Transformer-Based Multimodal Fusion for Survival Prediction by Integrating Whole Slide Images, Clinical, and Genomic Data.
2023 IEEE 20TH INTERNATIONAL SYMPOSIUM ON BIOMEDICAL IMAGING, ISBI(2023)
Renmin Univ China | Univ Hong Kong
Abstract
Survival prediction using whole slide images (WSIs) is a complex and difficult task, as handling gigapixel WSI directly is computationally impossible. In the past few years, people have worked out multiple instance learning (MIL) strategies to deal with WSIs, i.e., splitting WSI into many patches (instances) and aggregating features across patches. Moreover, to better predict the survival outcome of patients, different modalities have been explored, among which gene features are used the most frequently. In this paper, we explore a graph-based strategy to handle WSIs and investigate a transformer-based strategy to combine different modalities for survival prediction. Moreover, clinical data was also adopted and different encoding manners of clinical information were explored. Experiments on two public datasets from The Cancer Genome Atlas (TCGA) demonstrate the effectiveness of the proposed graph-transformer framework for survival prediction.
MoreTranslated text
Key words
Survival Prediction,Whole Slide Image,Multi-modality,Transformer,Graph Neural Network
求助PDF
上传PDF
View via Publisher
AI Read Science
AI Summary
AI Summary is the key point extracted automatically understanding the full text of the paper, including the background, methods, results, conclusions, icons and other key content, so that you can get the outline of the paper at a glance.
Example
Background
Key content
Introduction
Methods
Results
Related work
Fund
Key content
- Pretraining has recently greatly promoted the development of natural language processing (NLP)
- We show that M6 outperforms the baselines in multimodal downstream tasks, and the large M6 with 10 parameters can reach a better performance
- We propose a method called M6 that is able to process information of multiple modalities and perform both single-modal and cross-modal understanding and generation
- The model is scaled to large model with 10 billion parameters with sophisticated deployment, and the 10 -parameter M6-large is the largest pretrained model in Chinese
- Experimental results show that our proposed M6 outperforms the baseline in a number of downstream tasks concerning both single modality and multiple modalities We will continue the pretraining of extremely large models by increasing data to explore the limit of its performance
Upload PDF to Generate Summary
Must-Reading Tree
Example
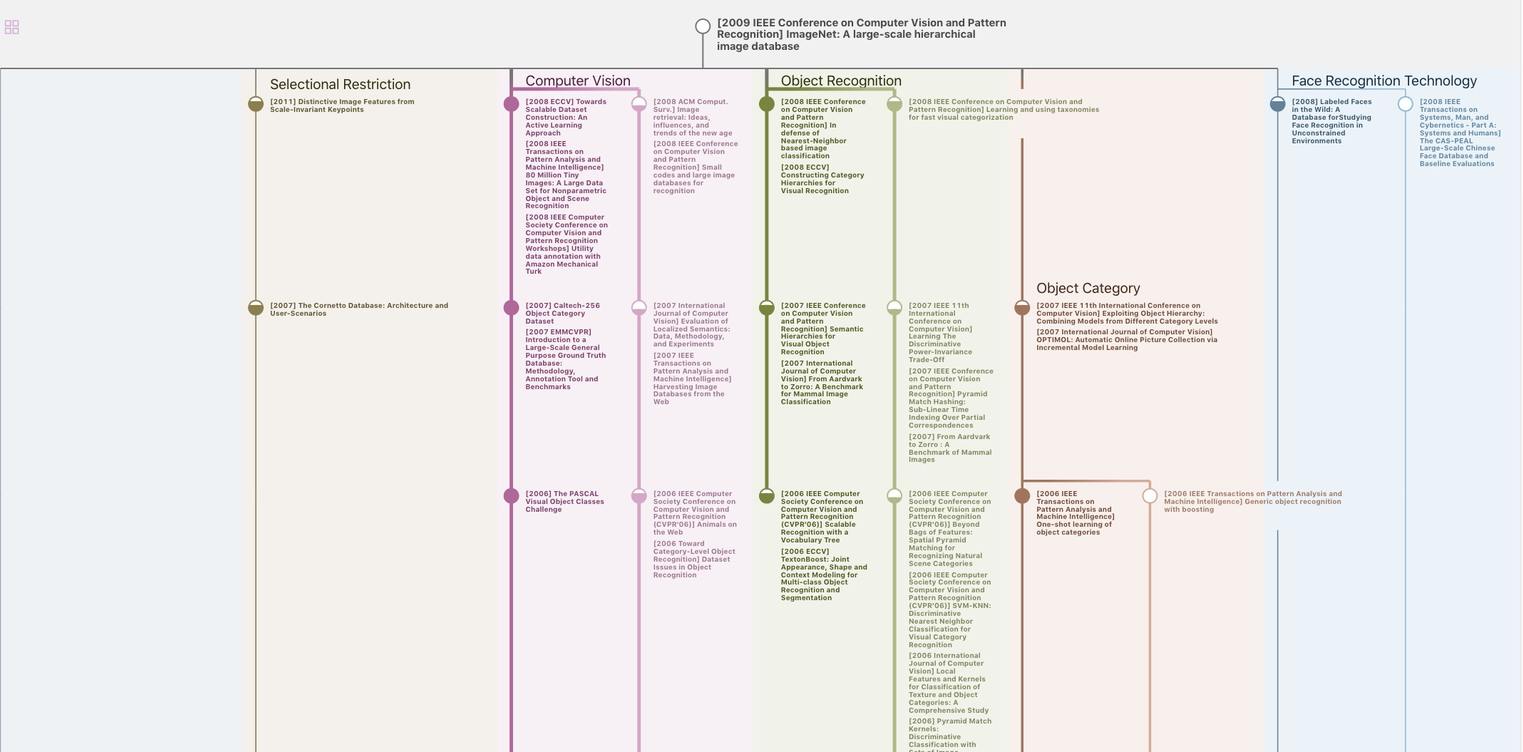
Generate MRT to find the research sequence of this paper
Related Papers
2019
被引用215 | 浏览
2020
被引用145 | 浏览
2020
被引用219 | 浏览
2021
被引用82 | 浏览
2021
被引用27 | 浏览
2021
被引用140 | 浏览
2022
被引用23 | 浏览
Data Disclaimer
The page data are from open Internet sources, cooperative publishers and automatic analysis results through AI technology. We do not make any commitments and guarantees for the validity, accuracy, correctness, reliability, completeness and timeliness of the page data. If you have any questions, please contact us by email: report@aminer.cn
Chat Paper