Fast and Interpretable Unsupervised Domain Adaptation for FIB-SEM Cell Segmentation.
ISBI(2023)
摘要
Electron microscopy imaging techniques allow biologists to obtain nanoscale slice volumes of cells in which biological structures can be statistically analyzed under the condition that the tedious task of segmentation can be automated. If a learned segmentation has good results on images obtained under the same acquisition process, variations in this acquisition can drastically affect the final image's features and can lead to a drop of segmentation performances. To tackle this problem, we propose an Unsupervised Domain Adaptation (UDA) framework based on Batch Normalization. Our method tested on mitochondria segmentation shows better results than state-of-the art methods and uses few computation resources, allowing a near real-time use. Furthermore, we propose a metric based on the Wasserstein distance to evaluate the effect of this normalization. We empirically show that this measure is log-linearly correlated with the drop of performances of the adapted segmentation and sheds light on the gain obtained by our UDA framework.
更多查看译文
关键词
Unsupervised domain adaptation, Cell mitochondria segmentation, FIB-SEM, quantifying domain shift
AI 理解论文
溯源树
样例
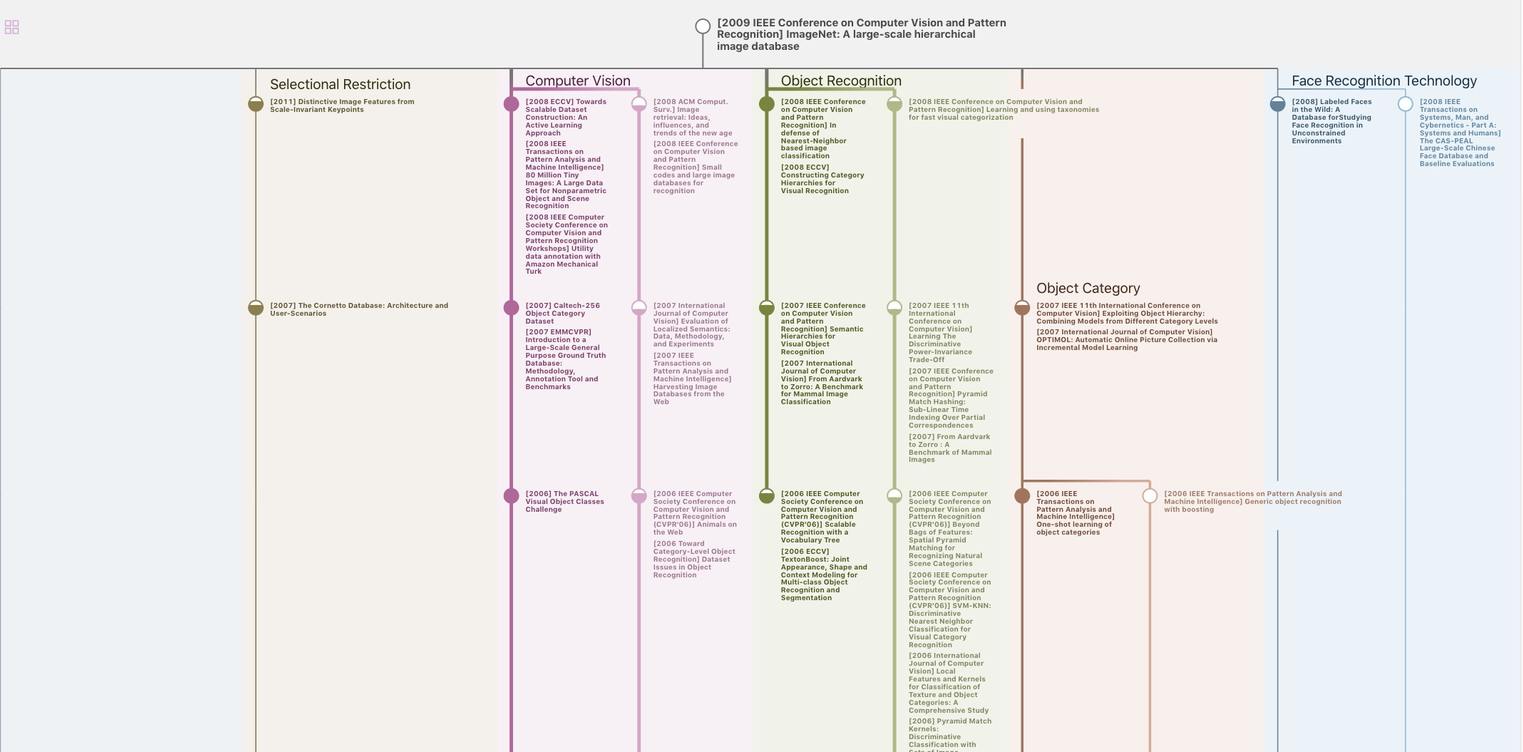
生成溯源树,研究论文发展脉络
Chat Paper
正在生成论文摘要