Attention in a Little Network is All You Need to Go Green
ISBI(2023)
摘要
The widely adopted practice to improve performance of deep neural networks is by increasing its depth and the number of parameters. Restraining their energy consumption is yet another community expectation. However, energy consumption is dependent on the number of operation and the memory involved during computation which increase with depth and number of parameters. It seems to be a conflicting goal to achieve high performance at low energy consumption. This phenomena can be observed with UNeXt which consumes significantly less energy on account of lesser number of parameters but fails to achieve adequate performance. In this paper, we crack the code by introducing attention mechanism within a network with lesser number of parameters in order to compensate its reduced information capacity, by fixating the attention to relevant regions per layer. The Energy efficient, Lightweight, and computationally Thin Network (ELiTNet) is thus proposed for semantic segmentation tasks, and demonstrated for semantic segmentation of retinal arteries and optic disc in digital color fundus images. Experiments compare it against SUMNet, U-Net, UNeXt and ResUNet++ architectures on five publicly available datasets including HRF, DRIVE, AMD, IDRiD, and REFUGE. We demonstrate that the proposed method consumes 2.2× lower energy, while featuring 155.2× fewer parameters, and 41.22× lesser GFlop in comparison with U-Net while maintaining an F1-score of 97.22 % and Jaccard Index of 94.74 % on IDRiD dataset. Source codes are available at Github
1
.
更多查看译文
关键词
Attention,optic disc,retinal vessels,semantic segmentation
AI 理解论文
溯源树
样例
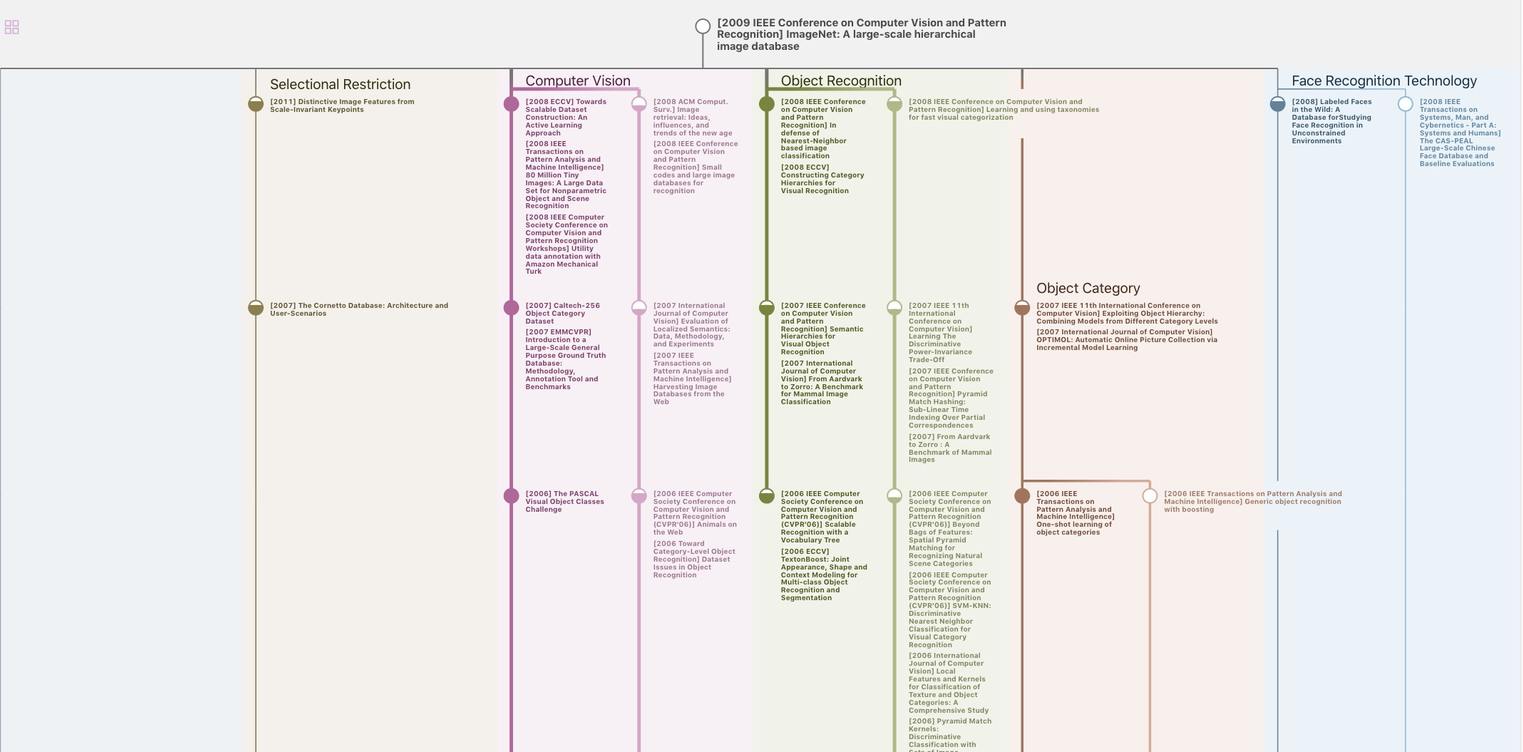
生成溯源树,研究论文发展脉络
Chat Paper
正在生成论文摘要