Effective Training Strategy for NN Models of Working Memory Classification with Limited Samples.
ISBI(2023)
摘要
In biomedical imaging, we often encounter tasks requiring machine learning models to analyze large amounts of variables. However, in practice, limited and imbalanced samples hinder the prediction power of the machine learning methods, particularly for data-hungry techniques like Neural Networks (NN). This study proposes a strategy for training NN models with limited samples. When the data condition result in a model with suboptimal solutions even under a shallow network configuration and best learning practices, we propose a simple yet efficient reusing and refining training strategy, termed self-transfer training. We demonstrated this strategy would improve the model performance. We modified the SFCN (Simple Fully Convolutional Network) model to classify the working memory capacity using structural MRI data. In this study, we analyzed 5469 subjects, of which 3501 have low working memory capacity, and 1968 have high-memory capacity. First, we fully trained the model using distinct sets of hyperparameters and obtained a mean balanced accuracy of 67.80%. Further training the model using the Self-Transfer Training strategy improved the model performance to 72.53%. We conclude that i) the brain imaging features presented in the shallow layers of the models are more generic and can be trained initially and reused, while brain imaging features in later layers are more task-specific and can be refined via further training, and ii) the self-transfer training will aid in improving the model's performance with limited samples to learn.
更多查看译文
关键词
CNNs,Transfer Learning,Structural MRI,Working Memory
AI 理解论文
溯源树
样例
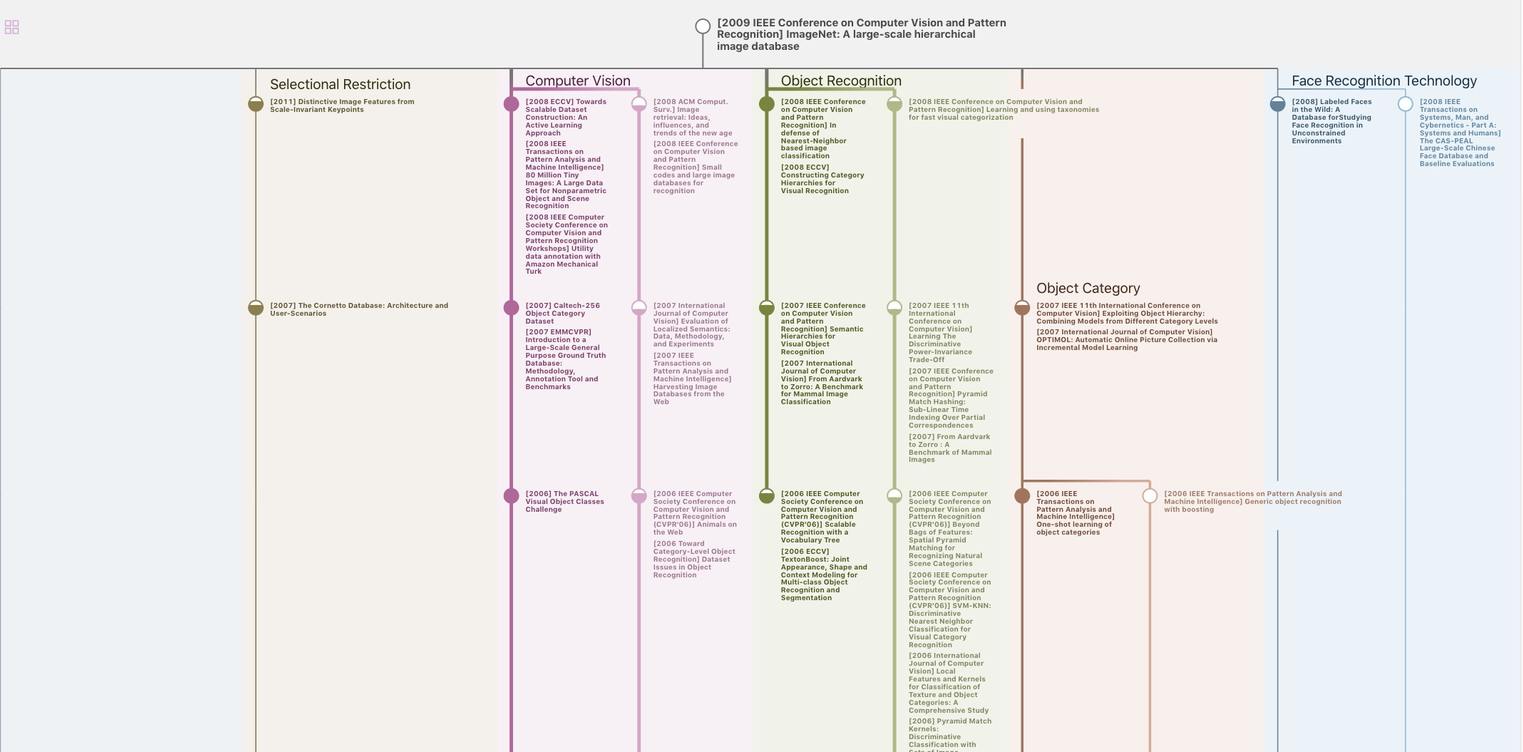
生成溯源树,研究论文发展脉络
Chat Paper
正在生成论文摘要