Multi-View Variational Autoencoders Allow for Interpretability Leveraging Digital Avatars: Application to the HBN Cohort.
ISBI(2023)
摘要
If neural network-based methods are praised for their prediction performance, they are often criticized for their lack of interpretability. When dealing with multi-omics or multi-modal data, neural network methods must be able learn the independent and joint effect of heterogeneous views while yielding interpretable results intra- and inter-views. In the literature, multi-view generative models exist to learn joint information in a reduced-size latent space. Among these models, multi-view variational autoencoders are very promising. In this work, we demonstrate how they provide a convenient statistical framework to learn the input data joint distribution and offer opportunities for the results interpretation. We design a method that discovers the relationships between one view and others. The generative capabilities of the model enable the exploration of a whole disorder spectrum through the generation of realistic values. While modifying a subject's clinical score, the model retrieves a representation of the subject's brain at this clinical status, so-called digital avatar. By computing associations between cortical regions measures and behavioral scores, we showcase that such digital avatars convey interpretable information in a multi-modal cohort with children experiencing mental health issues.
更多查看译文
关键词
behavioral scores,brain,clinical score,clinical status,cortical regions measures,digital avatar,disorder spectrum,generative capabilities,HBN cohort,heterogeneous views,independent effect,input data joint distribution,interpretability,interpretable information,joint effect,joint information,mental health issues,multimodal cohort,multimodal data,multiomics data,multiview generative models,multiview variational autoencoders,neural network methods,prediction performance,reduced-size latent space,statistical framework
AI 理解论文
溯源树
样例
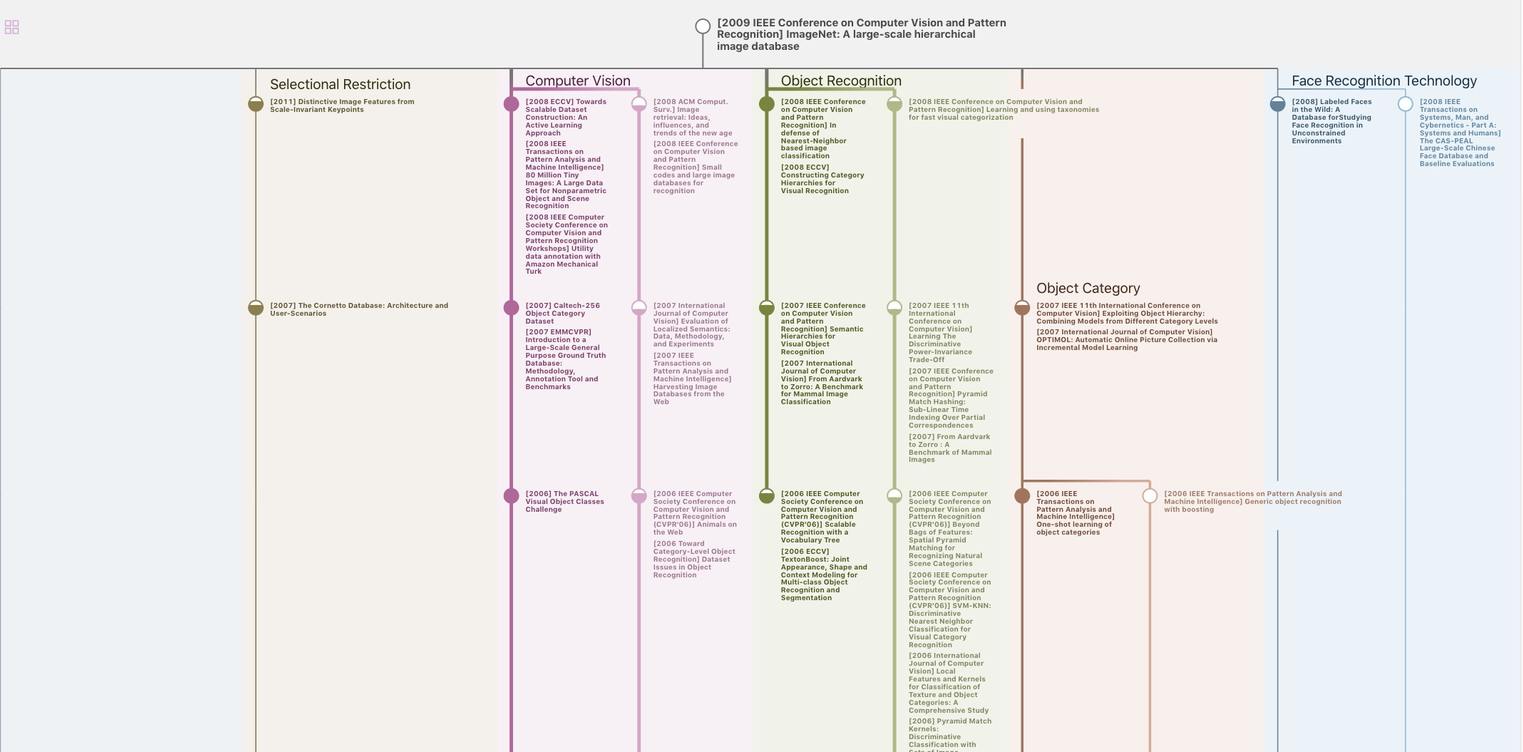
生成溯源树,研究论文发展脉络
Chat Paper
正在生成论文摘要