Evidence Dictionary Network Using Multiple Instance Contrastive Learning for Explainable Pathological Image Analysis.
ISBI(2023)
摘要
In this study, we aim to construct a pathological image diagnosis support system that can perform accurate diagnoses and enable users to easily interpret the basis for its decisions. To this end, we propose an explainable neural network that can provide the basis for its decisions from a dictionary constructed by the network itself. The network explains the basis by retrieving images from its dictionary similar to local regions in an input image. The network learns histological patterns such as pathological findings that contributed to the decisions and constructs its dictionary from the representative patterns via multiple instance learning (MIL) and contrastive learning. MIL enables the network to learn histological patterns without pixel-wise annotations, whereas contrastive learning makes the dictionary items consistent for interpretability. We perform pathological whole-slide image classification using a lung biopsy dataset, and the results show that interpretable dictionary items could be learned. Further, based on the results obtained, we establish that our method is more diagnostically accurate than the existing methods.
更多查看译文
关键词
Explainable AI,Pathological Image Analysis,Multiple Instance Learning,Deep Neural Networks,Computer-aided diagnosis
AI 理解论文
溯源树
样例
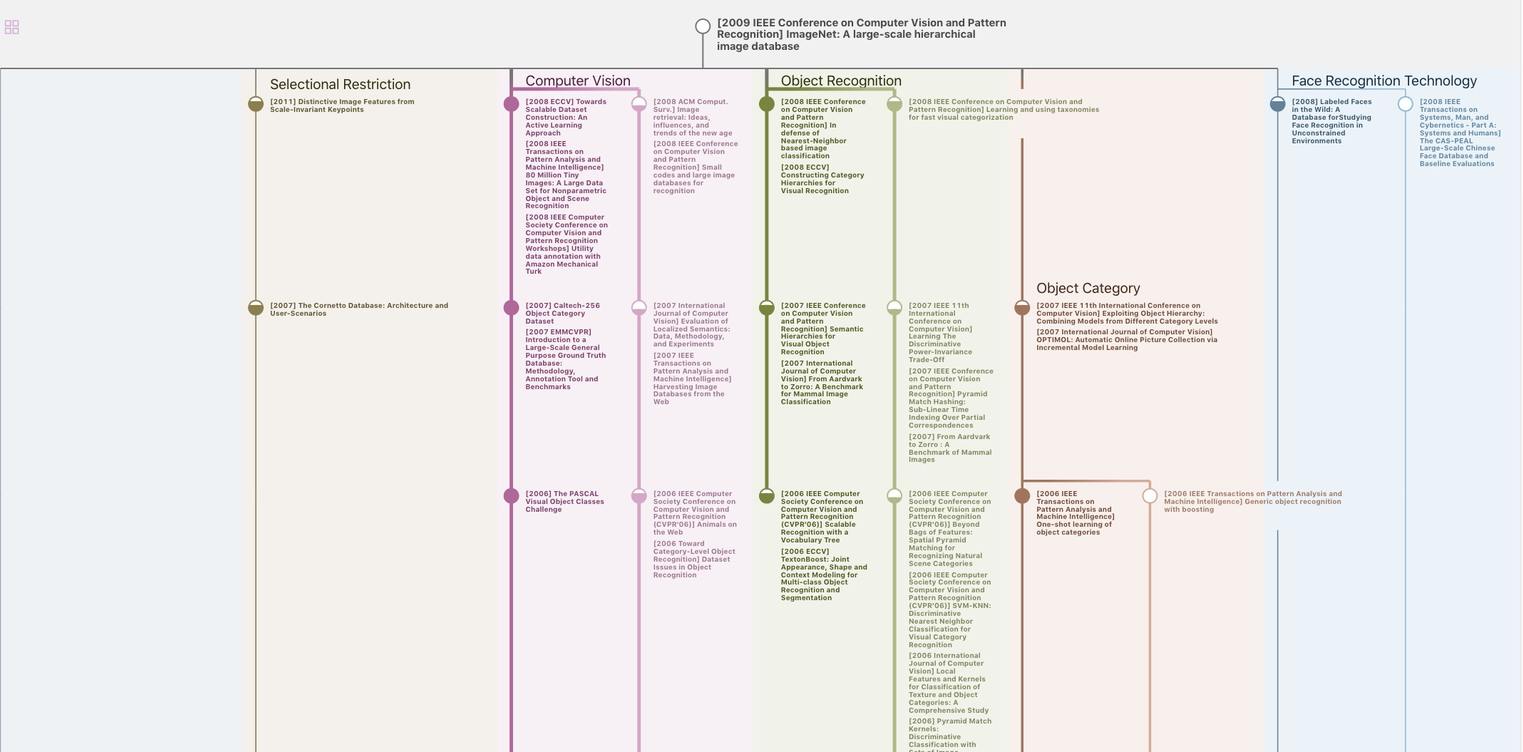
生成溯源树,研究论文发展脉络
Chat Paper
正在生成论文摘要