Iterative Data Refinement for Self-Supervised Learning MR Image Reconstruction.
ISBI(2023)
摘要
Magnetic Resonance Imaging (MRI) is an important technique in the clinic. Fast MRI based on k-space undersampling and high-quality image reconstruction has been widely utilized, and many deep learning-based methods have been developed in recent years. Although promising results have been achieved, most existing methods require fully-sampled reference data. Unfortunately, fully-sampled MRI data are difficult to obtain in real-world applications. To address this issue, we propose a data refinement framework for self-supervised MR image reconstruction. Specifically, we first analyze the reason of the performance gap between self-supervised and supervised methods and identify that the bias in the training datasets is one major factor. Then, we design an effective self-supervised data refinement learning framework to reduce this data bias. With the data refinement, an enhanced self-supervised MR image reconstruction method is developed to prompt fast MR imaging. We evaluate our method on an in-vivo MRI dataset. Experimental results show that without utilizing any fully sampled MRI data, our self-supervised learning framework possesses strong capabilities in capturing image details and structures at high acceleration factors.
更多查看译文
关键词
Deep Learning, MRI reconstruction, self-supervised learning, iterative data refinement
AI 理解论文
溯源树
样例
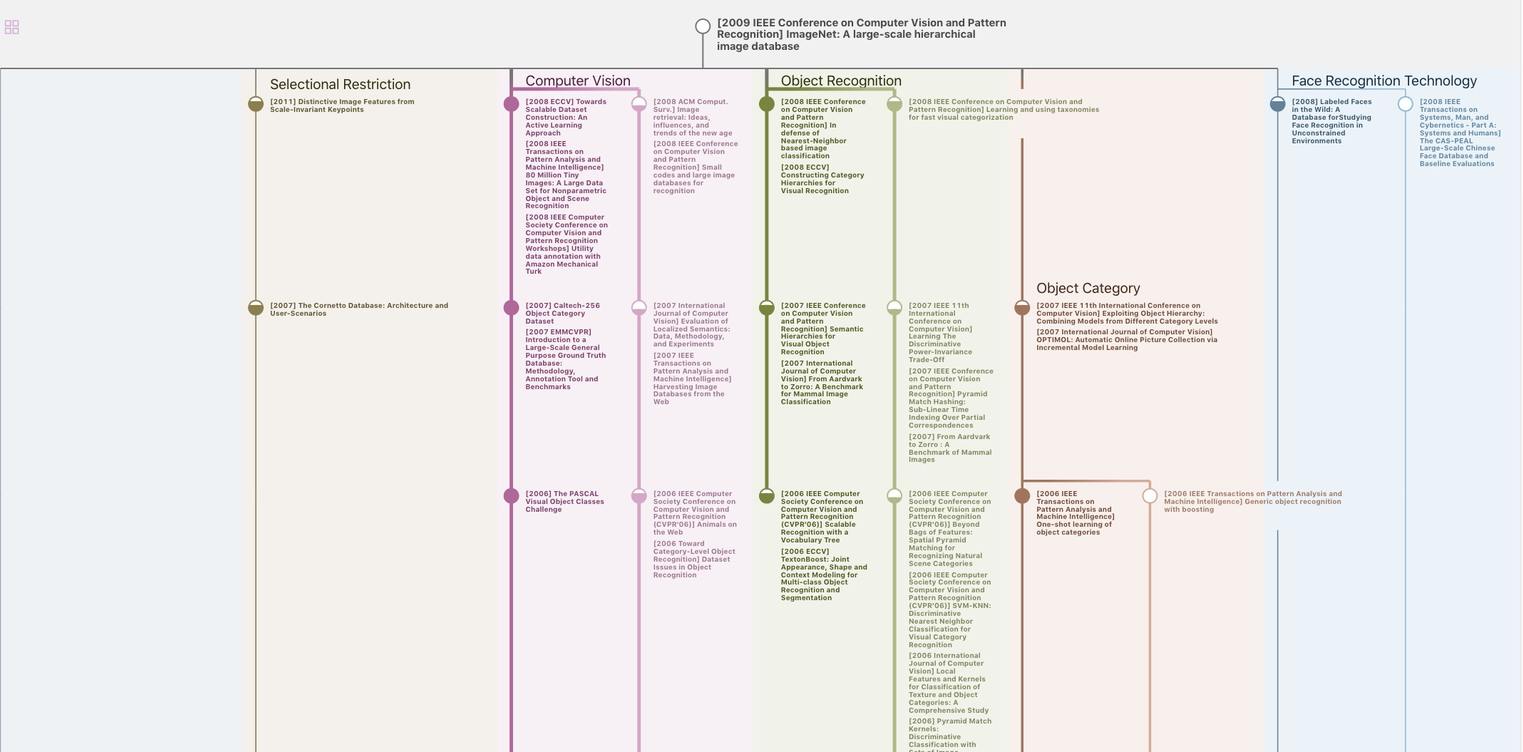
生成溯源树,研究论文发展脉络
Chat Paper
正在生成论文摘要