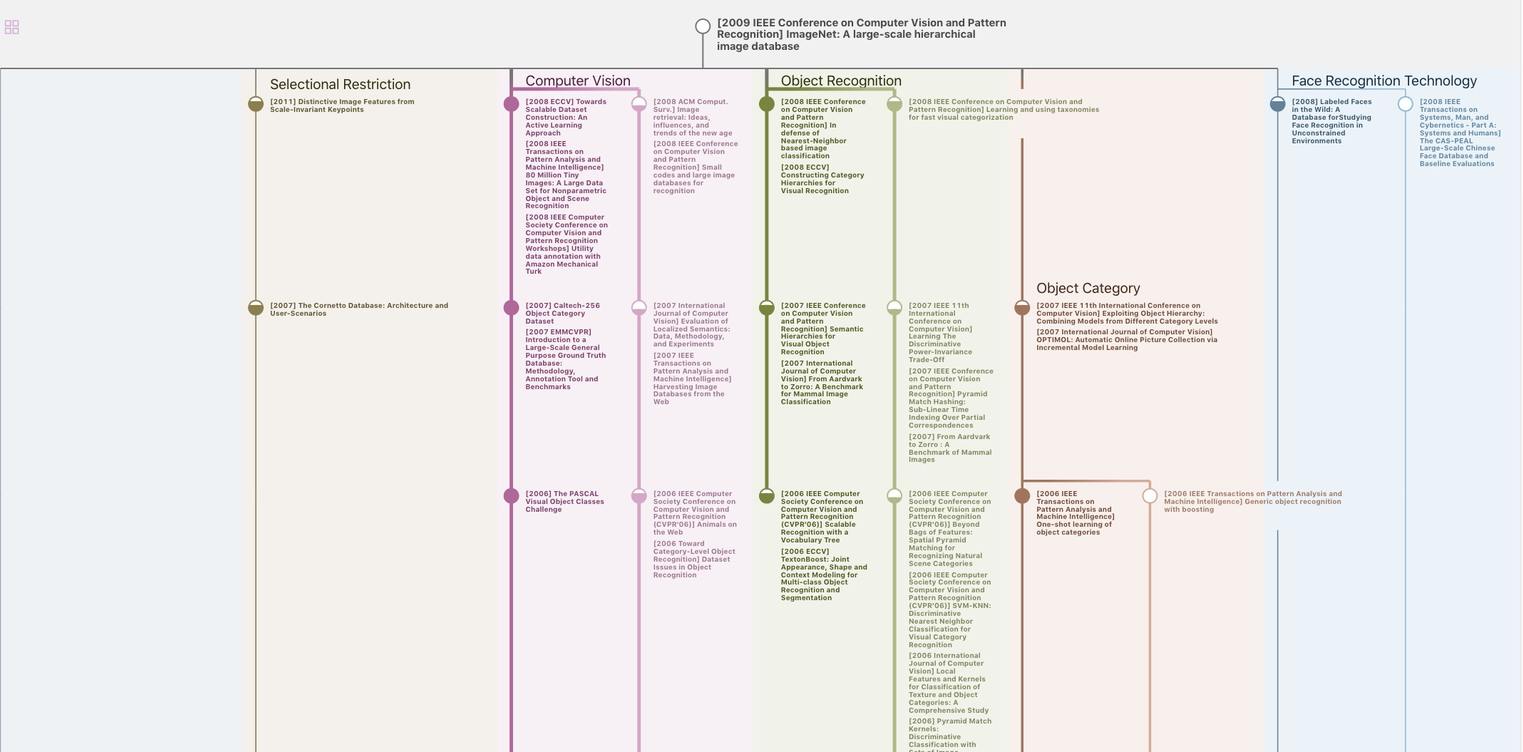
生成溯源树,研究论文发展脉络
Chat Paper
正在生成论文摘要