Joint Representation Learning from French Radiological Reports and Ultrasound Images
ISBI(2023)
摘要
In this study, we explore the value of using a recently proposed multimodal learning method as an initialization for anomaly detection in abdominal ultrasound images. The method efficiently learns visual concepts from radiological reports using natural language supervision and constrastive learning. The underlying requirement of the method is simply the availability of image and textual descriptions pairs. However, in abdominal ultrasound examinations, radiological reports are associated with several images and describe all organs observed during the examination. To address this shortcoming, we automatically construct image and text pairs using 1) deep clustering for abdominal organ classification on ultrasound images and 2) natural language processing tools to extract the corresponding description on the report. We show that pre-training the model with these constructed pairs yields representations that better separate normal classes from abnormal ones on ultrasound images for the kidneys, compared to ImageNet-based representations, with a 10% improvement in macro-average accuracy.
更多查看译文
关键词
multimodal learning, deep clustering, natural language processing, ultrasound examinations, kidneys
AI 理解论文
溯源树
样例
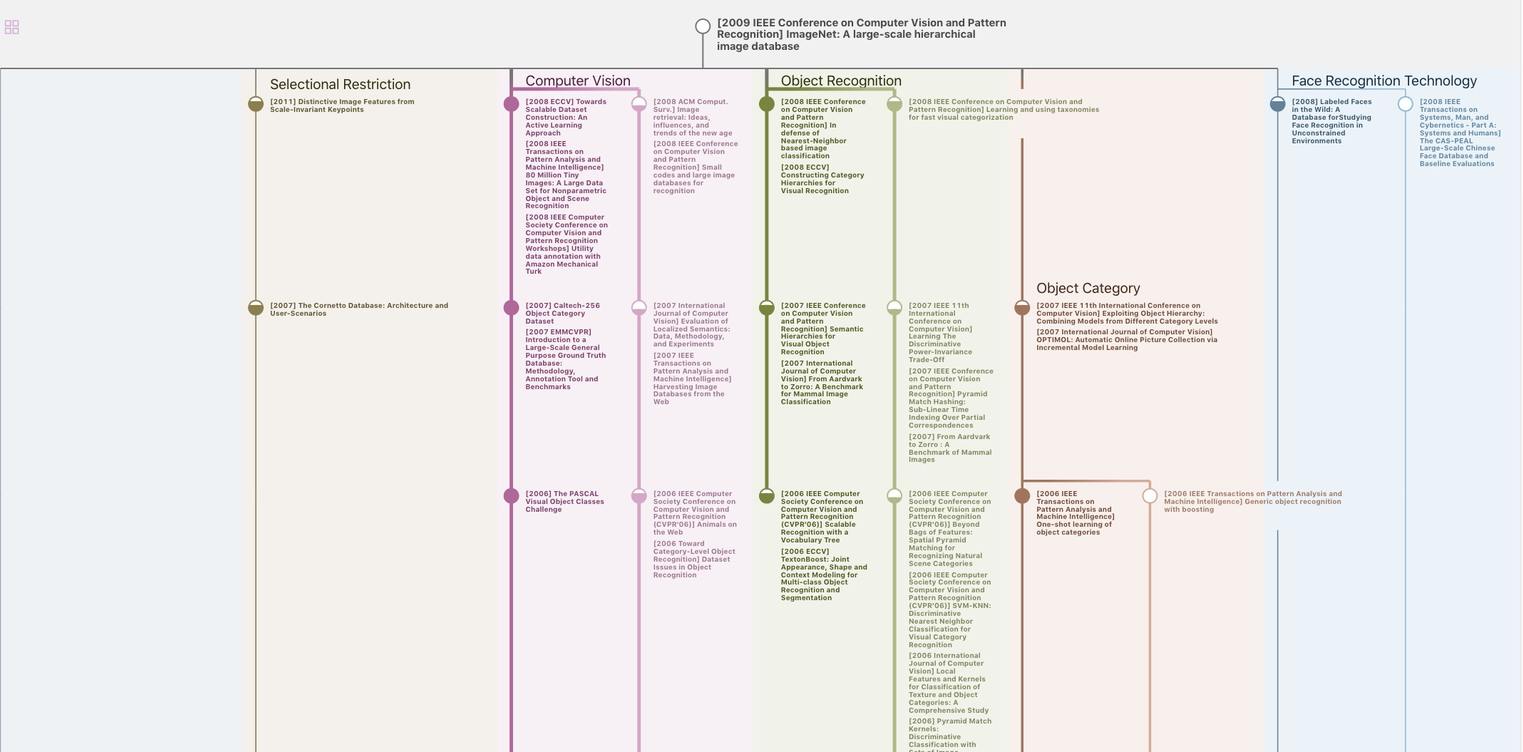
生成溯源树,研究论文发展脉络
Chat Paper
正在生成论文摘要